S.-Y. He1, W.-M. Su1, X.-J. Wen2, S.-J. Lu3, B. Cao1, B. Yan1, Y.-P. Chen1
1. Department of Neurology, West China Hospital, Sichuan University, Chengdu 610041, Sichuan, China; 2. West China School of Medicine, West China Hospital, Sichuan University, Chengdu, 610041, Sichuan, China; 3. Department of Respiratory, The Fourth People’s Hospital of Chengdu, Mental Health Center of Chengdu, Chengdu, 610036, Sichuan, China.
Corresponding Author: Yong-Ping Chen, Department of Neurology, West China Hospital, Sichuan University, Chengdu 610041, Sichuan, China. Tel: (86)-189-8060-6964. Email: Yongping Chen (yongping.chen@wchscu.edu.cn). Alternate corresponding author: Bo Yan, Department of Neurology, West China Hospital, Sichuan University, Chengdu 610041, Sichuan, China. Tel: (86)-189-8060-1689. Email: yanbo96@163.com
J Prev Alz Dis 2024;4(11):917-927
Published online May 29, 2024, http://dx.doi.org/10.14283/jpad.2024.100
Abstract
BACKGROUND: Alzheimer’s disease (AD) is a progressive neurodegenerative disorder characterized by intricate genetic and environmental etiology. The objective of this study was to identify robust non-genetic risk factors for AD through an updated umbrella review.
METHODS: We conducted a comprehensive search of meta-analyses and systematic reviews on non-genetic risk factors associated with AD in PubMed, Cochrane, Embase, and Ovid Medline up to June 30, 2023. After collecting data, we estimated the summary effect size and their 95% confidence intervals. The degree of heterogeneity between studies was assessed using I2 statistics and a 95% prediction interval was determined. Additionally, we evaluated potential excess significant bias and small study effects within the selected candidate studies.
RESULTS: The umbrella review encompassed a total of 53 eligible papers, which included 84 meta-analyses covering various factors such as lifestyle, diet, environmental exposures, comorbidity or infections, drugs, and biomarkers. Based on the evidence classification criteria employed in this study, two factors as convincing evidence (Class I), including rheumatoid arthritis (RA), potentially reduced the risk of AD, but diabetes significantly increased the risk of AD. Furthermore, three factors as highly suggestive evidence (Class II), namely depression, high homocysteine, and low folic acid level, potentially increased the risk of AD.
CONCLUSION: Our findings highlight several risk factors associated with AD that warrant consideration as potential targets for intervention. However, it is crucial to prioritize the identified modifiable risk factors, namely rheumatoid arthritis, diabetes, depression, elevated homocysteine levels, and low folic acid levels to effectively address this complex neurodegenerative disorder.
Key words: Alzheimer’s disease, modifiable risk factors, meta-analysis, umbrella review.
Introduction
Alzheimer’s disease (AD) is an insidious and irreversible neurodegenerative disorder, which represents the main etiology of dementia clinically characterized by memory loss, cognitive decline, behavior changes, and functional impairment (1). Currently, the prevalence of AD is 4%~7% in the global elderly population over 65 years old, and the prevalence of AD can be as high as 20%~30% in the elderly population over 85 years old (2). Based on the 2020 Alzheimer’s disease facts and figures, the number of AD patients in the United States could increase significantly from 5.8 million to 13.8 million by 2050. Over the past few decades, community surveys in Japan and China have found a significant increase in the prevalence of AD (3). Consequently, this exacerbates both the economic burden on AD patients and their families’ caregiving responsibilities (4).
The etiology of AD is multifaceted and intricate, with the main pathologic features of amyloid plaque formation and neurofibrillary tangles (5, 6). While familial AD accounts for a portion of patients, approximately one-third of AD cases may be attributed to non-genetic modifiable factors such as cardiovascular diseases, diabetes, smoking, alcohol consumption, physical activity, and so on (6, 7). Given that there is currently no effective treatment for AD, it is imperative to identify the risk factors associated with AD and address them in order to prevent or delay the onset of this debilitating condition. In Western countries, the prevalence and incidence of dementia appear to be declining due to insights gained from several population-based longitudinal studies focusing on modifiable factors (8-12). For instance, randomized clinical trials have demonstrated that engaging in regular physical activity can enhance cognitive function and mitigate the rate of cognitive decline (13), shedding light on a potential association between physical activity and AD. However, it is crucial for clinical trials to consider the characteristics of the included population as well as employ long-term follow-up strategies given the slow progression nature of AD. Furthermore, most trials primarily focus on assessing cognitive decline rather than disease progression. To address this matter comprehensively, numerous systematic reviews and meta-analyses have been conducted to analyze the risk factors associated with AD (14-16). Nevertheless, these studies failed to offer a comprehensive evaluation and consistent recommendations regarding the risk factors associated with AD.
Umbrella reviews, which systematically evaluate meta-analyses and/or systematic reviews, encompass multiple comparisons within the same disease and possess a higher level of evidence classification. Therefore, we selected this approach to summarize and update non-genetic risk factors associated with AD based on established guidelines (17), aiming to arrive at a conclusion by integrating all available meta-analyses and systematic reviews. Subsequently, robust associations between these factors and AD were also identified.
Methods
The protocol for this study was preregistered in the International Prospective Register of Systematic Reviews (PROSPERO) under the ID number CRD42023454097. This umbrella review was conducted in accordance with the guidelines (17) and followed the updated Preferred Reporting Items for Systematic Reviews and Meta-analysis (18).
Search strategy
We conducted a comprehensive search on PubMed, Cochrane, Embase, and Ovid Medline up to June 30, 2023, in order to identify meta-analyses and systematic reviews of observational studies investigating the associations between potential biomarkers or environmental risk factors for AD. Our search strategy employed the terms (Alzheimer* OR dementia) AND (meta-analyses OR systematic review) AND risk, with each term limited to abstracts or titles. Two independent investigators (SYH and WMS) were responsible for meticulously reviewing the full text of potentially eligible articles.
Eligibility criteria
We included meta-analyses of observational studies investigating risk factors associated with AD, including prospective cohort studies, case-control studies, and cross-sectional studies. Firstly, these meta-analyses were required to report the effect size, 95% confidence interval (95% CI), and any potential publication bias either in the text or graph. Secondly, only one meta-analysis with the largest number of primary studies or the most recent update was selected for each exposure to avoid duplicate studies concerning the same factor.
If the articles exclusively investigated non-modifiable genetic factors associated with AD, they were excluded. Meta-analyses solely focusing on imaging, diagnostic, prognostic, and other non-observational studies were also excluded. Additionally, articles that primarily examined outcomes related to mild cognitive impairment, cognitive impairment severity, and symptom progression of AD rather than AD risk were excluded as well. Lastly, articles where results for AD could not be extracted from subgroups in patients with dementia were also excluded.
Quality assessment and data extraction
The methodological quality of the included articles was assessed using the Measurement Tool for Assessing Systematic Reviews (AMSTAR), a valid and reliable measurement tool for assessing the quality of systematic reviews and meta-analyses (19). Two reviewers (SYH and WMS) conducted the assessment. The included studies were categorized as high quality (scores 8-11), medium quality (scores 4-7), or low quality (scores 0-3) (20). Subsequently, we employed the Grading of Recommendations, Assessment, Development and Evaluation (GRADE) framework to evaluate the quality of evidence (21), which classified it as “high”, “moderate”, “low”, or “very low”.
Two independent reviewers conducted the study selection and data extraction processes. Relevant information, such as first author, publication year, factors studied, and number of AD cases were extracted from eligible meta-analyses and systematic reviews. Additionally, we retrieved risk estimates such as odds ratio (OR), hazard ratio (HR), and risk ratio (RR), along with their corresponding 95%CI for each study. Quality assessment of the studies was noted when available. In cases where multiple control groups were used, data from healthy groups took priority.
