S. Piccirella1, L. Van Neste2, C. Fowler3, C.L. Masters3, J. Fripp4, J.D. Doecke4, C. Xiong5, D. Uberti6,7, P. Kinnon1
1. Diadem SpA, Brescia, Italy; 2. Halixo BV, Hoegaarden, Belgium; 3. The Florey Institute of Neuroscience and Mental Health, Parkville, Australia; 4. The Australian e-Health Research Centre, CSIRO, Herston, Australia; 5. Washington University School of Medicine, Division of Biostatistics, Saint Louis, MO, USA; 6. Department of Molecular and Translational Medicine, University of Brescia, Brescia, Italy; 7. Molecular Markers Laboratory, IRCCS Istituto Centro San Giovanni di Dio Fatebenefratelli, Brescia, Italy
Corresponding Author: Simona Piccirella, Diadem SpA, Brescia, Italy, s.piccirella@diademdx.com
J Prev Alz Dis 2022;3(9):469-479
Published online May 17, 2022, http://dx.doi.org/10.14283/jpad.2022.52
Abstract
Background: Ongoing research seeks to identify blood-based biomarkers able to predict onset and progression of Alzheimer’s disease (AD).
Objective: The unfolded conformational variant of p53 (U-p53AZ), previously observed in AD individuals, was evaluated in plasma samples from individuals participating in the Australian Imaging, Biomarkers and Lifestyle (AIBL) cohort for diagnostic and prognostic assessment, validated on a neuropsychological-based diagnosis, over the course of six years.
Design: Retrospective Longitudinal Prognostic biomarker study.
Setting: Single-center study based on the AIBL cohort.
Participants: 482 participants of the AIBL cohort, aged 60-85 years, without uncontrolled diabetes, vascular disease, severe depression or psychiatric illnesses.
Measurements: The AlzoSure® Predict test, consisting of immunoprecipitation (IP) followed by liquid chromatography (LC) tandem mass spectrometry (MS/MS), was performed to quantify the AZ 284® peptide as readout of U-p53AZ and compared with an independent neuropsychological diagnosis. The amyloid load via amyloid β-positron emission tomography (Aβ-PET) and supporting clinical information were included where possible.
Results: U-p53AZ diagnostic and prognostic performance was assessed in both time-independent and time-dependent (36, 72 and 90 months following initial sampling) analyses. Prognostic performance of Aβ-PET and survival analyses with different risk factors (gender, Aβ-PET and APOE ε4 allele status) were also performed. U-p53AZ differentiated neuropsychologically graded AD from non-AD samples, and its detection at intermediate/high levels precisely identified present and future symptomatic AD. In both time-independent and time-dependent prognostic analyses U-p53AZ achieved area under the curve (AUC) >98%, significantly higher than Aβ-PET AUCs (between 84% and 93%, P respectively <0.0001 and <0.001). As single factor, U-p53AZ could clearly determine the risk of AD neuropsychological diagnosis over time (low versus intermediate/high U-p53AZ hazard ratio=2.99). Proportional hazards regression analysis identified U-p53AZ levels as a major independent predictor of AD onset.
Conclusions: These findings support use of U-p53AZ as blood-based biomarker predicting whether individuals would reach neuropsychologically-defined AD within six years prior to AD diagnosis. Integration of U-p53AZ in screening processes could support refined participant stratification for interventional studies.
Key words: Alzheimer’s disease, blood-based biomarker, AD, p53, prognosis, U-p53.
Abbreviations: Aβ: Amyloid beta; AIBL: Australian Imaging, Biomarkers and Lifestyle; AUC: Area under the receiver operating characteristic curve; AD: Alzheimer’s disease; APOE ε4: Apolipoprotein E ε4 allele; BACE1: β-site amyloid precursor protein-cleaving enzyme 1; CI: Confidence interval; CN: Cognitively normal; CSF: Cerebrospinal fluid; ELISA: Enzyme linked immunosorbent assay; ESI: Electrospray ionization; HCD: Higher energy collision-induced dissociation; HPLC: High performance liquid chromatography; IP: Immunoprecipitation; MCI: Mild cognitive impairment; MRI: Magnetic resonance imaging; MS/MS: Tandem mass spectrometry; OD: Other types of dementias; PiB-PET: Positron emission tomography utilizing Pittsburgh compound B; ROC: Receiver operating characteristic; PTMs: Post-translational modifications; ROS/RNS: Reactive oxygen/reactive nitrogen species; U-p53: Unfolded p53.
Introduction
Alzheimer’s disease (AD) is a progressive neurodegenerative disorder, starting with an asymptomatic phase of normal cognition lasting approximately two decades. While some individuals experience subjective memory complaints (SMC), all eventually progress to mild cognitive impairment (MCI) before finally reaching the AD stage (1, 2). To date, the formal diagnosis of AD dementia, as stated by the National Institute on Aging and Alzheimer’s Association (NIA-AA), relies on neuropsychological tests further confirmed by brain imaging and cerebrospinal fluid (CSF) sampling (3).
For more than 15 years, different disease-modifying treatments (DMTs) have been investigated, yet none of them were found to be clinically effective, (4-6) except for aducanumab, which was recently approved by the Food and Drug Administration (FDA) for treatment of AD dementia at the MCI stage (7, 8). However, earlier interventions remain unavailable, increasing the need for cost-effective screening biomarkers for early stage prevention clinical trials. The search for effective DMTs would largely benefit from stratification of high-risk participants in clinical trials by the implementation of minimally-invasive blood-based biomarkers that can reliably predict AD in cognitively normal (CN) individuals (9, 10). The pathogenic features of AD that have an onset in the asymptomatic phase may allow identification of early blood-based biomarkers (11, 12). By eliciting compensatory responses, these early pathogenic changes were found to initially prevent the increase in reactive oxygen and nitrogen species (ROS/RNS) through activation of antioxidant mechanisms. However, these compensatory antioxidant responses become inefficient throughout the AD continuum, resulting in progressive oxidative stress exacerbation (13-16). This increase in oxidative stress has been described to induce redox post-translational modifications (PTMs) of the p53 protein, altering its native structure and disrupting its physiological functions (17). Besides redox PTMs, in vitro experiments have shown that nanomolar concentrations of Aβ1-40 and Aβ1-42 can induce similar alterations in the tertiary structure of p53, previously described as the unfolded p53 conformational variant (U-p53) (18-20). Accordingly, higher levels of U-p53 have been consistently detected in peripheral cells derived from patients with AD as compared with controls (21-23). Recently, a novel antibody (2D3A8) was developed to detect this AD-specific U-p53 variant (herein described as “U-p53AZ”) (24), demonstrating its promising performance in predicting the AD risk at the asymptomatic and symptomatic stages (24, 25).
