S. Censi1,2, C. Sestieri1,2, M. Punzi1, A. Delli Pizzi2,3, A. Ferretti1,2,4, F. Gambi1, V. Tomassini1,2,5, S. Delli Pizzi1,6, S.L. Sensi1,2,5,6 for the Alzheimer’s Disease Neuroimaging Initiative*
1. Department of Neuroscience, Imaging, and Clinical Sciences, University «G. d’Annunzio» of Chieti-Pescara, Italy; 2. Institute for Advanced Biomedical Technologies (ITAB), “G. d’Annunzio” University, Chieti-Pescara, Italy; 3. Department of Innovative Technologies in Medicine and Dentistry, «G. d’Annunzio» University of Chieti-Pescara, Chieti, Italy; 4. UdA-TechLab, Research Center, University “G. d’Annunzio” of Chieti-Pescara, 66100 Chieti, Italy; 5. MS Centre, Institute of Neurology, SS Annunziata University Hospital, Chieti, Italy; 6. Molecular Neurology Unit, Center for Advanced Studies and Technology (CAST), University «G. d’Annunzio» of Chieti-Pescara, Italy.
Corresponding Author: Stefano Delli Pizzi, Department of Neuroscience, Imaging, and Clinical Sciences, University «G. d’Annunzio» of Chieti-Pescara, Via Polacchi, 11 Chieti 66100, Italy, stefano.dellipizzi@unich.it; Stefano L Sensi, Department of Neuroscience, Imaging, and Clinical Sciences, University «G. d’Annunzio» of Chieti-Pescara, Via Polacchi, 11 Chieti 66100, Italy, stefano.sensi@unich.it
J Prev Alz Dis 2024;4(11):1030-1040
Published online February 21, 2024, http://dx.doi.org/10.14283/jpad.2024.42
Abstract
BACKGROUND: Patients with Alzheimer’s Disease (AD) exhibit structural alterations of the thalamus that correlate with clinical symptoms. However, given the anatomical complexity of this brain structure, it is still unclear whether atrophy affects specific thalamic nuclei and modulates the clinical progression from a prodromal stage, known as Mild Cognitive Impairment (MCI), to full-fledged AD.
OBJECTIVES: To characterize the structural integrity of distinct thalamic nuclei across the AD spectrum, testing whether MCI patients who convert to AD (c-MCI) show a distinctive pattern of thalamic structural alterations compared to patients who remain stable (s-MCI).
DESIGN: Investigating between-group differences in the volumetric features of distinct thalamic nuclei across the AD spectrum.
SETTING: Prodromal and clinical stages of AD.
PARTICIPANTS: We analyzed data from 84 healthy control subjects (HC), 58 individuals with MCI, and 102 AD patients. The dataset was obtained from the AD Neuroimaging Initiative (ADNI-3) database. The MCI group was further divided into two subgroups depending on whether patients remained stable (s-MCI, n=22) or progressed to AD (s-MCI, n=36) in the 48 months following the diagnosis.
MEASUREMENTS: A multivariate analysis of variance (MANOVA) assessed group differences in the volumetric features of distinct thalamic nuclei obtained from magnetic resonance (MR) images. A stepwise discriminant function analysis identified which feature most effectively predicted the conversion to AD. The corresponding predictive performance was evaluated through a Receiver Operating Characteristic approach.
RESULTS: AD and c-MCI patients showed generalized atrophy of thalamic nuclei compared to HC. In contrast, no significant structural differences were observed between s-MCI and HC subjects. Compared to s-MCI, c-MCI individuals displayed significant atrophy of the nucleus reuniens and a trend toward significant atrophy in the anteroventral and laterodorsal nuclei. The discriminant function analysis confirmed the nucleus reuniens as a significant predictor of AD conversion, with a sensitivity of 0.73 and a specificity of 0.69.
CONCLUSIONS: In line with the pathophysiological relevance of the nucleus reuniens proposed by seminal post-mortem studies on patients with AD, we confirm the pivotal role of this nucleus as a critical hub in the clinical progression to AD. We also propose a theoretical model to explain the evolving dysfunction of subcortical brain networks in the disease process.
Key words: Alzheimer’s Disease (AD), Mild Cognitive Impairment (MCI), thalamus, reuniens, Magnetic Resonance Imaging (MRI).
Introduction
Alzheimer’s disease (AD) is one of the most common age-related neurodegenerative diseases, with a growing incidence worldwide. The clinical manifestations usually initiate with a prodromal state known as Mild Cognitive Impairment (MCI), which may eventually progress to subsequent AD in a fraction of subjects (10-15%). Since the neurodegenerative process leading to AD begins more than a decade before a clinical diagnosis can be made (1), the detection of early signs of the future conversion from MCI to AD is of great clinical importance for the decision to initiate both pharmacological and non-pharmacological interventions. Magnetic Resonance Imaging (MRI) is a non-invasive tool for exploring macrostructural changes across the AD spectrum (2–4). In particular, atrophy and metabolic alterations in the hippocampal and entorhinal regions have emerged as potential clinical markers of the progression to AD (5–7). This emphasis is coherent with the pivotal role of the hippocampus in episodic memory and navigation (8), two cognitive domains that are significantly impacted in early AD. However, MRI research also demonstrates the involvement of other cortical and subcortical networks, like the Papez circuit, in the neurodegenerative process leading to AD (9).