Statistical analysis
The pooled effect size and the 95%CI were estimated for each meta-analysis using both random-effects and fixed-effects models (22). In cases involving continuous data, the standard mean difference (SMD) was converted to an odds ratio (OR) by multiplying it by π/√3 (23, 24). Additionally, a 95% prediction interval (PI), accounting for variation in different settings, was calculated (25, 26). If the 95% PI crosses the null value, it indicates that these factors may not have an effect or even have an opposite effect. To estimate OR, RR, and HR accurately, natural logarithm scale transformations were applied. For calculation purposes, the standard error (SE) of the pooled effect size (M) and the heterogeneity estimate (τ2) are required. The 95% PI is calculated as log M ± t1-0.05/2, k-1 × √(τ2+SE2) (26). An approximation was made for the SE of the effect size in the largest study of each meta-analysis. If SE < 0.10, we evaluated whether the difference between the effect estimation and the lower or upper 95% CI was less than 0.20 to ascertain the presence of a small study effect. Heterogeneity among studies was quantified using the I2 metric (27). I2 ranges from 0% to 100% and represents the proportion of variation in effect estimation due to heterogeneity rather than sampling error, which is commonly calculated in most published meta-analyses (28). I2 > 50% or > 75% indicates large or very large heterogeneity, respectively.
The presence of small study effects, which may contribute to publication bias, was assessed using Egger’s test and visualized on a funnel plot. A p-value greater than 0.10 indicated that the degree of asymmetry was small and non-significant (29, 30). Conversely, it indicated the presence of small study effects, particularly in larger studies with more conservative effects. Additionally, we employed the excess statistical significance test to assess if the number of observed studies (O) with positive results (p < 0.05) exceeded the expected number (E) (31). By utilizing effect size, 95% CI, and standard error of studies in each meta-analysis, we calculated each study power through a power-enhanced funnel plot (31, 32). When a two-tailed p-value was less than 0.10 and O surpassed E, it indicated an excess of observed studies compared to what was expected.
Assessment of Epidemiological Credibility
Based on the classification of evidence from previous studies (33, 34), we categorized the identified factors into five distinct classes: Class I (convincing evidence), Class II (highly suggestive evidence), Class III (suggestive evidence), Class IV (weak evidence), and NS (non-significant). Detailed criteria for the classification of evidence are presented in Table 2.
Although certain associations were supported as Class I or Class II evidence, it is imperative to acknowledge that this does not establish a causal relationship between risk factors and the disease. Therefore, we must meticulously scrutinize these two levels of evidence. The statistical analysis and the power evaluation were conducted utilizing Stata 17.0 and R 4.3.1.
Results
Search results
After conducting an extensive search of a total of 8684 articles, we identified 53 articles that met our criteria (Fig. 1). These 53 articles encompassed a cumulative total of 84 meta-analyses, which included 892 observational studies and were published between 2013 and 2023. The 84 meta-analyses examined various types of risk factors, including habits (9), dietary factors (6), environmental exposures (8), diseases (26), drugs (7), and biomarkers (28). Notably, among these meta-analyses, 60 had sample sizes exceeding 1000 cases (Table 1). Importantly, the eligible meta-analyses did not have direct access to individual participant data; instead, they solely relied on summary-level information derived from published research.
*When articles are screened by title and abstract, they are excluded because of non-observational studies, non-human studies, genetic studies, or studies’ outcome other than risk of AD.
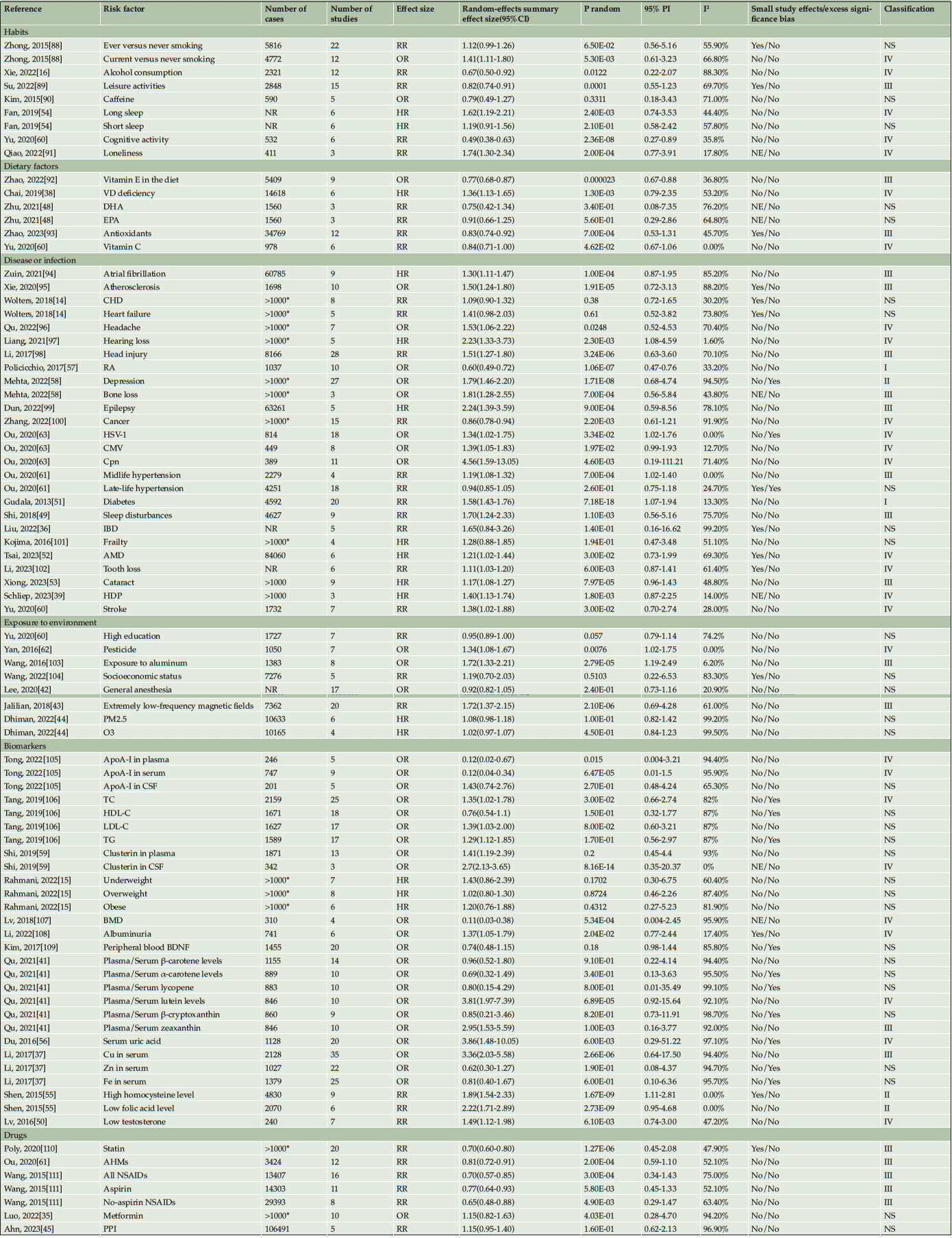
Table 1. Quantitative synthesis of 84 meta-analyses in 53 eligible articles investigating risk factors with Alzheimer’s disease
For biomarkers, ApoA-I and clusterin were classified by plasma and CSF respectively. Fat, carotenoid, homocysteine, folic acid, and metals such as Cu, Zn, and Fe were analyzed from low to high levels. Other evidence including drugs, exposure, and diseases were mostly analyzed by yes and no. TC: total cholesterol, TG: total triglycerides, HDL-C: high-density lipoprotein, LDL-C: low-density lipoprotein, CHD: coronary heart disease, ApoA-I: Napoli protein A1, BMI: body mass index, RA: rheumatoid arthritis, BMD: bone mineral density, HSV-1: herpes simplex virus, CMV: cytomegalovirus, Cpn: chlamydia pneumonia, AHMs: anti-hypertensive medications, NSAIDs: non-steroidal anti-inflammatory drugs, IBD: inflammatory bowel disease, VD: vitamin D, GA: general anesthesia, PM2.5: particulate matter, O3: ozone, DHA: docosahexaenoic acid, EPA: eicosapentaenoic acid, AMD: age-related macular degeneration, PPI: proton pump inhibitors, HDP: hypertensive disorders of pregnancy, HR: hazard ratio, OR: odds ratio, RR: relative risk, PI: prediction interval. *For some meta-analyses, the authors conducted associations with AD and dementia, We can only roughly judge that the sample size may be greater than 1000.