In the present study, we 1) investigated U-p53AZ abundance across neuropsychologically defined diagnostic groups (no memory complaints [NMC], SMC, MCI and AD), 2) tested the diagnostic performance of both U-p53AZ and the amyloid burden (Aβ) to detect AD neuropsychological status, and 3) assessed the prognostic performance of U-p53AZ in differentiating individuals at risk of developing AD from individuals with stable diagnoses. With these analyses we thus further confirm the potential of U-p53AAZ as a reliable and minimally-invasive blood-based biomarker to predict the neuropsychological onset of AD in cognitively normal (CN, asymptomatic) or MCI (early symptomatic) individuals.
Materials and methods
Subjects
The plasma samples and supporting clinical information were provided by the Australian Imaging, Biomarkers and Lifestyle (AIBL) longitudinal cohort study, active since 2006 (26, 27). In total, 482 subjects aged between 60 and 85 years who did not present specific comorbidities (uncontrolled diabetes, vascular disease, severe depression, or psychiatric illnesses) were included in this retrospective study, collecting a blood sample for each included subject from the AIBL study by applying a consecutive sampling approach. Subjects were followed-up every 18 months. At each visit, the neuropsychological status and medical history were reviewed by a neuropsychologist and clinician. A clinical review panel consisting of a geriatrician, a neurologist and a neuropsychologist, blinded to amyloid β-positron emission tomography (Aβ-PET) and biomarker status, determined the diagnosis of CN, MCI and AD subjects. Additionally, three subjects were defined to be affected by other forms of dementia (OD), while some were classified as OD by their last diagnosis. Data on the mini-mental state examination (MMSE), clinical dementia rating Scale (CDR) and amyloid brain burden (determined by PET with the labelled Pittsburgh compound B [PiB-PET], flutemetamol, florbetapir, or NAV4694) were collected. Data on Aβ-amyloid burden was converted to the 100-points-based centiloid calibration scale (28) and subjects were assigned to different amyloid categories according to the following scoring in centiloid units (CL): “Negative” <15 CL, “Uncertain” ≥15 CL and <25 CL, “Moderate” ≥25 CL and <50 CL, “High” ≥50 CL and <100 CL, “Very high” ≥100 CL. Individuals with missing imaging values were not included in some analyses of this study. Based on the NINCDS-ADRDA criteria, participants were classified as cognitive normal (CN), MCI and symptomatic AD solely according to their neuropsychological status and irrespective of traditional markers as Aβ-PET status and tau. The CN diagnostic group consisted of two subgroups: no memory complaints (NMC) and subjective memory complaints (SMC). The term “AD” herein used refers to AD as defined on the neuropsychological status found in the individuals and does not represent clinical AD based on Aβ status. The appropriate institutional ethics committee approved this study, which was carried out following all relevant ethical regulations (Human Research Ethics Committee, Research Governance Unit, St Vincent’s Healthcare, Australia (no. 028/06)).
Blood processing and APOE alleles genotyping
According to the AIBL standard operating procedures, blood samples were handled at room temperature in EDTA collection tubes (S-Monovette®, Sarstedt, Germany) preventively supplemented with prostaglandin-E (final concentration: 33 ng/mL, Sapphire Biosciences, Australia). Processing took place within three hours from blood withdrawal, followed by aliquoted sample storage in liquid nitrogen. Genotyping was carried out as has been previously described (29).
Immunoprecipitation (IP) and nanoflow electrospray ionization tandem mass spectrometry (ESI-MS/MS)
The 2D3A8 antibody selectively binds the AD-clinically relevant U-p53AZ (24, 30) and was here deployed to identify peptides through protein sequencing via MS/MS. Protein sequencing was performed at MyomicsDX Inc. (MD, USA). IP took place on high abundance protein-depleted plasma samples with different antibodies. One reaction was performed with 2D3A8 (10 μg/sample, Diadem SpA, Italy), while the other was based on a mixture of p53-specific antibodies at 10 μg/sample (DO11:DO12:SAPU:KJC12, respective volume ratios 1:1:2:2 and final concentration of 1 μg/μl) (31, 32). Upon enrichment, the peptides were eluted, fractionated through an Agilent 1290 Infinity II liquid chromatography (LC) system (Agilent, CA, USA) and analyzed in MS by a Thermo Scientific™ Q Exactive Mass Spectrometer (Thermo Fisher Scientific, MA, USA). Tandem mass tag labelled peptides were analyzed by MS/MS in a data-dependent approach on a Thermo Scientific™ EASY-nLC 1000™ HPLC system, coupled to a Thermo Scientific EASYSpray™ source supported by an analytical nanoflow column system (Thermo Fisher Scientific, MA, USA). Acquisition of the survey full scan MS spectra (m/z 350−1800) was achieved with the Orbitrap with 35,000 resolution, following ion accumulation to a 3 × 106 target value, selected on predictive automated gain control based on the previous full scan. Sequential isolation of the 10 most intense multiply charged ions (z ≥ 2) followed and these were then fragmented in the Axial Higher energy Collision-induced Dissociation (HCD) cell through normalized HCD collision energy at 30% (automatic gain control target: 1e5, maximal injection time: 400 ms, resolution: 35,000). The Proteome Discoverer 2.2 software (Thermo Fisher Scientific, MA, USA) processed automatically the MS raw files, and Xtract was deployed in addition to default spectrum selector node. The Mascot search engine combined with Sequest HT (interfaced with different processing nodes of Proteome Discoverer 2.2) was used to address the searches. The final dataset was reprocessed through the MyProt-QuantiR (MyOmicsDx Inc., MD, USA) software package, allowing the identification of the AZ 284® peptide sequence as the most clinically relevant peptide in samples from individuals affected by AD.
Sample preparation
Fractions of samples provided by the AIBL cohort (25 μL) underwent IP using the patented 2D3A8 monoclonal antibody (30) (Diadem SpA, Italy) coupled to Protein L magnetic beads (Thermo Fisher Scientific, MA, USA). Upon protein enrichment, the samples were treated with trypsin (3.5 hours at 37°C followed by 0.5 hours at 57°C).