Growing lines of evidence indicate that patients with AD also exhibit significant structural alterations of the thalamus (10–13), pathological features that correlates with clinical symptoms (9, 14). However, previous studies treated the thalamus as a whole, potentially neglecting its constituent nuclei’s anatomical and functional specificity. Anatomically, the thalamus is a heterogeneous structure comprising several nuclei, each bidirectionally connected to distinct subcortical and cortical regions (15). Post-mortem AD studies revealed a hierarchical pathological evolution within thalamic nuclei, indicating abnormal accumulation of tau protein, especially in nuclei associated with the limbic system, such as the anterior and lateral nuclei (16). While the AD model based on amyloid deposition is highly debated (17, 18), tau-driven pathological processes are considered more fit to indicate the regional progression of the disease and its impact on symptoms. In addition, the classical model of tau pathology spreading in AD, developed by Braak and Braak (19, 20), has highlighted the possible pathophysiological relevance of the nucleus reuniens. This structure exhibits an interesting pattern of anatomical connections with cortical and subcortical regions typically affected by AD, including the hippocampal formation and the medial prefrontal cortex (21).
Despite these seminal post-mortem observations, the spatial pattern of structural integrity of the thalamus across the AD spectrum remains largely unexplored. One reason for this knowledge gap is that the structure’s composition complicates the structural analysis of the thalamus via MRI, as the individual nuclei cannot be easily separated using standard analysis methods of anatomical images. To overcome these limitations, we combined probabilistic techniques and a priori information derived from ex-vivo MRI and histology to analyze MR raw images obtained from the AD Neuroimaging Initiative (ADNI) platform and perform an accurate parcellation of thalamic nuclei (22). This innovative approach has demonstrated test-retest solid reliability and robustness when applied to data involving elderly healthy subjects and/or AD patients (23). We characterized the structural integrity of distinct thalamic nuclei across the AD spectrum and tested whether MCI patients who convert to AD (c-MCI) exhibit a distinctive pattern of thalamic structural alterations compared to patients who remain stable (s-MCI).
Material and Methods
Study Data, Inclusion, and Diagnostic Criteria
All data for this article were obtained from the ADNI-3 database, an ongoing large-scale, multi-center study across the U.S. and Canada (adni.loni.usc.edu). ADNI-3 was launched in late 2016 as a public/private partnership to identify brain imaging [MRI, Positron Emission Tomography (PET)] and clinical, cognitive, and molecular biomarkers of AD and aging. For updated information on the initiative, see www.adniinfo.org. The study was performed according to ethical standards and the Declaration of Helsinki (1997). Informed consent was obtained from study participants or legally authorized representatives. Details on the study protocol are reported on the ADNI website (http://www.adni-info.org).
A total of 244 subjects were included in this study, divided into 84 HC, 58 patients with MCI, and 102 patients with probable AD. The HC group included cognitively normal individuals from baseline to follow-up 48 months. MCI subjects were further divided based on clinical follow-up into 35 subjects who converted to AD within 48 months (c-MCI) and 22 subjects who did not convert to AD within 48 months (s-MCI). The Detailed demographic characteristics are summarized in Table 1. The age range was between 55 and 95 years.
T1-weighted images were acquired by a 3T scanner using a harmonized protocol and identical acquisition parameters to minimize site differences (https://adni.loni.usc.edu/methods/mri-tool/mri-analysis/). In our study, we included only those subjects who completed baseline 3D T1-weighted scans and neuropsychological/clinical investigations. Subjects who underwent MRI but had incomplete clinical and demographic information and those whose MRI scan had technical issues (severe motion, missing volumes, or corrupted files) were excluded from the study sample.
Clinical assessments
At the time of the MRI scan, all participants were extensively assessed for cognitive functions with the Mini Mental State Examination (MMSE) (24) and the Montreal Cognitive Assessment (MoCA) (25) to evaluate global cognition; Functional Activities Questionnaire (FAQ) for the investigation of daily living activities (26); the Cognitive subscales of the Alzheimer’s Disease Assessment Scale (ADAS-11 items scores; ADAS-13 items scores; ADAS-Q4 delayed word recall subscale) to study the severity of impairments of memory, learning, language, praxis, and orientation (27, 28); the Clock Drawing Test (CT-Drawing) to assess dysfunction of visuoconstructive abilities (29); the Animal Fluency [30] and Multilingual Naming Test (MINT) to detect language capacities and naming deficits (31); the Trail Making Test (time to completion, TMT parts A and B) for processing speed and executive function (32); the Rey Auditory Verbal Learning Test (RAVLT) to investigate auditory verbal learning and memory (immediate memory span, learning, delayed recall, and delayed recognition) and the Logical Memory II subscale (LM) of the Wechsler Memory Scales Revised (WMS-R) Story A to assess immediate and delayed recall (33, 34); the Neuropsychiatric Inventory (NPI) and the Geriatric Depression Scale (GDS) to obtain information on psychopathological and behavioral features (35, 36).