Quality assessment
Among the 53 articles, a total of 34 articles employed the Newcastle-Ottawa Scale (NOS) for qualitative assessment, encompassing 496 studies. Out of these studies, 70 (14%) were classified as high quality (NOS score = 9), while moderate quality was observed in 270 (54%) studies with NOS scores ranging from 7 to 8. Additionally, low quality was identified in 156 (32%) studies with NOS scores below 7. Five articles (35-39) utilized both the NOS and either the Agency for Healthcare Research (40) and Quality or the Cochrane Risk of Bias tool for assessment purposes. Furthermore, five articles (41-45) employed alternative evaluation tools such as the Hill Criteria (46) and open-access Joanna Briggs Institute criteria (47) among others. Moreover, seven articles (48-54) did not provide sufficient data on quality assessment, and two publications (55, 56) refrained from utilizing any assessment tool due to potential bias concerns (Supplementary Table 1).
Characteristics and Quantitative Analysis
After summarizing these data, statistically significant effects (p < 0.05) were observed in 53 out of the 84 meta-analyses conducted using the random-effects model. Among these, 27 demonstrated highly significant effects at p < 0.001 (Table 2). Importantly, seven factors, namely rheumatoid arthritis (RA) (57), diabetes (51), depression (58), homocysteine (55), folic acid (55), clusterin in cerebrospinal fluid (59), and cognitive activity (60), exhibited remarkably significant effects at p < 10-6 (Table 1, Supplementary Table 2). Notably, among all associations examined, nine risk factors including RA (57), diabetes (51), homocysteine (55), vitamin E supplementation, midlife hypertension (61), extremely low-frequency magnetic fields (43), exposure to pesticide (62), HSV-1 infection (63), and testosterone levels (50) showed a strong association with AD as indicated by their respective 95% PI excluding the null value (Table 1).
TC: total cholesterol, TG: total triglycerides, HDL-C: high-density lipoprotein, LDL-C: low-density lipoprotein, CHD: coronary heart disease, ApoA-I: Napoli protein A1, BMI: body mass index, RA: rheumatoid arthritis, BMD: bone mineral density, HSV-1: herpes simplex virus, CMV: cytomegalovirus, Cpn: chlamydia pneumonia, AHMs: anti-hypertensive medications, NSAIDs: non-steroidal anti-inflammatory drugs, IBD: inflammatory bowel disease, VD: vitamin D, GA: general anesthesia, PM2.5: particulate matter, O3: ozone, DHA: docosahexaenoic acid, EPA: eicosapentaenoic acid, AMD: age-related macular degeneration, PPI: proton pump inhibitors, HDP: hypertensive disorders of pregnancy
Bias assessment
Furthermore, among the 84 meta-analyses conducted, negligible heterogeneity estimates were observed in 27 (32%) cases, while moderate heterogeneity (I2 ≥ 50%, but ≤ 75%) was exhibited in 22 (26%) cases and substantial heterogeneity (I2 > 75%) was displayed in 35 (42%) cases (Supplementary Table 3). Moreover, small study effects of evidence were observed in 14 (17%) meta-analyses, and excess significant bias was noted in 13 (15%) meta-analyses. However, for six (7%) meta-analyses, the presence of small study effects could not be estimated due to the inclusion of fewer than three studies.
Quantitative Synthesis with Evidence Classification
As previously mentioned above, among the 84 meta-analyses, 7 (8%) demonstrated a significant association with AD at p < 10-6 under the random-effects model (Table 1, Supplementary Table 2). The median AMSTAR score for all evidence was 8.6 (range: 6-11). Detailed criteria and scores for the AMSTAR assessment are presented in Supplementary Table 4. Due to the inclusion of observational studies and factors that degrade quality, such as inconsistency, indirectness, imprecision, significant risk of bias, and publication bias, all evaluated evidence using GRADE was categorized as “low” or “very low” quality (Supplementary Table 5). After applying the evidence classification criteria to assess these risk factors, most of them were classified as Class III (21, 25%), IV (26, 31%), or NS (32, 38%) (Table 2, Supplementary Table 6). However, it is important to note five factors classified as Class I and Class II evidence (Table 3). Among these factors, RA and diabetes, were evaluated as having a “low” quality but demonstrated an association based on criteria including more than1000 cases, p < 10-6, no small study effects or excess significant bias, the 95% PI excluded null value, and non-significant heterogeneity. Additionally, the three other risk factors namely depression (low), homocysteine (very low), and folic acid (very low) were classified as Class II evidence due to meeting criteria such as having more than1000 cases, p < 10-6, and exhibiting the largest study with a 95% CI excluding null value.

Table 3. Quantitative synthesis of five associations with Alzheimer’s disease with convincing or highly suggestive evidence
RA: rheumatoid arthritis, CHD: coronary heart disease; OR: odds ratio, RR: relative risk, PI: prediction interval
Discussion
Here, we performed an umbrella review to establish a definitive correlation between biomarkers, environmental risk factors, and AD. Through meticulous quantitative synthesis and bias assessment, two factors pertaining to RA and diabetes were classified as Class I evidence for their associations with AD. Meanwhile, three factors (depression, elevated homocysteine levels and insufficient folic acid) were categorized as Class II evidence despite the presence of cases exceeding 1000 and p-values less than 10-6; however, it is worth noting that there may exist substantial heterogeneity in these studies along with a 95% PI encompassing the null hypothesis. Additionally, potential small-study effects and/or excess significance bias cannot be disregarded.
Two factors, RA and diabetes, presented Class I evidence for an association with AD. In a meta-analysis of two population-based and eight case-control studies, RA (57) was found to significantly reduce the risk of AD (OR = 0.60, 95% CI 0.49-0.72, p= 1.06*10-7), demonstrating low overall heterogeneity (I2 = 33.2%) and no evidence of bias according to Egger’s tests. Bae et al.’s Mendelian randomization study (64) further supported a negative correlation between RA and AD by leveraging cross-ethnic genome-wide association study meta-analysis data from participants in Europe and Asia. Additionally, previous research has shown that RA-induced production of granulocyte-macrophage colony-stimulating factor can stimulate the proliferation of monocytes and granulocytes derived from peripheral bone marrow as well as bone marrow-derived microglia (65). Interestingly, these microglia have been observed to reduce amyloid deposition in mouse models of AD (66), suggesting potential long-term protective effects against cognitive impairment. Furthermore, Trzeciak et al. highlighted the similarity in pathological mechanisms between AD and RA regarding immune system activation (67). Therefore, it is plausible that cytokines produced during RA may mediate certain processes while also potentially impacting the blood-brain barrier. Cytokine-mediated amyloid degradation observed in RA could disrupt the bone-joint bond through complex mechanisms that may be linked to Aβ plaques.