AlzoSure® Predict test: measurement of U-p53AZ in patient plasma samples
U-p53AZ plasma levels were assessed through quantization of the peptide AZ 284® by LC coupled to electrospray ionization (ESI)-MS/MS at ISB srl (Italy). A surveyor HPLC Thermofisher Quaternary Pump was paired to a ThermoFisher mass spectrometer. For analyte separation, a Phenomenex Kinetex PFP column (50×4.1 mm, 2.6 m, Phenomenex, CA, USA) was used and the mobile phases were A (H2O-0.2 % HCOOH, Sigma-Aldrich, MO, USA) and C (CH3OH, Sigma-Aldrich, MO, USA). LC was performed in a binary gradient at a chromatographic flow of 0.2 mL/min: 2% of phase C was maintained for 2 minutes and raised to 40% in 3 minutes and maintained as such for 7 additional minutes. Phase C was further raised to 70% 2 minutes later and kept at 70% for 4 minutes before resetting to the starting conditions for 5 minutes. A calibration curve was developed prior to analysis of the clinical samples, spiking a negative control of plasma with AZ 284® labelled peptide covering the range between 0.02 fmol/10 μl and 4 fmol/10μl by at least 4 different points. Peptide sequence was then analyzed with a ThermoFisher Mass Spectrometer (TSQ Vantage Thermo Fisher Scientific heated-ESI ion source, MA, USA). The ion source parameters were: multiple reaction monitoring scan; capillary temperature: 320°C; collision energy: 5eV; sheath gas flow: 30 L/min; auxiliary gas flow: 2 L/min; and sweep gas flow: 15 L/min. All tests were performed while being blinded from the clinical and cognitive data. In the present study, arbitrary thresholds were temporarily defined to assign individuals to pre-established categories, namely “low U-p53AZ” for AZ 284® <0.76 fmol/10μl, “intermediate U-p53AZ” for 0.76 fmol/10μl ≤ AZ 284® <1.28 fmol/10μl and “high U-p53AZ” for AZ 284® >1.28 fmol/10μl. The high level threshold was set at the maximal Youden index that separates AD from non-AD diagnoses at baseline, while the low level threshold was determined similarly, but using the final diagnosis instead.
Statistical analyses
The diagnostic and prognostic performance of U-p53AZ to predict AD were assessed. The performance of amyloid status (as measured by Aβ imaging, calibrated CL, or inferred Aβ categories as reported above) was included as reference at the diagnostic level and compared with U-p53AZ at the prognostic level. Survival curves were plotted on risk models based on U-p53AZ alone or a model encompassing all the risk factors.
Diagnostic analyses were performed on the biomarker data and neuropsychological assessments and the analyses used measurements defined at the baseline assessment (i.e., the sample corresponding to the visit at which U-p53AZ was first measured). The diagnostic performance was evaluated through two specific models based on the neuropsychological assessment: 1) comparing AD individuals with non-AD individuals (including subjects in the study pooled from any other diagnosis than AD) and 2) specifically comparing CN and MCI subjects with AD subjects.
For prognostic evaluation, both time-independent and time-dependent analyses were performed to verify the potential of U-p53AZ in predicting the diagnosis of AD. Time-independent analyses were structured using the baseline measure of U-p53AZ and Aβ-PET to discern AD-progressing individuals from stable non-AD subjects based on their final neuropsychological diagnosis. Time-dependent analyses of individuals regardless of the neuropsychologically assigned status (AD versus non-AD) were included and compared when available with information on amyloid status (considered positive for a CL score ≥15). In a sub-cohort of samples with available amyloid status data, the prognostic performance of U-p53AZ was compared to the performance of the Aβ-PET biomarker. In this comparison, non-AD individuals for which their true disease status was unknown or ambiguous due to lack of sufficient follow-up were right-censored in these specific analyses.
A survival analysis with respect of AD-free survival was performed excluding subjects with a baseline neuropsychological AD diagnosis to assess the prognostic value of U-p53AZ from the baseline measurement. A right-censored model was considered to be sufficiently accurate and allowed the use of a semi-parametric Cox proportional hazards model, based on 1) the considerably larger median follow-up times for CN (67 months), MCI (27 months) and OD (37 months) compared to the interval period between patient visits (approximately 18 months) during which AD onset could occur, and 2) the 20-month median time to progression observed for those patients whose neuropsychological status changed to AD. Cox models were evaluated for 1) U-p53AZ alone (N=338), or 2) along with other covariates (U-p53AZ, CL, baseline age, gender and dichotomous APOE ε4 status; N=294). Hazard ratios (HR) were then evaluated for all risk factors in models adjusted or unadjusted to the factors and two models (U-p53AZ and a complete model including all factors) were then plotted as Kaplan-Meier curves to visualize the probability of AD onset according to the risk strata. The analyses were obtained using survival, survminer, and survcomp R packages. For direct HR comparison, the continuous variables of the reference model (U-p53AZ, Aβ-PET [CL] and age) were normalized into standard scores by subtracting the mean from each value and dividing by the standard deviation.
To evaluate diagnostic and prognostic performances, receiver operating characteristic (ROC) curves were generated and their corresponding area under the curve (AUC) were calculated to determine the accuracy, sensitivity, specificity, positive (PPV) and negative predictive values (NPV), using the R-package bdpv. Unless otherwise specified, cut-offs for sensitivity and specificity were determined using the maximal Youden J statistic, i.e. the sum of sensitivity and specificity. Prognostic analyses were performed in R, using the pROC and timeROC packages for time-independent and time-dependent analyses respectively (33-35). ROC curve analyses to determine the optimal cut-off were performed using the cutpointr package (36).
Where applicable, numerical values were compared using Student’s T test or ANOVA followed by Tukey’s test for evaluation of pairwise differences. Differences in follow-up time were evaluated using a non-parametric Wilcoxon rank sum test with continuity correction. Fisher’s exact test was used to evaluate categorical count data. Comparison of ROC curves was performed through the DeLong test, while NPV and PPV were defined at a prevalence rate of AD of 30% at baseline and 45% at the final diagnosis. All the mentioned analyses were carried out in R.
Results
Demographics and characteristics of the study cohort
In total, samples were included from 482 individuals spanning the AD continuum. Three of these individuals who were initially diagnosed with neuropsychological AD, but afterwards with OD, were excluded from the dataset due to lacking or persistently high amyloid levels resulting in a non-congruent pathology. Out of the 479 included individuals, 422 (88%) and 472 (99%) had available information on Aβ load (quantified by CL) and APOE ε4 allele status (through genotyping), respectively. The demographics and neuropsychological classification at baseline, as well as the neuropsychological classification at last follow-up, are shown in Table 1.
During the follow-up visits, a subset of participants changed their neuropsychological classification towards MCI, AD, or OD. Additional insight into the total number of subjects changing neuropsychological classification status can be found in Supplementary Material (Table 1). A flow of subjects across diagnostic and prognostic analyses is also reported, in Supplementary Material (Figure 1).
AD: Alzheimer’s disease; CN: cognitively normal; IQR: interquartile; MCI: mild cognitive impairment; NMC: no memory complaints; non-AD: individuals classified other than AD (as CN, MCI, or OD); OD: other dementia; SMC: subjective memory complaints; The total number of subjects involved, regardless of the groups or subgroup, refers to the neuropsychological classification at the last follow-up. The distribution in subgroups (non-AD or AD) at baseline is organized according to the final classification; a Percentages were calculated on the individuals included in the analyses (i.e., missing subjects [Not available] were not considered in this analysis); P-values in the parameters column resulted from the comparison of non-AD and AD subjects for the specific entry. Numerical values were compared using a standard T-test, categorical values by Fisher’s Exact test; ** P≤0.01; *** P≤0.001.