The HC subjects exhibited no significant impairment in cognitive functions or activities of daily living. Their MMSE scores fell within the range of 27 to 30. They achieved a global score of 0 on the Clinical Dementia Rating Scale (CDR-RS) (37), with a specific score of 0 in the Memory Box category, indicating a lack of dementia. Moreover, their memory function was assessed using the LM, and they scored above the education-adjusted cut-offs (9 for 16 or more years of education, 5 for 8-15 years of education, 3 for 0-7 years of schooling), confirming normal memory performance.
For subjects with MCI, the inclusion criteria involved MMSE scores between 24 and 30, subjective memory concerns, and memory impairments identified by their partners. Moreover, the CDR Memory Box score for MCI subjects was required to be at least 0.5, indicating a mild cognitive decline. Memory function was evaluated using the LM, and MCI subjects scored below education-adjusted cut-offs (< 11 for 16 or more years of education; ≤ 9 for 8-15 years of schooling; ≤ 6 for 0-7 years of education), confirming abnormal memory performance. However, their general cognitive status and functional abilities were preserved enough to exclude a diagnosis of AD.
Subjects of the AD group met the criteria for probable AD as set by the National Institute of Neurologic and Communicative Disorders and Stroke, as well as the Alzheimer’s Disease and Related Disorders Association.
For more details about the ADNI-3 inclusion criteria, see https://adni.loni.usc.edu/wp-content/themes/freshnews-dev-v2/documents/clinical/ADNI-3_Protocol.pdf.
The clinical determination of the conversion from MCI to dementia was made by experienced ADNI clinicians. This determination relied on a comprehensive evaluation, including information from the patient and a well-informed caregiver. Additionally, biological markers and neuropsychological assessments were considered to support the diagnosis.
MRI data acquisition and analysis
The imaging protocol included a 3T T1-weighted sagittal 3D MPRAGE volume (voxel size 1.05×1.05×1.2 mm). A detailed ADNI data acquisition protocol description can be found at https://adni.loni.usc.edu/methods/documents/mriprotocols/. T1-weighted images were processed with FSL-FIRST’s pipeline (https://fsl.fmrib.ox.ac.uk/fsl/fslwiki/FIRST) to obtain an accurate volumetry of the entire thalamus (38). T1-weighted images were processed with FreeSurfer 7.3 using the «recon-all -all» command line. Briefly, this processing includes motion correction and intensity normalization of T1-weighted images, removal of non-brain tissue using a hybrid watershed/surface deformation procedure, automated Talairach transformation, segmentation of the subcortical white matter and deep gray matter volumetric structures (including the hippocampus, amygdala, caudate, putamen, ventricles), tessellation of the gray matter white matter boundary, and derivation of cortical thickness. The tool provided an automated reconstruction and labeling of cortical and subcortical regions and a measure of estimated Intracranial Volume (eTIV). Based on the methods developed by Iglesias and colleagues (2018), the thalamus of each subject was parceled in twenty-five nuclei for each hemisphere (Figure 1). Specifically, the anterior group included the anterior (AV), the laterodorsal (LD), and lateroposterior (LP) nuclei; the medial groups consisted of the paratenial (Pt), medial ventral reuniens (MV-re), magnocellular medial mediodorsal (MDm) and parvocellular lateral mediodorsal (MDl) nuclei; the pulvinar regions included the anterior (PuA), inferior (PuI), lateral (PuL), medial (PuM) nuclei; the metathalamus encompassed the medial (MGN) and lateral (LGN) geniculate nucleus, the nucleus limitans (SG); the ventral portion consisted of the ventral-anterior (VA) nucleus, ventrolateral anterior (VLa) and posterior (VLp) regions, and the ventral-postero-lateral and ventromedial nuclei; the non-specific nuclei included the central medial (CeM), central lateral (CL), paracentral (Pc), centromedian (CM), and parafascicular (Pf) nuclei. We thoroughly examined all the segmentations of thalamic nuclei and found no issues. It is noteworthy that no additional manual corrections were made, as the anatomical boundaries consistently matched the atlas and remained uniform across subjects, a result confirmed by meticulous visual inspection.
The images are presented in the neuroradiological convention.