The association between diabetes and AD was found to be statistically significant with a low p-value
(7.18*10-18). A meta-analysis of 20 prospective cohort studies on diabetes reported an increased risk of AD (RR = 1.58, 95% CI 1.43-1.76). Hyperglycemia in diabetic patients leads to the generation of advanced glycation end-products (AGEs) in the brain, which have been identified as important contributors to neurodegeneration (51). AGEs can accelerate amyloid-β aggregation, thereby establishing a link between diabetes and AD (68). Additionally, oxidative stress and neuroinflammation have also been implicated in the development of AD under hyperglycemic conditions (51, 69). Moreover, previous studies have demonstrated that insulin stimulates the release of Aβ into the extracellular space and enhances the expression of insulin-degrading enzyme (IDE), which plays a crucial role in Aβ degradation (70, 71). Interestingly, Michailidis et al. (72) posed a hypothesis that AD, a type 3 diabetes, is a metabolic disease caused by insulin resistance because they are interlinked with the above mechanisms. Not only that, amyloid peptide, which is also called amylin, is produced with β-islet cells and co-secreted with insulin. So, it can be overproduced in T2D and cause insulin resistance in the brain over time, which consequently induce Aβ deposition, Tau phosphorylation, and inflammation (73). However, it is noteworthy that the efficacy of insulin in diabetic patients is relatively limited. Therefore, these aforementioned mechanisms could potentially elucidate the association between diabetes and AD. Implementing lifestyle modifications aimed at preventing individuals at risk of developing diabetes may yield substantial societal benefits by mitigating cognitive decline.
Three factors, namely depression, high homocysteine levels, and low folic acid levels, were classified as Class II evidence for association. The heterogeneity estimate of homocysteine and folic acid levels was not significant (I2 < 50%), and the former 95% PI excluded the null value; however, these two factors may have some study bias. High homocysteine levels (Hcy) and low folic acid levels as biomarkers including nine case-control studies that showed a significant association with the development of AD (55). Firstly, a low level of folic acid might impair vitamin B12 intake and degrade cognitive function by aggravating inflammatory reactions (74). Reduced folic acid also damages antioxidant activity in different aspects and may increase homocysteine levels (75). Secondly, homocysteine is an excitatory amino acid that causes cell death by acting on NMDA receptors (76). Homocysteine thiolactone, the metabolite of Hcy, is considered one cause of cellular Hcy toxicity through protein covalent modification inducing protein N-homocysteylation and amyloid formation (77, 78). Li et al. (79) demonstrated that hyperhomocysteinemia epigenetically controls gene expression in the amyloid pathway increasing amyloid deposition in the brain along with phosphorylated tau production (p-Tau), leading to hippocampal neuron death as well as various cognitive deficits. However, more studies are still needed to clarify the relationship between homocysteine/folic acid/vitamin B12 and AD.
The association between depression and risk for AD remained significant, although there may be a bi-directional relationship between them. Studies have suggested that patients with depression may exhibit overperfusion and atrophy in specific brain regions (i.e., anterior cingulate cortex, anterior cuneiform, and parietal lobule) (80), as well as alterations in NMDA receptors (81). These chronic changes could potentially lead to neurodegeneration and the development of AD. Another study (82) demonstrated that depression is accompanied by neuroinflammation, which can activate astrocytes and contribute to amyloid aggregation and p-Tau accumulation. This process is believed to accelerate brain aging and increase the risk of AD (83). On the other hand, several studies (58, 83) have proposed that depression should not only be considered a risk factor for AD but also regarded as a prodromal symptom of AD. It should be given special attention, particularly in older patients. Additionally, due to cognitive decline and decreased self-care abilities later in life, AD can also affect mood and lead to depressive symptoms (84). Therefore, antidepressants may hold potential as a treatment option for AD (85). Further research is needed to fully understand the relationship between depression and AD.
Strengths and limitations
The strengths of our review lie in the utilization of standardized methods and statistical approaches to conduct an umbrella review, providing a comprehensive summary of current evidence on the association between non-genetic risk factors and AD. This methodology has been previously employed to investigate the credibility of risk factors in relation to other neurodegenerative diseases (86, 87). Additionally, we applied three standard methods (AMSTAR, GRADE, and the evidence classification criteria) to assess the methodological quality, evidence quality, and classification for each risk factor meta-analysis. Upon comparison, inconsistencies were observed between the results derived from GRADE and those obtained using evidence classification criteria. However, it is important to consider that GRADE entails some subjectivity while evidence classification criteria offer a more objective approach; therefore both methods should be taken into account.
However, there are several limitations that need to be acknowledged. Firstly, umbrella reviews require the inclusion of all relevant studies pertaining to the selected topic, which inevitably increases workload and time consumption (17). Secondly, our inclusion criteria encompassed cohort, case-control, or cohort studies that inherently carry a risk of bias. Moreover, it is challenging to address potential issues present in individual systematic reviews such as bias in meta-analysis evaluation due to small sample size or study size (25), which may introduce biases into the evidence classification process. Despite these considerations, we ensured an adequate number of studies were included and the majority of meta-analyses chosen employed NOS for appraising observational studies. Additionally, each meta-analysis was assessed using AMSTAR with most scoring 8 points or higher. In cases where multiple studies discussed the relationship between the same factor and AD, preference was given to meta-analyses with larger sample sizes or more recent studies. Furthermore, statistical methods were extensively utilized for re-evaluating effect sizes under random or fixed-effect models and assessing study bias.
Conclusion
Based on the evidence classification criteria, we have identified five factors (RA, diabetes, depression, homocysteine levels, and folic acid) that exhibit a robust correlation with the occurrence of AD. These factors can be targeted for improvement through public health policy measures or other interventions. However, it should be noted that while observational evidence has provided convincing or highly suggestive links between modifiable risk factors and AD, translating these findings into tangible benefits for AD patients requires further efforts.
Contributors: YPC and BY designed and supervised the project. SYH selected the articles, extracted and cross-checked the data, contributed to the statistical analysis and wrote the first draft of the manuscript. WMS and SYH selected the articles and extracted and cross-checked the data. XJW, SJL and BC contributed to the statistical analysis. YSH, BY and YPC wrote, revised and discussed the final edition. All authors read and approved the final version of the manuscript.
Funding: This study was supported by the National Natural Science Fund of Sichuan (Grant no. 2022NSFSC0749 to B.C.), the 1·3·5 project for disciplines of excellence–Clinical Research Fund, West China Hospital, Sichuan University (Grant No. 2023HXFH032 to Y.P.C.), the Science and Technology Bureau Fund of Sichuan Province (Grant no 2023YFS0269 to Y.P.C.), and the National Innovation and Entrepreneurship Training Program for College Students (Grant No. C2023124417 to X.J.W.).
Declaration of Competing Interest: The authors have nothing to disclose.
Patient consent for publication: Not required.
Open Access: This article is distributed under the terms of the Creative Commons Attribution 4.0 International License (http://creativecommons.org/licenses/by/4.0/), which permits use, duplication, adaptation, distribution and reproduction in any medium or format, as long as you give appropriate credit to the original author(s) and the source, provide a link to the Creative Commons license and indicate if changes were made.
References
1. Soria Lopez JA, González HM, Léger GC: Alzheimer’s disease. Handb Clin Neurol 2019, 167:231-255. doi.10.1016/b978-0-12-804766-8.00013-3.
2. Zhang XX, Tian Y, Wang ZT, Ma YH, Tan L, Yu JT: The Epidemiology of Alzheimer’s Disease Modifiable Risk Factors and Prevention. J Prev Alzheimers Dis 2021, 8(3):313-321. doi.10.14283/jpad.2021.15.
3. Association As: 2023 Alzheimer’s disease facts and figures. Alzheimers Dement 2023, 19(4):1598-1695. doi.10.1002/alz.13016.
4. Pudelewicz A, Talarska D, Bączyk G: Burden of caregivers of patients with Alzheimer’s disease. Scand J Caring Sci 2019, 33(2):336-341. doi.10.1111/scs.12626.
5. Khan S, Barve KH, Kumar MS: Recent Advancements in Pathogenesis, Diagnostics and Treatment of Alzheimer’s Disease. Curr Neuropharmacol 2020, 18(11):1106-1125. doi.10.2174/1570159×18666200528142429.