Levels of U-p53AZ in different diagnostic groups
The levels of U-p53AZ were assessed by AZ 284® quantization and visualized according to the baseline neuropsychological classification, defined as NMC, SMC, MCI and AD (Figure 1). Although U-p53AZ was similarly distributed among individuals neuropsychologically diagnosed as NMC and SMC, it was markedly increased in individuals with MCI and AD. For statistical testing, NMC and SMC were combined as a single group due to the subjective nature of this grouping and the overall similar behavior of U-p53AZ. Subsequent ANOVA testing was performed to compare mean levels of U-p53AZ, underscoring a significant difference across neuropsychological groups (P<0.001) (Figure 1A), which was further substantiated by Tukey test for difference across different groups (P<0.001). Accordingly, plasma U-p53AZ could accurately and reliably discriminate subjects at the asymptomatic stage (NMC and SMC) from those with AD (AUC=99.8%; 95% CI: 99.6-100.0%) with a sensitivity of 95.0% and specificity of 99.6%. Similarly, the sensitivity and specificity of U-p53AZ to differentiate subjects at the early symptomatic stage (MCI) from those with neuropsychological AD status (AUC=95.7%; 95% CI: 92.8-98.5%) were 95.0% and 91.8%, respectively. This was confirmed categorizing individuals at final neuropsychological diagnosis (i.e., last visit) according to their baseline U-p53AZ levels, which were significantly different across groups (ANOVA; P<0.001) and even among individuals with MCI and AD (Tukey test; P<0.001) (Figure 1B). Interestingly, baseline levels of U-p53AZ in individuals with stable neuropsychological MCI through the study (i.e. baseline and final diagnosis; N=38) were significantly lower (Tukey test; P<0.001) compared to those MCI patients that developed AD (N=60), suggesting that individuals with MCI who had high U-p53AZ levels at baseline are more likely to convert to neuropsychological AD status over time. Similarly, individuals who changed their neuropsychological classification from CN to AD (N=8) presented significantly higher baseline levels of U-p53AZ compared with those (N=207) who had a stable CN profile (Tukey Test; P=0.003). A similar trend of higher U-p53AZ was noted for CN individuals (N=17) whose neuropsychological classification changed to MCI over the course of the study (Tukey Test; P<0.001).
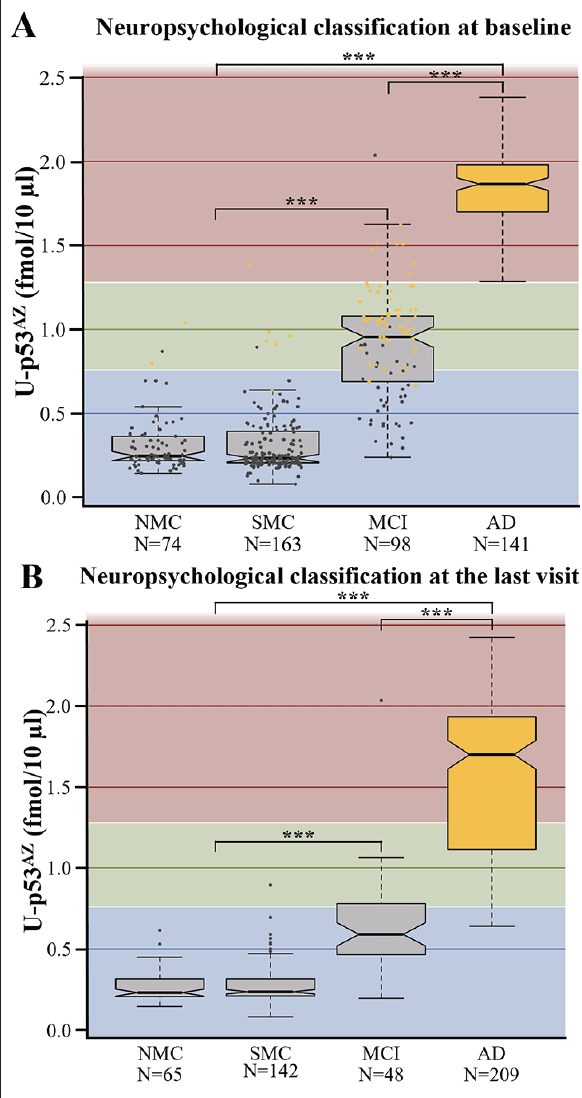
Figure 1. Boxplots showing the average levels of U-p53AZ in different neuropsychological classifications. A) U-p53AZ levels across diagnostic groups as defined at baseline. B) U-p53AZ levels across diagnostic groups as defined at the final follow-up (i.e., the last visit) used in this study
AD: Alzheimer’s disease; MCI: mild cognitive impairment; NMC: no memory complaints; SMC: subjective memory complaints. Color-coding in Figure 1A: blue background: low U-p53AZ; green background: intermediate background U-p53AZ; red: high U-p53AZ; grey dots: individuals whose status was non-AD at the final diagnosis; yellow dots: individuals whose status was AD at the final diagnosis. The Y axis reports AZ 284® concentration (fmol/10 μL) and is used as a readout of U-p53AZ, after which is labelled. *** P<0.001
Diagnostic performance of U-p53AZ and Aβ-PET to detect AD neuropsychological status
The performance of U-p53AZ and Aβ-PET for AD diagnosis was evaluated based only on the neuropsychological classification (Figure 2, Supplementary Material Table 2).
Given the promising trend observed for U-p53AZ, with the available dataset its association to the AD neuropsychological status was further investigated in subsets comparing CN with AD and MCI with AD (Supplementary Material Table 3). In both subgroups, U-p53AZ consistently scored a high performance (AUC>95%) in differentiating respectively CN and MCI individuals from AD ones, indicating its association with AD defined on the basis of neuropsychological assessment.
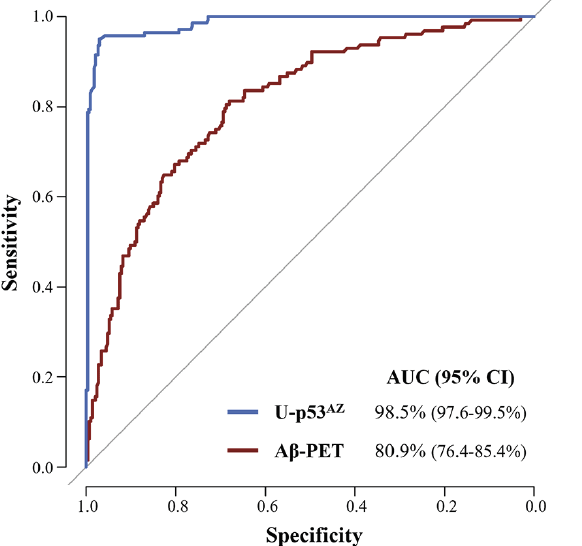
Figure 2. ROC curves comparing the performance of U-p53AZ and Aβ-PET (CL numerical values) to differentiate AD from non-AD individuals at baseline neuropsychological diagnosis
AUC: area under the curve; CI: confidence interval.