Statistical analysis
Analysis of variance and Turkey’s post hoc tests were used to evaluate the group differences regarding demographic, neuropsychological, and clinical data. Categorical variables were analyzed using chi-square tests. For the MRI measures, a multivariate analysis of variance (MANOVA) with 4 levels [HC, s-MCI, c-MCI, AD], followed by Turkey’s post-hoc comparison, was applied to test the differences among groups. The MRI volumes of the thalamic nuclei and the whole thalamus were additionally included in a stepwise discriminant function analysis to determine whether a set of variables effectively predicted category membership (s-MCI or c-MCI). Wilk’s lambda tests how well each independent variable level contributes to the model. Each independent variable is tested by putting it into the model and then taking it out — generating a Wilks’ lambda statistic. The significance of changes in Wilks’ lambda is measured with an F-test; if the F-value is greater than the critical value, the variable is kept in the model. Finally, a Receiver Operating Characteristic (ROC) analysis of the predictive function was used to determine a relative sensitivity and specificity cut-off to investigate whether the baseline volumes of specific subfields could discriminate MCI subjects that progress to AD. ROC analysis allows for the comprehensive evaluation of classification performance by assessing the trade-off between true positive rates (sensitivity) and false positive rates (1-specificity). This provides a robust measure of diagnostic accuracy, particularly in situations where the optimal classification threshold may vary. All statistical tests were two-tailed, and the significant p-value threshold was set at 0.05.
Results
Demographic, clinical, and cognitive features of the study groups
Summary statistics on demographics are shown in Table 1 and Supplementary Tables 1-2. No significant difference across study groups (HC, s-MCI, c-MCI, and AD) was found on age, educational level, and sex.
Values are expressed as the mean ± standard deviation (SD). Bold values are statistically significant comparisons. Abbreviations: AD=Alzheimer’s disease; ADAS=Alzheimer’s Disease Assessment Scale (11 items and 13 items versions); ADAS-Q4=ADAS delayed word recall subscale; AF=Animal Fluency; RAVLT-TOT=Rey’s Auditory Verbal Learning Total Recognition score; CDR-RS=Clinical Dementia Rating Scale; CT-Copy=Clock Test- Copy score; CT-Drawing=Clock Test- Drawing total score; c-MCI=patients with MCI who convert to AD within 48-month follow-up; FAQ=Functional Activities Questionnaire; GDS=Geriatric Depression Scale; HC=healthy control stable after 48 months of follow-up; LM-IR=Logical Memory-Immediate Recall Total Number of Story Units Recalled; LM-DR=Logical Memory-Delayed Recall Total Number of Story Units Recalled; MINT-CUE=Multilingual Naming Test Total Correct – with Semantic Cue; MINT-Total=Multilingual Naming Test Total Correct (Uncued + Correct with Semantic cue); MINT-UNC =Multilingual Naming Test Total Uncued Correct; MMSE=Mini-Mental State Examination Total Score; MoCA=Montreal Cognitive Assessment; NA=Not Applicable; NPI=Neuropsychiatric Inventory Questionnaire; RAVLT-IR=Rey’s Auditory Verbal Learning Test, Immediate Recall (sum of 5 trials); RAVLT-L=Rey’s Auditory Verbal Learning Test, learning (trial 5 – trial 1); RAVLT-DR=Rey’s Auditory Verbal Learning Test, 30 minute Delayed Recall; RAVLT-RN=Rey’s Auditory Verbal Learning Test Delayed Recognition (RAVLT TOT Recognition Score – Total Intrusions); RAVLT-TOT= Rey’s Auditory Verbal Learning Test Total Recognition Score; s-MCI=MCI patients who did not convert to AD after 48-month follow-up; TMT=Trail Making Test (parts A and B); t-Tau=CSF Total Tau concentration (pg/mL).
The baseline behavioral analysis (Table 1; Supplementary Tables 1 and 2) indicated that the s-MCI and the c-MCI groups displayed differences in general cognition (i.e., ADAS-11/13, MMSE) and memory (i.e., ADASQ4, RAVLT, LM-IR, and LM-DR). No further significant difference was observed between the MCI groups for neuropsychological and neuropsychiatric variables. Compared with the HC subjects, both the c-MCI subsets and the AD group exhibited significant impairments in general cognition, memory functions, language (i.e., animal fluency), and executive functions (i.e., TMT-B). Subjects of the AD group also had higher impairment in visuospatial abilities (i.e., CT-Drawing), naming (i.e., Multilingual Naming Test), and neuropsychiatric conditions (i.e., NPI and the GDS). The s-MCI group showed general cognitive performance comparable to the HC group, although their memory performance was significantly compromised. They were characterized by severe deficits across multiple cognitive domains compared to HC and MCI subjects.