6. Barnes DE, Yaffe K: The projected effect of risk factor reduction on Alzheimer’s disease prevalence. Lancet Neurol 2011, 10(9):819-828. doi.10.1016/s1474-4422(11)70072-2.
7. Lane CA, Hardy J, Schott JM: Alzheimer’s disease. Eur J Neurol 2018, 25(1):59-70. doi.10.1111/ene.13439.
8. Wiberg P, Waern M, Billstedt E, Ostling S, Skoog I: Secular trends in the prevalence of dementia and depression in Swedish septuagenarians 1976-2006. Psychol Med 2013, 43(12):2627-2634. doi.10.1017/s0033291713000299.
9. Serrano-Pozo A, Growdon JH: Is Alzheimer’s Disease Risk Modifiable? J Alzheimers Dis 2019, 67(3):795-819. doi.10.3233/jad181028.
10. Schrijvers EM, Verhaaren BF, Koudstaal PJ, Hofman A, Ikram MA, Breteler MM: Is dementia incidence declining?: Trends in dementia incidence since 1990 in the Rotterdam Study. Neurology 2012, 78(19):1456-1463. doi.10.1212/WNL.0b013e3182553be6.
11. Matthews FE, Arthur A, Barnes LE, Bond J, Jagger C, Robinson L, Brayne C: A two-decade comparison of prevalence of dementia in individuals aged 65 years and older from three geographical areas of England: results of the Cognitive Function and Ageing Study I and II. Lancet 2013, 382(9902):1405-1412. doi.10.1016/s0140-6736(13)61570-6.
12. Langa KM, Larson EB, Crimmins EM, Faul JD, Levine DA, Kabeto MU, Weir DR: A Comparison of the Prevalence of Dementia in the United States in 2000 and 2012. JAMA Intern Med 2017, 177(1):51-58. doi.10.1001/jamainternmed.2016.6807.
13. Lautenschlager NT, Cox KL, Flicker L, Foster JK, van Bockxmeer FM, Xiao J, Greenop KR, Almeida OP: Effect of physical activity on cognitive function in older adults at risk for Alzheimer disease: a randomized trial. Jama 2008, 300(9):1027-1037. doi.10.1001/jama.300.9.1027.
14. Wolters FJ, Segufa RA, Darweesh SKL, Bos D, Ikram MA, Sabayan B, Hofman A, Sedaghat S: Coronary heart disease, heart failure, and the risk of dementia: A systematic review and meta-analysis. Alzheimers Dement 2018, 14(11):1493-1504. doi.10.1016/j.jalz.2018.01.007.
15. Rahmani J, Roudsari AH, Bawadi H, Clark C, Ryan PM, Salehisahlabadi A, Rahimi Sakak F, Goodarzi N, Razaz JM: Body mass index and risk of Parkinson, Alzheimer, Dementia, and Dementia mortality: a systematic review and dose-response meta-analysis of cohort studies among 5 million participants. Nutr Neurosci 2022, 25(3):423-431. doi.10.1080/1028415x.2020.1758888.
16. Xie C, Feng Y: Alcohol consumption and risk of Alzheimer’s disease: A dose-response meta-analysis. Geriatr Gerontol Int 2022, 22(4):278-285. doi.10.1111/ggi.14357.
17. Fusar-Poli P, Radua J: Ten simple rules for conducting umbrella reviews. Evid Based Ment Health 2018, 21(3):95-100. doi.10.1136/ebmental-2018-300014
18. Page MJ, McKenzie JE, Bossuyt PM, Boutron I, Hoffmann TC, Mulrow CD, Shamseer L, Tetzlaff JM, Akl EA, Brennan SE et al: The PRISMA 2020 statement: an updated guideline for reporting systematic reviews. Bmj 2021, 372:n71. doi.10.1136/bmj.n71.
19. Shea BJ, Grimshaw JM, Wells GA, Boers M, Andersson N, Hamel C, Porter AC, Tugwell P, Moher D, Bouter LM: Development of AMSTAR: a measurement tool to assess the methodological quality of systematic reviews. BMC Med Res Methodol 2007, 7:10. doi.10.1186/1471-2288-7-10.
20. Xiu-xia L, Ya Z, Yao-long C, Ke-hu Y, Zong-jiu Z: The reporting characteristics and methodological quality of Cochrane reviews about health policy research. Health Policy 2015, 119(4):503-510. doi.10.1016/j.healthpol.2014.09.002.
21. Guyatt G, Oxman AD, Akl EA, Kunz R, Vist G, Brozek J, Norris S, Falck-Ytter Y, Glasziou P, DeBeer H et al: GRADE guidelines: 1. Introduction-GRADE evidence profiles and summary of findings tables. J Clin Epidemiol 2011, 64(4):383-394. doi.10.1016/j.jclinepi.2010.04.026.
22. Lau J, Ioannidis JP, Schmid CH: Quantitative synthesis in systematic reviews. Ann Intern Med 1997, 127(9):820-826. doi.10.7326/0003-4819-127-9-199711010-00008.
23. Murad MH, Wang Z, Chu H, Lin L: When continuous outcomes are measured using different scales: guide for meta-analysis and interpretation. Bmj 2019, 364:k4817. doi.10.1136/bmj.k4817.
24. Chinn S: A simple method for converting an odds ratio to effect size for use in meta-analysis. Stat Med 2000, 19(22):3127-3131. doi.10.1002/1097-0258(20001130)19:22<3127::aid-sim784>3.0.co;2-m.
25. Higgins JP, Thompson SG, Spiegelhalter DJ: A re-evaluation of random-effects meta-analysis. J R Stat Soc Ser A Stat Soc 2009, 172(1):137-159. doi.10.1111/j.1467-985X.2008.00552.x.
26. IntHout J, Ioannidis JP, Rovers MM, Goeman JJ: Plea for routinely presenting prediction intervals in meta-analysis. BMJ Open 2016, 6(7):e010247. doi.10.1136/bmjopen-2015-010247.
27. Langan D: Assessing Heterogeneity in Random-Effects Meta-analysis. Methods Mol Biol 2022, 2345:67-89. doi.10.1007/978-1-0716-1566-9_4.
28. Higgins JP, Thompson SG: Quantifying heterogeneity in a meta-analysis. Stat Med 2002, 21(11):1539-1558. doi.10.1002/sim.1186.
29. Egger M, Smith GD: Bias in location and selection of studies. Bmj 1998, 316(7124):61-66. doi.10.1136/bmj.316.7124.61.
30. Egger M, Davey Smith G, Schneider M, Minder C: Bias in meta-analysis detected by a simple, graphical test. Bmj 1997, 315(7109):629-634. doi.10.1136/bmj.315.7109.629.
31. Ioannidis JPA: Clarifications on the application and interpretation of the test for excess significance and its extensions. Journal of Mathematical Psychology 2013, 57(5):184-187. doi.10.1016/j.jmp.2013.03.002
32. Ioannidis JP, Trikalinos TA: An exploratory test for an excess of significant findings. Clin Trials 2007, 4(3):245-253. doi.10.1177/1740774507079441.
33. Johnson VE: Revised standards for statistical evidence. Proc Natl Acad Sci U S A 2013, 110(48):19313-19317. doi.10.1073/pnas.1313476110.
34. Ioannidis JP: Integration of evidence from multiple meta-analyses: a primer on umbrella reviews, treatment networks and multiple treatments meta-analyses. Cmaj 2009, 181(8):488-493. doi.10.1503/cmaj.081086.
35. Luo A, Ning P, Lu H, Huang H, Shen Q, Zhang D, Xu F, Yang L, Xu Y: Association Between Metformin and Alzheimer’s Disease: A Systematic Review and Meta-Analysis of Clinical Observational Studies. J Alzheimers Dis 2022, 88(4):1311-1323. doi.10.3233/jad-220180.