The potential of U-p53AZ to identify individuals at risk of AD neuropsychological diagnosis
Both U-p53AZ levels and amyloid categories (as determined by Aβ-PET) were evaluated, based on availability, in a subset of respectively 476 and 419 subjects to detect individuals at high risk of AD progression based on assessment of the neuropsychological classification. Individuals were classified in five diagnostic groups: 1) “CN”, 2) “MCI”, 3) “CN individuals whose neuropsychological classification changed to MCI by the end of the follow-up” (cognitive decline [CD] progressors), 4) “CN or MCI individuals neuropsychological classification changed to AD by the end of the follow-up” (AD progressors), and 5) “individuals with neuropsychological AD” (AD). Classification of the different diagnostic groups by U-p53AZ categories (“low U-p53AZ”, “intermediate U-p53AZ” and “high U-p53AZ”) indicated that the majority of subjects belonging to the AD progressors and AD groups were classified to have intermediate to high U-p53AZ measurements (respectively, 96% and 99%) (Supplementary Material, Figure 2A and Table 4). The same dataset, classified by amyloid categories as estimated within each neuropsychological group, was in line with the U-p53AZ classification, as most individuals who were CN presented low amyloid burden (Supplementary Material, Figure 2B and Table 4). However, individuals with AD and those whose neuropsychological classification changed to AD were less clearly assigned to risk amyloid categories, as high and very high amyloid (namely, 79% for AD subjects and 78% for AD progressors). MCI subjects were similarly identified between risk amyloid categories and U-p53AZ status (47% and 39%). For further clarification over the detection potential of U-p53AZ for at-risk groups, the subsequent analyses focused on assessing its prognostic performance.
Prognostic performance of U-p53AZ to predict the onset of AD as assessed through the neuropsychological status
Due to the promising performance of U-p53AZ in identifying individuals at risk of changing neuropsychological classification to AD, a time-independent evaluation of prognostic performance to differentiate AD from non-AD was performed on the dataset as classified at final neuropsychological diagnosis (Supplementary Material, Table 5). The baseline quantification of U-p53AZ and of Aβ-PET were included and compared in the analysis. Based on subject classification at final neuropsychological diagnosis, U-p53AZ presented a significantly greater performance compared to Aβ-PET, underscoring the potential of U-p53AZ in prognostic settings (U-p53AZ AUC [95% CI]=99.0% [98.2-99.8%], Aβ-PET AUC [95% CI]=83.5% [79.5-87.5%], P<0.0001).
Baseline levels of U-p53AZ were also considered to predict neuropsychologically diagnosed AD at different timepoints following plasma collection, independently of the initial classification. Time-dependent ROC analyses were thus performed to gain insight into the prognostic performance at specific timepoints. Samples from non-AD individuals who changed neuropsychological group to AD were compared with samples from non-AD individuals whose status remained non-AD (either changing to another non-AD group, or remaining in the same diagnostic group). By time-dependent ROC curves, the prognostic performance of U-p53AZ to predict neuropsychological AD was superior to amyloid brain imaging by Aβ-PET 36 and 72 months after baseline assessment (Figure 3). The corresponding AUC values of both markers are displayed in Supplementary Material (Table 6), confirming that the prognostic performance of U-p53AZ was significantly better than that of Aβ-PET for identification of individuals whose neuropsychological status would change in time to AD (P<0.001).
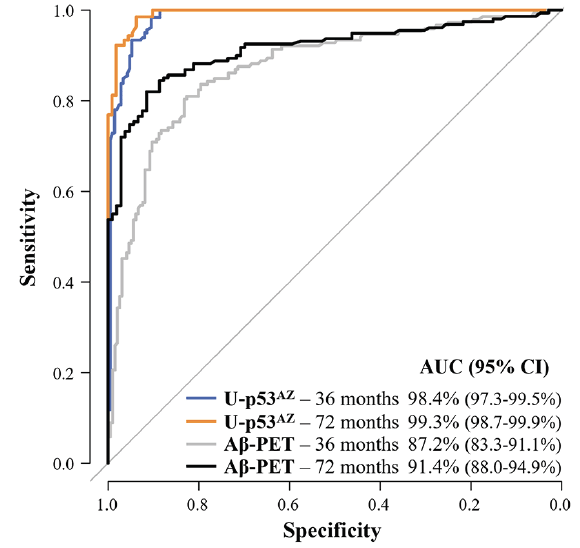
Figure 3. Prognostic performance of U-p53AZ and Aβ-PET (CL-based Aβ categories) in predicting the change of neuropsychological classification to AD
ROC curves show the performance of U-p53AZ and Aβ-PET in predicting the onset of neuropsychological AD in non-AD individuals at 36 and 72 months following baseline assessment compared to individuals whose neuropsychological remained stable or progressed to another non-AD group (values in Supplementary Material, Table 5). AUC: area under the curve; CI: confidence interval.
The probability of progression due to U-p53AZ or due to other risk factors
To assess the relevance of U-p53AZ as prognostic AD biomarker throughout time, Cox proportional hazards models were created based on either a single risk factor (U-p53AZ) or different combinations of multiple risk factors (including U-p53AZ and traditionally accepted factors as Aβ-PET, APOE ε4 and age) (Supplementary Material, Table 7). Kaplan-Meier curves were then plotted for U-p53AZ alone (Figure 4A, dividing the subject population among “low” and “intermediate-high” in reference to the preliminary U-p53AZ threshold adopted in the present study) and for a multifactorial model based on U-p53AZ, Aβ-PET, APOE ε4 and age (Figure 4B).
Both models precisely divided the population in high and low risk of neuropsychological AD onset based on the selected thresholds and strata (P<0.001). Hazards ratios (HRs) were estimated for risk factors included in adjusted and non-adjusted models (Supplementary Material, Table 8). Evaluation of an adjusted model normalized for all the factors further enabled the direct comparison of HRs across risk factors, indicating that U-p53AZ is the major contributor and an independent predictor for cognitive decline to neuropsychological AD (HR=2.92, P<0.0001).
Plots for A) U-p53AZ positivity and B) all the risk factors.
Discussion
In the last years, there has been a growing interest in blood-based biomarkers allowing early identification of individuals at risk for developing AD (10). Despite these efforts, none of the studied blood-based biomarkers have yet been included in the screening protocols for the assessment of AD risk (3), leaving more than half of individuals with AD dementia without a formal diagnosis (10). Additionally, clinical intervention trials suffer from the lack of accessible prognostic biomarkers, leading to inadequate enrolment of individuals at risk of AD. Implementation of minimally-invasive and reliable blood-based biomarkers could respond to these unmet needs increasing the accuracy of AD diagnosis while improving clinical trial enrolment (9, 10). This is particularly relevant in the coming years due to aging of the population and the subsequent increase in the numbers of patients receiving the clinical diagnosis of AD (37).