We further evaluated longitudinal variations in neuropsychological features across the HC and MCI groups (Table 2 and Supplementary Tables 3). As reported in Table 2, the c-MCI group, compared to the s-MCI group, exhibited more significant variations in global cognition measures as indicated by the ADAS-11/13, MOCA, and FAQ. Additionally, there was a significant variation in visuo-spatial abilities (i.e., Clock Test-Drawing) and neuropsychiatric symptoms (i.e., NPI total). The c-MCI, but not the s-MCI group, showed significant changes in test scores of memories (i.e., LM-IR), language/executive functions (i.e., animal fluency and TMT-B), and neuropsychiatric features (i.e., NPI total).
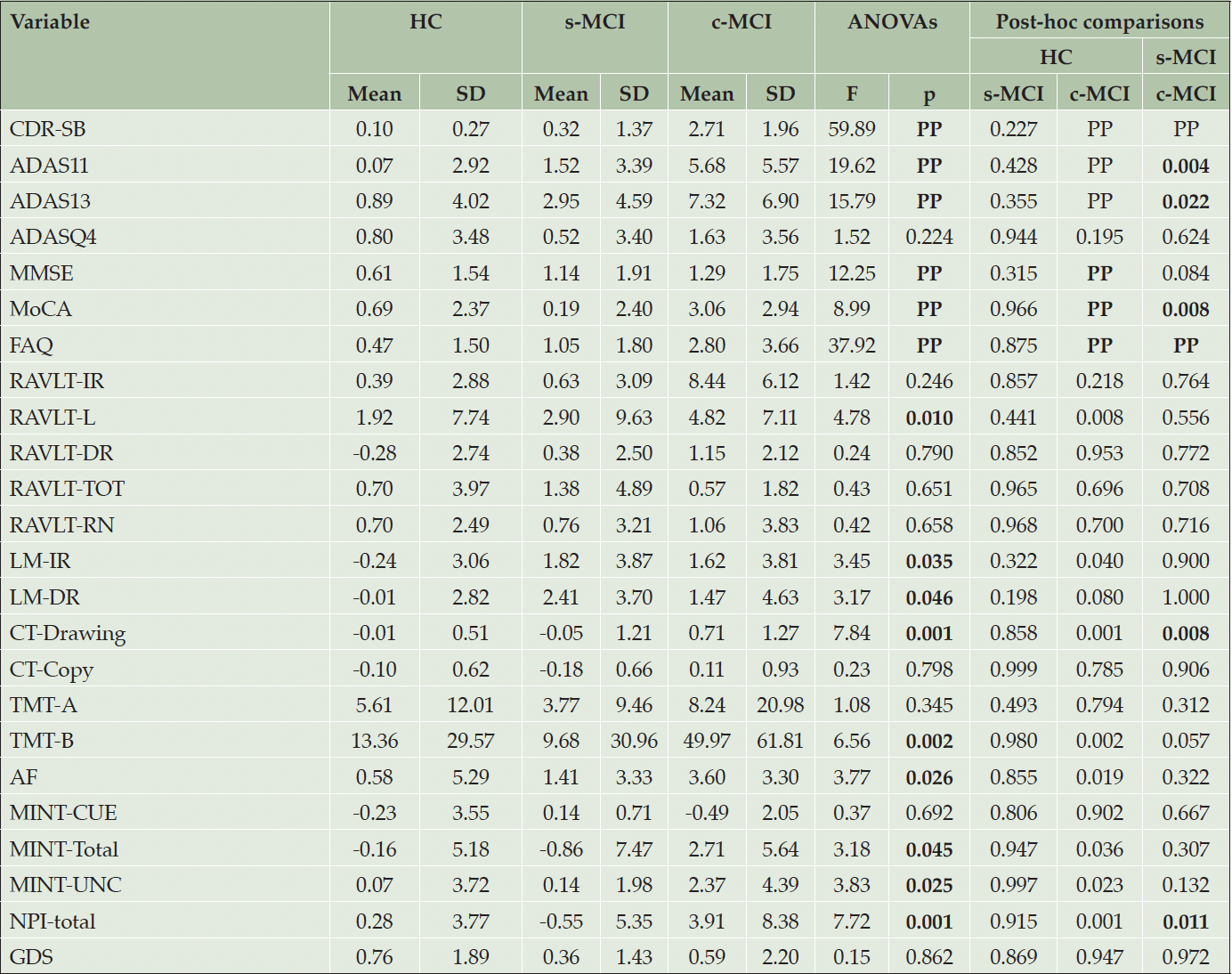
Table 2. Demographic. neuropsychological, and clinical features of HC and MCI groups after follow-up
Abbreviations: ADAS=Alzheimer’s Disease Assessment Scale (11 items and 13 items versions); ADAS-Q4=ADAS delayed word recall subscale; AF=Animal Fluency; AVDEL-TOT=Rey Auditory Verbal Learning Test (Trials 1-6); CDR-RS=Clinical Dementia Rating Scale; CT-Copy=Clock Test- Copy score; CT-Drawing=Clock Test- Drawing total score; c-MCI=patients with MCI who convert to AD within 48-month follow-up; FAQ=Functional Activities Questionnaire; GDS=Geriatric Depression Scale; HC=healthy control stable after 48 months of follow-up; LM-IR=Logical Memory-Immediate Recall Total Number of Story Units Recalled; LM-DR=Logical Memory-Delayed Recall Total Number of Story Units Recalled; MINT-CUE=Multilingual Naming Test Total Correct – with Semantic Cue; MINT-Total=Multilingual Naming Test Total Correct (Uncued + Correct with Semantic cue); MINT-UNC =Multilingual Naming Test Total Uncued Correct; MMSE=Mini-Mental State Examination Total Score; MoCA=Montreal Cognitive Assessment; NPI=Neuropsychiatric Inventory Questionnaire; RAVLT-IR=Rey’s Auditory Verbal Learning Test, Immediate Recall (sum of 5 trials); RAVLT-L=Rey’s Auditory Verbal Learning Test, learning (trial 5 – trial 1); RAVLT-DR=Rey’s Auditory Verbal Learning Test, 30 minute Delayed Recall; RAVLT-RN=Rey’s Auditory Verbal Learning Test Delayed Recognition (RAVLT TOT Recognition Score – Total Intrusions); RAVLT-TOT= Rey’s Auditory Verbal Learning Test Total Recognition Score; s-MCI=MCI patients who did not convert to AD after 48-month follow-up; TMT=Trail Making Test (parts A and B).