36. Liu N, Wang Y, He L, Sun J, Wang X, Li H: Inflammatory bowel disease and risk of dementia: An updated meta-analysis. Front Aging Neurosci 2022, 14:962681. doi.10.3389/fnagi.2022.962681.
37. Li DD, Zhang W, Wang ZY, Zhao P: Serum Copper, Zinc, and Iron Levels in Patients with Alzheimer’s Disease: A Meta-Analysis of Case-Control Studies. Front Aging Neurosci 2017, 9:300. doi.10.3389/fnagi.2017.00300.
38. Chai B, Gao F, Wu R, Dong T, Gu C, Lin Q, Zhang Y: Vitamin D deficiency as a risk factor for dementia and Alzheimer’s disease: an updated meta-analysis. BMC Neurol 2019, 19(1):284. doi.10.1186/s12883-019-1500-6.
39. Schliep KC, McLean H, Yan B, Qeadan F, Theilen LH, de Havenon A, Majersik JJ, Ostbye T, Sharma S, Varner MW: Association Between Hypertensive Disorders of Pregnancy and Dementia: a Systematic Review and Meta-Analysis. Hypertension 2023, 80(2):257-267. doi.10.1161/HYPERTENSIONAHA.122.19399.
40. Jue JJ, Cunningham S, Lohr K, Shekelle P, Shiffman R, Robbins C, Nix M, Coates V, Schoelles K: Developing and Testing the Agency for Healthcare Research and Quality’s National Guideline Clearinghouse Extent of Adherence to Trustworthy Standards (NEATS) Instrument. Ann Intern Med 2019, 170(7):480-487. doi.10.7326/m18-2950.
41. Qu M, Shi H, Wang K, Wang X, Yu N, Guo B: The Associations of Plasma/Serum Carotenoids with Alzheimer’s Disease: A Systematic Review and Meta-Analysis. J Alzheimers Dis 2021, 82(3):1055-1066. doi.10.3233/jad-210384.
42. Lee JJ, Choi GJ, Kang H, Baek CW, Jung YH, Shin HY, Park YH, Woo YC: Relationship between Surgery under General Anesthesia and the Development of Dementia: A Systematic Review and Meta-Analysis. Biomed Res Int 2020, 2020:3234013. doi.10.1155/2020/3234013.
43. Jalilian H, Teshnizi SH, Röösli M, Neghab M: Occupational exposure to extremely low frequency magnetic fields and risk of Alzheimer disease: A systematic review and meta-analysis. Neurotoxicology 2018, 69:242-252. doi.10.1016/j.neuro.2017.12.005.
44. Dhiman V, Trushna T, Raj D, Tiwari RR: Is Air Pollution Associated with Increased Risk of Dementia? A Meta-Analysis of Epidemiological Research. Neurol India 2022, 70(3):1004-1019. doi.10.4103/0028-3886.349654.
45. Ahn N, Nolde M, Krause E, Güntner F, Günter A, Tauscher M, Gerlach R, Meisinger C, Linseisen J, Baumeister SE et al: Do proton pump inhibitors increase the risk of dementia? A systematic review, meta-analysis and bias analysis. Br J Clin Pharmacol 2023, 89(2):602-616. doi.10.1111/bcp.15583.
46. Repacholi MH, Lerchl A, Röösli M, Sienkiewicz Z, Auvinen A, Breckenkamp J, d’Inzeo G, Elliott P, Frei P, Heinrich S et al: Systematic review of wireless phone use and brain cancer and other head tumors. Bioelectromagnetics 2012, 33(3):187-206. doi.10.1002/bem.20716.
47. Porritt K, Gomersall J, Lockwood C: JBI’s Systematic Reviews: Study selection and critical appraisal. Am J Nurs 2014, 114(6):47-52. doi.10.1097/01.Naj.0000450430.97383.64
48. Zhu R-Z, Chen M-Q, Zhang Z-W, Wu T-Y, Zhao W-H: Dietary fatty acids and risk for Alzheimer’s disease, dementia, and mild cognitive impairment: A prospective cohort meta-analysis. Nutrition (Burbank, Los Angeles County, Calif) 2021, 90:111355. doi.10.1016/j.nut.2021.111355.
49. Shi L, Chen S-J, Ma M-Y, Bao Y-P, Han Y, Wang Y-M, Shi J, Vitiello MV, Lu L: Sleep disturbances increase the risk of dementia: A systematic review and meta-analysis. Sleep medicine reviews 2018, 40:4-16. doi.10.1016/j.smrv.2017.06.010.
50. Lv W, Du N, Liu Y, Fan X, Wang Y, Jia X, Hou X, Wang B: Low Testosterone Level and Risk of Alzheimer’s Disease in the Elderly Men: a Systematic Review and Meta-Analysis. Mol Neurobiol 2016, 53(4):2679-2684. doi.10.1007/s12035-015-9315-y.
51. Gudala K, Bansal D, Schifano F, Bhansali A: Diabetes mellitus and risk of dementia: A meta-analysis of prospective observational studies. J Diabetes Investig 2013, 4(6):640-650. doi.10.1111/jdi.12087.
52. Tsai HR, Lo RY, Liang KH, Chen TL, Huang HK, Wang JH, Lee YC: Risk of Subsequent Dementia or Alzheimer Disease Among Patients With Age-Related Macular Degeneration: A Systematic Review and Meta-analysis. Am J Ophthalmol 2023, 247:161-169. doi.10.1016/j.ajo.2022.11.005.
53. Xiong Z, Li X, Yang D, Xiong C, Xu Q, Zhou Q: The association between cataract and incidence of cognitive impairment in older adults: A systematic review and meta-analysis. Behav Brain Res 2023, 450:114455. doi.10.1016/j.bbr.2023.114455.
54. Fan L, Xu W, Cai Y, Hu Y, Wu C: Sleep Duration and the Risk of Dementia: A Systematic Review and Meta-analysis of Prospective Cohort Studies. J Am Med Dir Assoc 2019, 20(12):1480-1487.e1485. doi.10.1016/j.jamda.2019.06.009.
55. Shen L, Ji H-F: Associations between Homocysteine, Folic Acid, Vitamin B12 and Alzheimer’s Disease: Insights from Meta-Analyses. Journal of Alzheimer’s disease : JAD 2015, 46(3):777-790. doi.10.3233/JAD-150140.
56. Du N, Xu D, Hou X, Song X, Liu C, Chen Y, Wang Y, Li X: Inverse Association Between Serum Uric Acid Levels and Alzheimer’s Disease Risk. Mol Neurobiol 2016, 53(4):2594-2599. doi.10.1007/s12035-015-9271-6.
57. Policicchio S, Ahmad AN, Powell JF, Proitsi P: Rheumatoid arthritis and risk for Alzheimer’s disease: a systematic review and meta-analysis and a Mendelian Randomization study. Sci Rep 2017, 7(1):12861. doi.10.1038/s41598-017-13168-8.
58. Mehta K, Thandavan SP, Mohebbi M, Pasco JA, Williams LJ, Walder K, Ng BL, Gupta VB: Depression and bone loss as risk factors for cognitive decline: A systematic review and meta-analysis. Ageing Res Rev 2022, 76:101575. doi.10.1016/j.arr.2022.101575.
59. Shi X, Xie B, Xing Y, Tang Y: Plasma Clusterin as a Potential Biomarker for Alzheimer’s Disease-A Systematic Review and Meta-analysis. Curr Alzheimer Res 2019, 16(11):1018-1027. doi.10.2174/1567205016666191024141757.
60. Yu J-T, Xu W, Tan C-C, Andrieu S, Suckling J, Evangelou E, Pan A, Zhang C, Jia J, Feng L et al: Evidence-based prevention of Alzheimer’s disease: systematic review and meta-analysis of 243 observational prospective studies and 153 randomised controlled trials. Journal of neurology, neurosurgery, and psychiatry 2020, 91(11):1201-1209. doi.10.1136/jnnp-2019-321913.