One of the recently discovered blood-based biomarker candidates is a conformational variant of p53. Although p53 is mostly known for its role as tumor suppressor, it is now clear that p53 is regulating cell fate decisions and preventing neurodegeneration through multiple mechanisms, including DNA damage repair, protection from oxidative stress, support of axonal regeneration, neuronal outgrowth and synaptic function as well as repression of β-site amyloid precursor protein-cleaving enzyme 1 (BACE1) expression (38-43). Nevertheless, its neuroprotective function becomes gradually lost throughout the AD continuum, as exposure to increasing amounts of Aβ and ROS/RNS leads to unfolding of the p53 protein resulting in elevated levels of the p53 conformational variant, identified as U-p53 (17-19, 44). U-p53 has previously been detected in peripheral cells derived from MCI and AD individuals and was shown to be predictive of the onset of AD (21-23). Although promising, these preliminary studies were based on limited patient numbers and conventional techniques, such as flow cytometry and ELISA, using commercially available antibodies (21, 22). Assessment of U-p53 has now been improved by the development of a novel antibody (2D3A8) specifically targeting the AD clinically relevant U-p53 conformational variant (U-p53AZ) (24, 25). To further improve its analytical performance, U-p53AZ was quantified by the AlzoSure® Predict method, a LC-ESI/MS/MS sequential technique using plasma samples from individuals at different stages of the AD continuum, available through the longitudinal AIBL cohort. Due to its high specificity in comparison with conventional techniques and its increasing accessibility at lower costs, MS has been widely used in several blood-based biomarker studies (45-47). By using this technique, the present study aimed to assess whether quantification of U-p53AZ was able to assign individuals to the correct diagnostic group and determine its performance for predicting the onset of AD at the asymptomatic and symptomatic stages.
The present study confirms and further expands former findings over the potential of U-p53AZ implementation in prognostic tests for AD (25). First, analysis of U-p53AZ distribution (through LC-MS/MS AZ 284® detection) across diagnostic groups both at baseline and last available assessment markedly revealed higher levels of the misfolded conformation in plasma samples of MCI and AD individuals, as well as of those whose classification changed (i.e., worsening of neuropsychological status to MCI or AD) over the time of the study. The higher U-p53AZ levels in individuals at risk of progression were also suggestive of a possible role of U-p53AZ as predictor of future AD. Secondly, the levels of U-p53AZ associated with baseline neuropsychological diagnoses of AD, in line with the diagnostic performance of Aβ-PET in discriminating AD from non-AD subjects. Such observation lead also to consistent results in selected subgroups, in which U-p53AZ reliably differentiated both CN and MCI individuals from AD ones (respectively, AUCCN vs AD[95% CI]=99.8% [99.6-100.0%] and AUCMCI vs AD[95% CI]=95.7% [92.8-98.5%]). Third, we noted that the promising association of increased U-p53AZ levels with individuals already AD or at risk of deterioration was further confirmed through U-p53AZ categories (subdivided in “low U-p53AZ”, “intermediate U-p53AZ” and “high U-p53AZ”): individuals progressing towards AD or already diagnosed as AD majorly presented intermediate to high levels of U-p53AZ. Interestingly, the same analysis revealed that progressors to CD (as CN individuals reclassified as MCI by the end of follow-up) were mostly characterized by low U-p53AZ levels, suggesting the prognostic specificity of U-p53AZ categories for AD detection rather than for the onset of cognitive impairment. The prognostic potential was further confirmed by time-independent and -dependent analyses of performance for both U-p53AZ and Aβ-PET, in which the former proved to be a powerful and precise predictor of AD onset, even long time before the formal diagnosis. Notably, while AUC values for Aβ-PET ranged between 87% and 92%, in the same subset of samples and time period (36-90 months) U-p53AZ presented a consistently and significantly higher performance (≥98%, P<0.001). Finally, Cox proportional hazard models weighting the contribution of different factors (dichotomous APOE ε4 allele status, Aβ-PET, age, gender, as well as U-p53AZ) confirmed the important role of U-p53AZ in predicting AD onset for detection in the intermediate to high threshold, as defined here in an arbitrarily assigned threshold. Inclusion of a single factor, as U-p53AZ, was sufficient to correctly predict present or future AD classification.
Generally, the herein presented results confirm and expand the ones from a previously published study using a similar technique. However, by including a larger study cohort, the predictive performance of U-p53AZ was found to be further improved (25). When comparing to a model based on magnetic resonance imaging (MRI), PET, CSF and covariates (48), the predictive potential of U-p53AZ was higher and without the limitations associated with costs and invasiveness.
While the development of blood-based biomarkers was initially intended for diagnostic purposes, their potential in accurate prediction of the onset of AD in non-demented CN adults and MCI patients is gaining more interest (49). As an example, plasma phospho-tau181 levels were recently shown (50) to predict the change of neuropsychological classification of individuals from SMC to AD with AUC values comparable to U-p53AZ (51). This poses the question whether screening based on an unique biomarker instead of a panel could be used for the precise identification of individuals who are prone to develop AD dementia. The data retrieved and used in the present study was largely based on imaging techniques, and the results herein reported majorly addressed the U-p53AZ performance within this framework. The achieved results indicate a promising trend on future applications of U-p53AZ as unique prognostic blood-based biomarker readily available for population screening, although by design this study was not developed to draw a final conclusion regarding the positioning of U-p53AZ in comparison with that of traditionally accepted CSF biomarkers. The ongoing follow-up study, as detailed in a subsequent passage of the discussion, could further confirm the observed trend.
Although our findings are suggestive of the predictive potential of U-p53AZ, the results herein described are based on a single longitudinal study, largely lacking follow-up data on amyloid burden. Hence, certain subjects were assigned to AD without knowing the amyloid status. Moreover, for some individuals the available Aβ status was initially present but negative on PET imaging, technically not classifying as AD dementia, while lacking sufficient follow-up data. Insufficient follow-up can give the false diagnosis of non-AD, while the marker is known for its diagnostic power since presents a later onset through PET (52). This lead to a discrepancy between the assigned AD classification and the performance of amyloid, despite the body of literature supporting amyloid positivity as the gold standard for AD dementia diagnosis.
Further studies will ensure a more complete follow-up, including different biomarkers for clinical AD confirmation as Aβ biomarkers (Aβ42), pathologic Tau biomarkers (total and phopsho-181) and severity staging through neurodegenerative biomarkers (NFL) (3), enabling a more rigorous adherence to the clinical classification. These studies will also include larger sample numbers provided by different centers to validate the performance of U-p53AZ in predicting onset of AD dementia and to determine a final cut-off that can be integrated in clinical practice for stratification of CN individuals who are at high risk to develop AD dementia. The ongoing follow-up study uses additional longitudinal data from different cohorts and centers, including individuals from Europe and USA, to validate the herein presented findings and to compare and correlate the potential of U-p53AZ as blood-based biomarker with traditionally studied markers of AD pathology.
Additionally, assuming that preventing the development of AD dementia could result in the reduced increase of U-p53AZ levels, the use of U-p53AZ could be evaluated to support patient stratification in clinical trials and assessment of DMTs effectiveness.