MRI volumetry of thalamic nuclei and whole thalamus
The main findings on MRI measures on thalamic nuclei are shown in Table 3. The results of the MANCOVA analysis revealed that, in comparison to HC subjects, both c-MCI individuals and AD patients exhibited widespread atrophy across the thalamic subfields. At the same time, the s-MCI group did not show significant differences (Supplementary Table 4). When comparing the s-MCI and c-MCI subjects, the c-MCI group showed significant atrophy in the nucleus reuniens and a trend toward significant atrophy in the AV and LD nuclei (Tables 3). The overall comparison of the entire thalamus did not yield significant differences, except when comparing AD subjects to HC subjects (Supplementary Tables 5 and 6).
Values x 10-4 are expressed as the mean ± standard deviation (SD). MANOVA’s outputs: F= 2.061, PP. Bold values are statistically significant comparisons in ANOVAs and post-hoc comparisons. Abbreviations: AD=Alzheimer’s disease; ANT=anterior group; AV=anterior; CeM=central medial; CL=central lateral; c-MCI=patients with MCI who convert to AD within 48-month follow-up; CM=centromedian; HC=healthy control stable after 48 months of follow-up; LD=laterodorsal; LP=lateroposterior (LP) nuclei; LGN=lateral geniculate nucleus; MGN=media geniculate nucleus; MDm=magnocellular medial mediodorsal; MDl= parvocellular lateral mediodorsal; MTH=metathalamus; Re=reuniens; NA=Not Applicable; NS=non-specific nuclei; Pc=paracentral; Pf=parafascicular; Pt=paratenial; PuA=anterior pulvinar; PuI=inferior pulvinar; PuL=lateral pulvinar; PuM=medial pulvinar; s-MCI=MCI patients who did not convert to AD after 48-month follow-up; SG=limitans; VA=ventral-anterior; VLa=ventrolateral anterior; VLp=posterior, VPL=ventral-postero-lateral; VM=ventromedial nuclei.
Discriminant function and ROC analyses
The discriminant function analysis showed that the right nucleus reuniens was the most significant predictor for distinguishing diagnostic groups, maximizing the separation between c-MCI and s-MCI subjects (Supplementary Table 7). The area under the curve (AUC) for discriminating c-MCI from s-MCI based on the volume of the nucleus reuniens was 0.76, with a sensitivity of 0.73 and specificity of 0.69. As expected, the AUC for the nucleus reuniens was higher than the AUCs calculated for any other thalamic nucleus or the whole thalamus (as shown in Supplementary Table 8).
Discussion
The primary aim of the present study was to detect morphometric alterations in specific thalamic nuclei in patients encompassing the AD spectrum. While AD individuals exhibited generalized atrophy of the whole thalamus, our intergroup analysis revealed a distinct morphometric pattern that characterizes the transition from MCI to AD. The analysis points to the crucial involvement of the nucleus reuniens. Additionally, a trend toward a significant difference between c-MCI and s-MCI was observed for the limbic thalamus, including the AV and LD nuclei. Consistently, the discriminant function analysis confirmed the atrophy of the nucleus reuniens as the most influential predictor for distinguishing between the c-MCI and s-MCI groups.