61. Ou Y-N, Tan C-C, Shen X-N, Xu W, Hou X-H, Dong Q, Tan L, Yu J-T: Blood Pressure and Risks of Cognitive Impairment and Dementia: A Systematic Review and Meta-Analysis of 209 Prospective Studies. Hypertension (Dallas, Tex : 1979) 2020, 76(1):217-225. doi.10.1161/HYPERTENSIONAHA.120.14993.
62. Yan D, Zhang Y, Liu L, Yan H: Pesticide exposure and risk of Alzheimer’s disease: a systematic review and meta-analysis. Sci Rep 2016, 6:32222. doi.10.1038/srep32222.
63. Ou Y-N, Zhu J-X, Hou X-H, Shen X-N, Xu W, Dong Q, Tan L, Yu J-T: Associations of Infectious Agents with Alzheimer’s Disease: A Systematic Review and Meta-Analysis. Journal of Alzheimer’s disease : JAD 2020, 75(1):299-309. doi.10.3233/JAD-191337.
64. Bae SC, Lee YH: Causal association between rheumatoid arthritis and a decreased risk of Alzheimer’s disease : A Mendelian randomization study. Z Rheumatol 2019, 78(4):359-364. doi.10.1007/s00393-018-0504-8.
65. Reddy PH, Manczak M, Zhao W, Nakamura K, Bebbington C, Yarranton G, Mao P: Granulocyte-macrophage colony-stimulating factor antibody suppresses microglial activity: implications for anti-inflammatory effects in Alzheimer’s disease and multiple sclerosis. J Neurochem 2009, 111(6):1514-1528. doi.10.1111/j.1471-4159.2009.06432.x.
66. Boyd TD, Bennett SP, Mori T, Governatori N, Runfeldt M, Norden M, Padmanabhan J, Neame P, Wefes I, Sanchez-Ramos J et al: GM-CSF upregulated in rheumatoid arthritis reverses cognitive impairment and amyloidosis in Alzheimer mice. J Alzheimers Dis 2010, 21(2):507-518. doi.10.3233/jad-2010-091471.
67. Trzeciak P, Herbet M, Dudka J: Common Factors of Alzheimer’s Disease and Rheumatoid Arthritis-Pathomechanism and Treatment. Molecules 2021, 26(19). doi.10.3390/molecules26196038.
68. Dickson DW, Sinicropi S, Yen SH, Ko LW, Mattiace LA, Bucala R, Vlassara H: Glycation and microglial reaction in lesions of Alzheimer’s disease. Neurobiol Aging 1996, 17(5):733-743. doi.10.1016/0197-4580(96)00116-9.
69. Burillo J, Marqués P, Jiménez B, González-Blanco C, Benito M, Guillén C: Insulin Resistance and Diabetes Mellitus in Alzheimer’s Disease. Cells 2021, 10(5). doi.10.3390/cells10051236.
70. Biessels GJ, Staekenborg S, Brunner E, Brayne C, Scheltens P: Risk of dementia in diabetes mellitus: a systematic review. Lancet Neurol 2006, 5(1):64-74. doi.10.1016/s1474-4422(05)70284-2.
71. Craft S: Insulin resistance syndrome and Alzheimer’s disease: age- and obesity-related effects on memory, amyloid, and inflammation. Neurobiol Aging 2005, 26 Suppl 1:65-69. doi.10.1016/j.neurobiolaging.2005.08.021.
72. Michailidis M, Moraitou D, Tata DA, Kalinderi K, Papamitsou T, Papaliagkas V: Alzheimer’s Disease as Type 3 Diabetes: Common Pathophysiological Mechanisms between Alzheimer’s Disease and Type 2 Diabetes. Int J Mol Sci 2022, 23(5). doi.10.3390/ijms23052687.
73. Grizzanti J, Corrigan R, Servizi S, Casadesus G: Amylin Signaling in Diabetes and Alzheimer’s Disease: Therapy or Pathology? J Neurol Neuromedicine 2019, 4(1):12-16. doi.10.29245/2572.942x/2019/1.1212.
74. Chen H, Liu S, Ji L, Wu T, Ji Y, Zhou Y, Zheng M, Zhang M, Xu W, Huang G: Folic Acid Supplementation Mitigates Alzheimer’s Disease by Reducing Inflammation: A Randomized Controlled Trial. Mediators Inflamm 2016, 2016:5912146. doi.10.1155/2016/5912146.
75. Chen TF, Huang RF, Lin SE, Lu JF, Tang MC, Chiu MJ: Folic Acid potentiates the effect of memantine on spatial learning and neuronal protection in an Alzheimer’s disease transgenic model. J Alzheimers Dis 2010, 20(2):607-615. doi.10.3233/jad-2010-1396.
76. Langmeier M, Folbergrová J, Haugvicová R, Pokorný J, Mares P: Neuronal cell death in hippocampus induced by homocysteic acid in immature rats. Epilepsia 2003, 44(3):299-304. doi.10.1046/j.1528-1157.2003.31702.x.
77. Sharma GS, Kumar T, Dar TA, Singh LR: Protein N-homocysteinylation: From cellular toxicity to neurodegeneration. Biochim Biophys Acta 2015, 1850(11):2239-2245. doi.10.1016/j.bbagen.2015.08.013.
78. Jakubowski H, Głowacki R: Chemical biology of homocysteine thiolactone and related metabolites. Adv Clin Chem 2011, 55:81-103. doi.10.1016/b978-0-12-387042-1.00005-8.
79. Li JG, Chu J, Barrero C, Merali S, Praticò D: Homocysteine exacerbates β-amyloid pathology, tau pathology, and cognitive deficit in a mouse model of Alzheimer disease with plaques and tangles. Ann Neurol 2014, 75(6):851-863. doi.10.1002/ana.24145.
80. Tagai K, Nagata T, Shinagawa S, Nemoto K, Inamura K, Tsuno N, Nakayama K: Correlation between both morphologic and functional changes and anxiety in Alzheimer’s disease. Dement Geriatr Cogn Disord 2014, 38(3-4):153-160. doi.10.1159/000358822.
81. Tsang SW, Vinters HV, Cummings JL, Wong PT, Chen CP, Lai MK: Alterations in NMDA receptor subunit densities and ligand binding to glycine recognition sites are associated with chronic anxiety in Alzheimer’s disease. Neurobiol Aging 2008, 29(10):1524-1532. doi.10.1016/j.neurobiolaging.2007.03.014.
82. Liao Y, Xing Q, Li Q, Zhang J, Pan R, Yuan Z: Astrocytes in depression and Alzheimer’s disease. Front Med 2021, 15(6):829-841. doi.10.1007/s11684-021-0875-0.
83. Herbert J, Lucassen PJ: Depression as a risk factor for Alzheimer’s disease: Genes, steroids, cytokines and neurogenesis – What do we need to know? Front Neuroendocrinol 2016, 41:153-171. doi.10.1016/j.yfrne.2015.12.001.
84. Ganguli M: Depression, cognitive impairment and dementia: Why should clinicians care about the web of causation? Indian J Psychiatry 2009, 51 Suppl 1(Suppl1):S29-34. https://pubmed.ncbi.nlm.nih.gov/21416013
85. Koch G, Di Lorenzo F, Bonnì S, Giacobbe V, Bozzali M, Caltagirone C, Martorana A: Dopaminergic modulation of cortical plasticity in Alzheimer’s disease patients. Neuropsychopharmacology 2014, 39(11):2654-2661. doi.10.1038/npp.2014.119.
86. Bellou V, Belbasis L, Tzoulaki I, Evangelou E, Ioannidis JP: Environmental risk factors and Parkinson’s disease: An umbrella review of meta-analyses. Parkinsonism Relat Disord 2016, 23:1-9. doi.10.1016/j.parkreldis.2015.12.008.