In conclusion, the present study demonstrated the high performance of U-p53AZ to predict the onset of AD in asymptomatic individuals or individuals early symptomatic stage, as early as six years prior to a neuropsychological AD diagnosis. We believe that quantification of U-p53AZ through AlzoSure® Predict has the potential to be implemented in screening strategies, allowing improved stratification of individuals at high risk to develop AD dementia in clinical intervention trials to subsequently support the development of effective DMTs. Ultimately, early detection of AD could ensure timely prescription of DMTs to maximize their efficacy.
Acknowledgement: We would like to thank Prof. Bourdon (Dundee Cancer Centre, University of Dundee, Dundee, United Kingdom) for kindly providing the antibody mixture detecting all human p53 isoforms, Simone Cristoni from ISB for MS consultancy and testing, and Ismar Healthcare NV for the medical writing assistance in support of Diadem srl, Brescia, Italy.
Ethics approval and consent to participate: An appropriate institutional ethics committee approved the AIBL study, which followed relevant ethical regulations. Further information: Human Research Ethics Committee, Research Governance Unit, St Vincent’s Healthcare, Australia (no. 028/06). Individuals registered in AIBL and could opt out of PiB-PET. Their plasma samples are stored in the biobank and a subset of them was requested for this exploratory study.
Consent for publication: All authors agreed to the manuscript prior to submission.
Availability of data and materials: Data available on request from the authors.
Funding: This study was sponsored by Diadem SpA, Brescia, Italy. On its behalf, SP with PK developed the study design, SP overviewed the development of the protocol. As consultants supported by the sponsor, LVN and CX contributed to the biostatistics analyses. Diadem SpA, Brescia, Italy, owns the 2D3A8 antibody patent rights.
Conflict of interests: Dr. Kinnon reports personal fees from DIADEM during the conduct of the study; Dr. Piccirella reports personal fees from Diadem during the conduct of the study; Prof. Dr. Uberti reports financial support from Diadem SpA, outside the submitted work; and reports the following patents pending/issued: 0001348902, EP3201234B1, and PCTIB2019051785; Dr. Van Neste reports personal fees from DIADEM, during and outside the conduct of the study; Dr. Xiong reports personal fees from DIADEM outside the submitted work and serves on FDA’s Medical Imaging Product external advisory committee; Dr. Doecke, Dr. Fowler, Dr. Fripp, Prof. Masters have nothing to disclose.
Open Access: This article is distributed under the terms of the Creative Commons Attribution 4.0 International License (http://creativecommons.org/licenses/by/4.0/), which permits use, duplication, adaptation, distribution and reproduction in any medium or format, as long as you give appropriate credit to the original author(s) and the source, provide a link to the Creative Commons license and indicate if changes were made.
References
1. Aisen PS, Cummings J, Jack CR, Jr., et al. On the path to 2025: understanding the Alzheimer’s disease continuum. Alzheimers Res Ther 2017;9, 60.
2. Albert MS, DeKosky ST, Dickson D, et al. The diagnosis of mild cognitive impairment due to Alzheimer’s disease: recommendations from the National Institute on Aging-Alzheimer’s Association workgroups on diagnostic guidelines for Alzheimer’s disease. Alzheimers Dement 2011;7, 270-279.
3. Jack CR, Jr., Bennett DA, Blennow K, et al. NIA-AA research framework: Toward a biological definition of Alzheimer’s disease. Alzheimers Dement 2018;14, 535-562.
4. Salloway S, Sperling R, Fox NC, et al. Two phase 3 trials of bapineuzumab in mild-to-moderate Alzheimer’s disease. N Engl J Med 2014;370, 322-333.
5. Doody RS, Thomas RG, Farlow M, et al. Phase 3 trials of solanezumab for mild-to-moderate Alzheimer’s disease. N Engl J Med 2014;370, 311-321.
6. Buttini M, Masliah E, Barbour R, et al. Beta-amyloid immunotherapy prevents synaptic degeneration in a mouse model of Alzheimer’s disease. J Neurosci 2005;25, 9096-9101.
7. Rabinovici GD. Controversy and Progress in Alzheimer’s Disease – FDA Approval of Aducanumab. N Engl J Med, 2021. doi: 10.1056/NEJMp2111320. Online ahead of print.
8. Biogen Inc. ADUHELM™ (aducanumab-avwa): US prescribing information 2021. https://www.accessdata.fda.gov/scripts/cder/daf/. Accessed 18 Aug 2021.
9. Cummings J. The role of biomarkers in Alzheimer’s disease drug development. Adv Exp Med Biol 2019;1118, 29-61.
10. Frisoni GB, Boccardi M, Barkhof F, et al. Strategic roadmap for an early diagnosis of Alzheimer’s disease based on biomarkers. Lancet Neurol 2017;16, 661-676.
11. Dubois B, Hampel H, Feldman HH, et al. Preclinical Alzheimer’s disease: definition, natural history, and diagnostic criteria. Alzheimers Dement 2016;12, 292-323.
12. Bateman RJ, Xiong C, Benzinger TL, et al. Clinical and biomarker changes in dominantly inherited Alzheimer’s disease. N Engl J Med 2012;367, 795-804.
13. Merlo S, Spampinato SF, Sortino MA. Early compensatory responses against neuronal injury: A new therapeutic window of opportunity for Alzheimer’s disease? CNS Neurosci Ther 2019;25, 5-13.
14. Tonnies E, Trushina E. Oxidative Stress, Synaptic Dysfunction, and Alzheimer’s Disease. J Alzheimers Dis 2017;57, 1105-1121.
15. Arce-Varas N, Abate G, Prandelli C, et al. Comparison of Extracellular and Intracellular Blood Compartments Highlights Redox Alterations in Alzheimer’s and Mild Cognitive Impairment Patients. Curr Alzheimer Res 2017;14, 112-122.
16. Katsel P, Tan W, Fam P, Purohit DP, Haroutunian V. Cell cycle checkpoint abnormalities during dementia: A plausible association with the loss of protection against oxidative stress in Alzheimer’s disease [corrected]. PLoS One 2013;8, e68361.
17. Uberti D, Carsana T, Bernardi E, et al. Selective impairment of p53-mediated cell death in fibroblasts from sporadic Alzheimer’s disease patients. J Cell Sci 2002;115, 3131-3138.
18. Lanni C, Necchi D, Pinto A, et al. Zyxin is a novel target for beta-amyloid peptide: characterization of its role in Alzheimer’s pathogenesis. J Neurochem 2013;125, 790-799.
19. Lanni C, Nardinocchi L, Puca R, et al. Homeodomain interacting protein kinase 2: a target for Alzheimer’s beta amyloid leading to misfolded p53 and inappropriate cell survival. PLoS One 2010;5, e10171.
20. Uberti D, Cenini G, Olivari L, et al. Over-expression of amyloid precursor protein in HEK cells alters p53 conformational state and protects against doxorubicin. J Neurochem 2007;103, 322-333.