A broad alteration of the Papez circuit has been reported in various conditions, including AD, Amyotrophic lateral sclerosis (ALS), Korsakoff syndrome, depression, Parkinson’s disease, epilepsy, and schizophrenia (19, 39–44). From a physiological standpoint, the nucleus reuniens represents a central hub within the Papez circuit (9, 45, 46), serving as the principal source of thalamic input to the hippocampus (47). Given the absence of direct bidirectional anatomical connectivity between these two structures, this nucleus functions as a crucial glutamatergic relay, facilitating the interaction between the medial prefrontal cortex (mPFC) and the hippocampus. By receiving inputs from the mPFC, the nucleus reuniens acts as a filter, contributing to memory consolidation and spatial navigation through excitatory projections to the hippocampus and presubiculum (21) (Figure 2). This mechanism remains active during slow-wave sleep (48, 49). Thirty years ago, Braak & Braak (19, 20, 39) first recognized the importance of the nucleus reuniens in the pathophysiology of AD. Their research demonstrated that the nucleus reuniens contains virtually no amyloid plaques but shows an abundance of neurofibrillary tangles (19). This phenomenon is associated with deficits in memory consolidation and spatial navigation that typically affect subjects at the early AD stages. More recently, atrophy of the nucleus reuniens has been described in ALS and AD (14, 40). Likewise, accumulation of neurofibrillary tangles and associated neurodegeneration in the nucleus reuniens has been observed in Guam’s ALS/Parkinsonism-Dementia Complex (50). Interestingly, a recent study demonstrated how deep brain stimulation of the nucleus reuniens promotes neuronal and cognitive resilience in an AD mouse model (51).
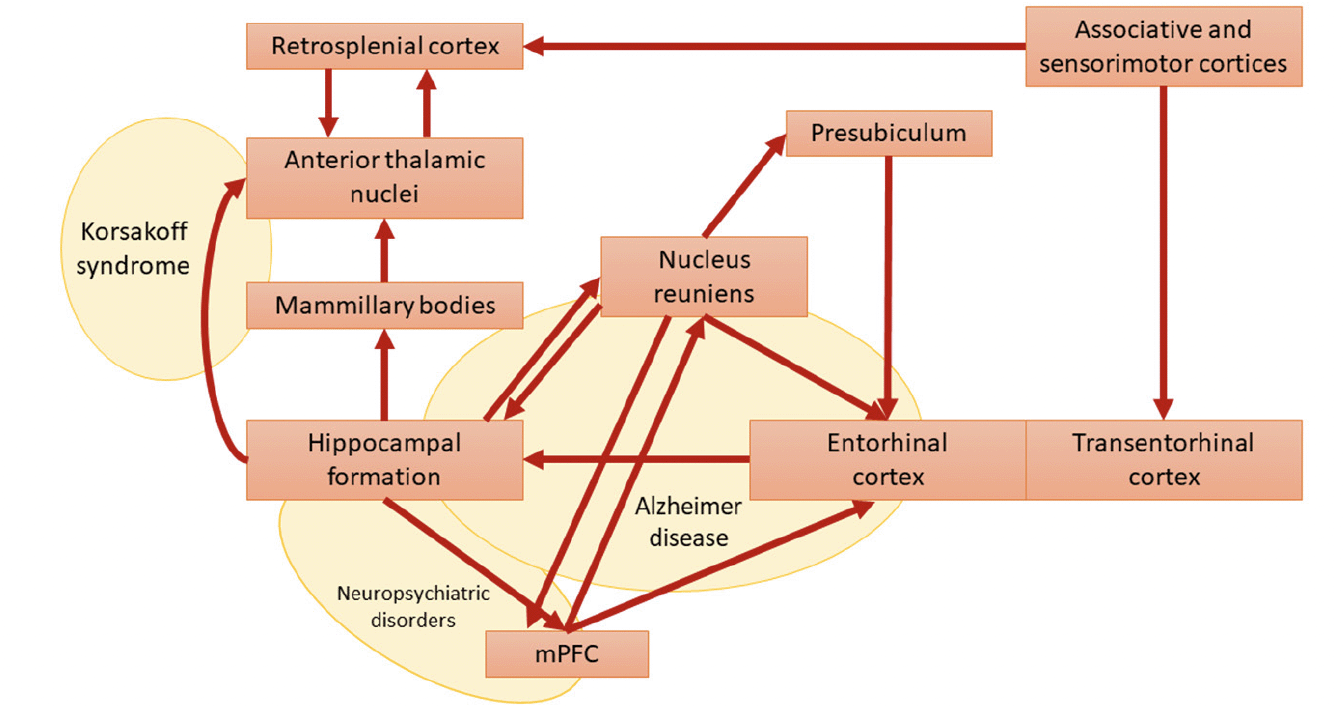
Figure 2. Papez-reuniens circuit in red [as per Braak and Braak nomenclature] and some possible lesion-derived clinical syndromes in yellow
Modified from (19). Abbreviation: mPFC, medial prefrontal cortex
While the syndromes mentioned above differ in their clinical presentations, they share progressive decline in mnemonic and cognitive functions. Therefore, the Papez circuit can be affected in various ways, but the resulting clinical symptoms are more homogeneous in their expression. This clinicopathological bottleneck (Figure 3) may help elucidate converging clinical manifestations of different neurological diseases.