87. Bellou V, Belbasis L, Tzoulaki I, Middleton LT, Ioannidis JPA, Evangelou E: Systematic evaluation of the associations between environmental risk factors and dementia: An umbrella review of systematic reviews and meta-analyses. Alzheimers Dement 2017, 13(4):406-418. doi.10.1016/j.jalz.2016.07.152.
88. Zhong G, Wang Y, Zhang Y, Guo JJ, Zhao Y: Smoking is associated with an increased risk of dementia: a meta-analysis of prospective cohort studies with investigation of potential effect modifiers. PLoS One 2015, 10(3):e0118333. doi.10.1371/journal.pone.0118333.
89. Su S, Shi L, Zheng Y, Sun Y, Huang X, Zhang A, Que J, Sun X, Shi J, Bao Y et al: Leisure Activities and the Risk of Dementia: A Systematic Review and Meta-Analysis. Neurology 2022, 99(15):e1651-1663. doi.10.1212/wnl.0000000000200929.
90. Kim Y-S, Kwak SM, Myung S-K: Caffeine intake from coffee or tea and cognitive disorders: a meta-analysis of observational studies. Neuroepidemiology 2015, 44(1):51-63. doi.10.1159/000371710.
91. Qiao L, Wang G, Tang Z, Zhou S, Min J, Yin M, Li M: Association between loneliness and dementia risk: A systematic review and meta-analysis of cohort studies. Front Hum Neurosci 2022, 16:899814. doi.10.3389/fnhum.2022.899814.
92. Zhao R, Han X, Zhang H, Liu J, Zhang M, Zhao W, Jiang S, Li R, Cai H, You H: Association of vitamin E intake in diet and supplements with risk of dementia: A meta-analysis. Front Aging Neurosci 2022, 14:955878. doi.10.3389/fnagi.2022.955878.
93. Zhao R, Han X, Jiang S, Zhao W, Liu J, Zhang H, Mao X, Zhang M, Lei L, You H: Association of Dietary and Supplement Intake of Antioxidants with Risk of Dementia: A Meta-Analysis of Cohort Studies. J Alzheimers Dis 2023. doi.10.3233/jad-220909.
94. Zuin M, Roncon L, Passaro A, Bosi C, Cervellati C, Zuliani G: Risk of dementia in patients with atrial fibrillation: Short versus long follow-up. A systematic review and meta-analysis. Int J Geriatr Psychiatry 2021, 36(10):1488-1500. doi.10.1002/gps.5582.
95. Xie B, Shi X, Xing Y, Tang Y: Association between atherosclerosis and Alzheimer’s disease: A systematic review and meta-analysis. Brain Behav 2020, 10(4):e01601. doi.10.1002/brb3.1601.
96. Qu H, Yang S, Yao Z, Sun X, Chen H: Association of Headache Disorders and the Risk of Dementia: Meta-Analysis of Cohort Studies. Front Aging Neurosci 2022, 14:804341. doi.10.3389/fnagi.2022.804341.
97. Liang Z, Li A, Xu Y, Qian X, Gao X: Hearing Loss and Dementia: A Meta-Analysis of Prospective Cohort Studies. Front Aging Neurosci 2021, 13:695117. doi.10.3389/fnagi.2021.695117.
98. Li Y, Li Y, Li X, Zhang S, Zhao J, Zhu X, Tian G: Head Injury as a Risk Factor for Dementia and Alzheimer’s Disease: A Systematic Review and Meta-Analysis of 32 Observational Studies. PLoS One 2017, 12(1):e0169650. doi.10.1371/journal.pone.0169650.
99. Dun C, Zhang Y, Yin J, Su B, Peng X, Liu L: Bi-directional associations of epilepsy with dementia and Alzheimer’s disease: a systematic review and meta-analysis of longitudinal studies. Age Ageing 2022, 51(3). doi.10.1093/ageing/afac010.
100. Zhang D-D, Ou Y-N, Fu Y, Wang Z-B, Huang L-Y, Tan L, Yu J-T: Risk of Dementia in Cancer Survivors: A Meta-Analysis of Population-Based Cohort Studies. Journal of Alzheimer’s disease : JAD 2022, 89(1):367-380. doi.10.3233/JAD-220436.
101. Kojima G, Taniguchi Y, Iliffe S, Walters K: Frailty as a Predictor of Alzheimer Disease, Vascular Dementia, and All Dementia Among Community-Dwelling Older People: A Systematic Review and Meta-Analysis. J Am Med Dir Assoc 2016, 17(10):881-888. doi.10.1016/j.jamda.2016.05.013.
102. Li L, Zhang Q, Yang D, Yang S, Zhao Y, Jiang M, Wang X, Zhao L, Liu Q, Lu Z et al: Tooth loss and the risk of cognitive decline and dementia: A meta-analysis of cohort studies. Front Neurol 2023, 14:1103052. doi.10.3389/fneur.2023.1103052.
103. Wang Z, Wei X, Yang J, Suo J, Chen J, Liu X, Zhao X: Chronic exposure to aluminum and risk of Alzheimer’s disease: A meta-analysis. Neurosci Lett 2016, 610:200-206. doi.10.1016/j.neulet.2015.11.014.
104. Wang AY, Hu HY, Ou YN, Wang ZT, Ma YH, Tan L, Yu JT: Socioeconomic Status and Risks of Cognitive Impairment and Dementia: A Systematic Review and Meta-Analysis of 39 Prospective Studies. Journal of Prevention of Alzheimer’s Disease 2022. doi.10.14283/jpad.2022.81.
105. Tong JH, Gong SQ, Zhang YS, Dong JR, Zhong X, Wei MJ, Liu MY: Association of Circulating Apolipoprotein AI Levels in Patients With Alzheimer’s Disease: A Systematic Review and Meta-Analysis. Front Aging Neurosci 2022, 14:899175. doi.10.3389/fnagi.2022.899175.
106. Tang Q, Wang F, Yang J, Peng H, Li Y, Li B, Wang S: Revealing a Novel Landscape of the Association Between Blood Lipid Levels and Alzheimer’s Disease: A Meta-Analysis of a Case-Control Study. Front Aging Neurosci 2019, 11:370. doi.10.3389/fnagi.2019.00370.
107. Lv XL, Zhang J, Gao WY, Xing WM, Yang ZX, Yue YX, Wang YZ, Wang GF: Association between Osteoporosis, Bone Mineral Density Levels and Alzheimer’s Disease: A Systematic Review and Meta-analysis. International Journal of Gerontology 2018, 12(2):76-83. doi.10.1016/j.ijge.2018.03.007.
108. Li H, Zhao S, Wang R, Gao B: The association between cognitive impairment/dementia and albuminuria: a systematic review and meta-analysis. Clin Exp Nephrol 2022, 26(1):45-53. doi.10.1007/s10157-021-02127-3.
109. Kim BY, Lee SH, Graham PL, Angelucci F, Lucia A, Pareja-Galeano H, Leyhe T, Turana Y, Lee IR, Yoon JH et al: Peripheral Brain-Derived Neurotrophic Factor Levels in Alzheimer’s Disease and Mild Cognitive Impairment: a Comprehensive Systematic Review and Meta-analysis. Mol Neurobiol 2017, 54(9):7297-7311. doi.10.1007/s12035-016-0192-9.
110. Poly TN, Islam MM, Walther BA, Yang H-C, Wu C-C, Lin M-C, Li Y-C: Association between Use of Statin and Risk of Dementia: A Meta-Analysis of Observational Studies. Neuroepidemiology 2020, 54(3):214-226. doi.10.1159/000503105.
111. Wang J, Tan L, Wang H-F, Tan C-C, Meng X-F, Wang C, Tang S-W, Yu J-T: Anti-inflammatory drugs and risk of Alzheimer’s disease: an updated systematic review and meta-analysis. Journal of Alzheimer’s disease : JAD 2015, 44(2):385-396. doi.10.3233/JAD-141506.
© The Authors 2024