21. Stanga S, Lanni C, Sinforiani E, Mazzini G, Racchi M. Searching for predictive blood biomarkers: misfolded p53 in mild cognitive impairment. Curr Alzheimer Res 2012;9, 1191-1197.
22. Lanni C, Racchi M, Stanga S, et al. Unfolded p53 in blood as a predictive signature signature of the transition from mild cognitive impairment to Alzheimer’s disease. J Alzheimers Dis 2010;20, 97-104.
23. Lanni C, Racchi M, Mazzini G, et al. Conformationally altered p53: a novel Alzheimer’s disease marker? Mol Psychiatry 2008;13, 641-647.
24. Abate G, Vezzoli M, Polito L, et al. A conformation variant of p53 combined with machine learning identifies Alzheimer disease in preclinical and prodromal stages. J Pers Med 2020;11.
25. Piccirella S, Uberti D, Xiong C, et al. 2021. Performance of a Non-Invasive Blood Test for a Conformational Variant of p53 to Predict Alzheimer’s Disease Within 6 Years of Clinical Diagnosis. Preprints 2021050267, doi: 10.20944/preprints202105.0267.v1.
26. Fowler C, Rainey-Smith SR, Bird S, et al. Fifteen Years of the Australian Imaging, Biomarkers and Lifestyle (AIBL) Study: Progress and Observations from 2,359 Older Adults Spanning the Spectrum from Cognitive Normality to Alzheimer’s Disease. J Alzheimers Dis Rep 2021;5, 443-68.
27. Ellis KA, Bush AI, Darby D, et al. The Australian Imaging, Biomarkers and Lifestyle (AIBL) study of aging: methodology and baseline characteristics of 1112 individuals recruited for a longitudinal study of Alzheimer’s disease. Int Psychogeriatr 2009;21, 672-687.
28. Klunk WE, Koeppe RA, Price JC, et al. The Centiloid Project: Standardizing quantitative amyloid plaque estimation by PET. Alzheimer’s & Dementia 2015;11, 1-15.e4.
29. Gupta VB, Laws SM, Villemagne VL, et al. Plasma apolipoprotein E and Alzheimer disease risk: the AIBL study of aging. Neurology 2011;76,1091-1098.
30. Memo M and Uberti DL. Antibody binding a linear epitope of human p53 and diagnostic applications thereof, 2018. EP3201234. November 7.
31. Joruiz SM, Bourdon JC. p53 Isoforms: Key regulators of the cell fate decision. Cold Spring Harb Perspect Med , 2016;6.
32. Khoury MP, Bourdon JC. The isoforms of the p53 protein. Cold Spring Harb Perspect Biol 2010;2, a000927.
33. Blanche P, Dartigues J, Jacqmin-Gadda H. Estimating and Comparing time-dependent areas under receiver operating characteristic curves for censored event times with competing risks. Statistics in Medicine, 2013;32, 5381-5397.
34. Robin X, Turck N, Hainard A, et al. pROC: an open-source package for R and S+ to analyze and compare ROC curves. BMC Bioinformatics 2011;12, 77.
35. R Core Team, 2020. R: A language and environment for statistical computing. R Foundation for Statistical Computing, Vienna, Austria. URL https://www.R-project.org/.
36. Thiele C and Hirschfeld G. cutpointr: Improved Estimation and Validation of Optimal Cutpoints in R. J Stat Softw 2021;98.
37. Horgan D, Nobili F, Teunissen C, et al. Biomarker Testing: Piercing the Fog of Alzheimer’s and Related Dementia. Biomed Hub 2020;5, 1-22.
38. Labuschagne CF, Zani F, Vousden KH. Control of metabolism by p53 – Cancer and beyond. Biochim Biophys Acta Rev Cancer 2018;1870, 32-42.
39. Singh AK, Pati U (2015) CHIP stabilizes amyloid precursor protein via proteasomal degradation and p53-mediated trans-repression of beta-secretase. Aging Cell 2015;14, 595-604.
40. Silva AR, Santos AC, Farfel JM, et al. Repair of oxidative DNA damage, cell-cycle regulation and neuronal death may influence the clinical manifestation of Alzheimer’s disease. PLoS One 2014;9, e99897.
41. Merlo P, Frost B, Peng S, et al. p53 prevents neurodegeneration by regulating synaptic genes. Proc Natl Acad Sci U S A 2014;111, 18055-18060.
42. Tedeschi A, Nguyen T, Puttagunta R, Gaub P, Di Giovanni S. A p53-CBP/p300 transcription module is required for GAP-43 expression, axon outgrowth, and regeneration. Cell Death Differ 2009;16, 543-554.
43. Di Giovanni S, Knights CD, Rao M, et al. The tumor suppressor protein p53 is required for neurite outgrowth and axon regeneration. EMBO J 2006;25, 4084-4096.
44. Cenini G, Sultana R, Memo M, Butterfield DA. Effects of oxidative and nitrosative stress in brain on p53 proapoptotic protein in amnestic mild cognitive impairment and Alzheimer disease. Free Radic Biol Med 2008;45, 81-85.
45. Stevens KG, Pukala TL. Conjugating immunoassays to mass spectrometry: solutions to contemporary challenges in clinical diagnostics. Trends Analyt Chem 2020;132, 116064.
46. Oeckl P, Otto M. A review on MS-based blood biomarkers for Alzheimer’s disease. Neurol Ther 2019;8, 113-127.
47. Jannetto PJ, Fitzgerald RL. Effective use of mass spectrometry in the clinical laboratory. Clin Chem 2016;62, 92-98.
48. Shaffer JL, Petrella JR, Sheldon FC, et al. Predicting cognitive decline in subjects at risk for Alzheimer disease by using combined cerebrospinal fluid, MR imaging, and PET biomarkers. Radiology 2013;266, 583-591.
49. Shen XN, Li JQ, Wang HF, et al. Plasma amyloid, tau, and neurodegeneration biomarker profiles predict Alzheimer’s disease pathology and clinical progression in older adults without dementia. Alzheimers Dement (Amst) 2020;12, e12104.
50. Janelidze S, Mattsson N, Palmqvist S, et al. Plasma P-tau181 in Alzheimer’s disease: relationship to other biomarkers, differential diagnosis, neuropathology and longitudinal progression to Alzheimer’s dementia. Nat Med 2020;26, 379-386.
51. Stockmann J, Verberk IMW, Timmesfeld N, et al. Amyloid-beta misfolding as a plasma biomarker indicates risk for future clinical Alzheimer’s disease in individuals with subjective cognitive decline. Alzheimers Res Ther 2020;12, 169.
52. Reimand J, de Wilde A, Teunissen CE, et al. PET and CSF amyloid-β status are differently predicted by patient features: information from discordant cases. Alz Res Therapy 2019;11, 100. https://doi.org/10.1186/s13195-019-0561-5.