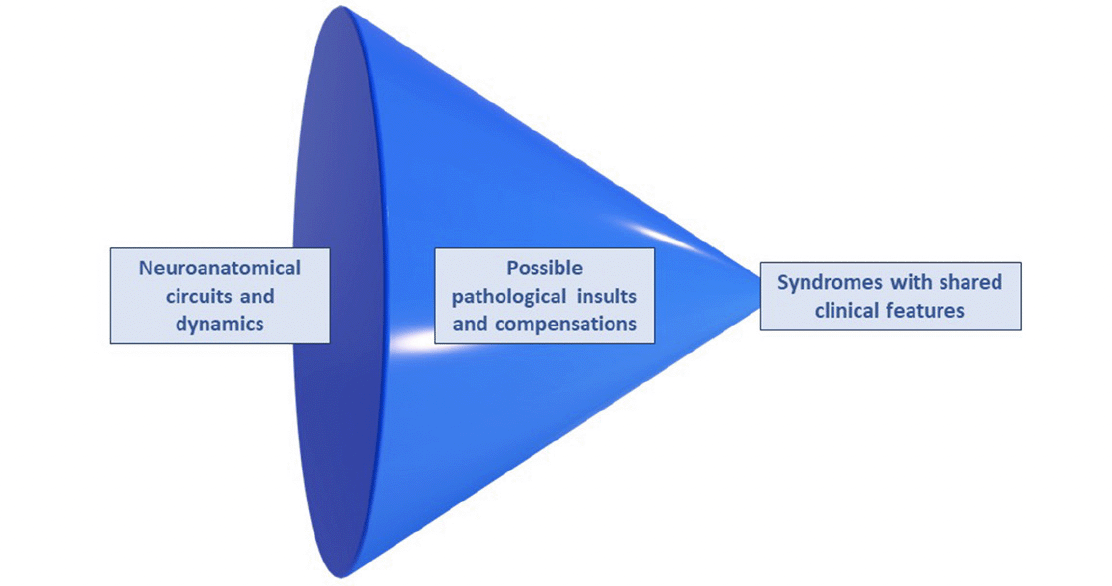
Figure 3. Clinicopathological bottleneck showing how different diseases share converging common manifestations
In simpler terms, while the connectivity of cortical and subcortical structures (and their potential deficits) is multidimensional, the clinical expression exhibits lower dimensionality (52). Considering the anatomopathological staging proposed by Braak and Braak, neuropathological changes in the nucleus reuniens emerge in stages 3-4, overlapping with marked deterioration of symptoms (20). Intriguingly, Braak & Braak’s studies revealed that the pace of neurofibrillary tangles accumulation in the nucleus reuniens mirrors what found in mammillary bodies, subiculum, and entorhinal cortex (20). Therefore, the degeneration of the nucleus reuniens and its connections could be viewed as one of the critical tipping points for AD progression, when the circuit’s topology and dynamics can no longer compensate for ongoing degeneration (53–55).
Another interesting result of the present study is the tendency for significant atrophy of the AV nuclei in cMCI compared to sMCI. The AV nuclei and nucleus reuniens share connections with cortical and subcortical structures but, despite their apparent functional similarities, do exhibit specific differences. According to the model proposed by Mathiasen and colleagues (56), the AV contributes to developing a spatial navigation system through its connections with the hippocampus. This function is also crucial for navigation in the mental world (e.g., episodic memory, (57)). Compared to the AV, the output of the nucleus reuniens to the hippocampus is less stable, suggesting a role in the flexible updating of the map constructed through AV. Based on this, the spatial gradient of morphometric alterations observed in the thalamus might be associated with the initial cognitive deterioration observed in MCI. While the AV nucleus is only partially affected, making it still possible to use spatial/cognitive maps, the evident atrophy of the nucleus reuniens would specifically impair the flexibility in using these maps. While we did not observe a significant correlation between MRI and neuropsychological outcomes, the present speculation might be tested using more sensitive and longitudinal cognitive evaluations that distinguish the capacity to form a map from the ability to use it flexibly.
The present study has several strengths. Firstly, fully automated algorithms for anatomical parcellation and measurement of pathological changes in thalamic nuclei, implemented in Freesurfer v.7.3, ensure consistent and dependable measurements. This is particularly advantageous when dealing with large sample sizes and regular clinical settings (58–60). Secondly, the substantial sample drawn from the multimodal ADNI-3 database allowed stringent screening criteria and a 4-year clinical follow-up, ensuring diagnostic accuracy within the HC and s-MCI groups. Third, the integration of probabilistic techniques and a priori information from ex-vivo MRI and histology to analyze MRI raw data enabled a precise evaluation of relatively small thalamic nuclei, such as the nucleus reuniens, which are difficult to isolate using standard imaging data analysis techniques. At the same time, several limitations of the study should be acknowledged. First, the cross-sectional design fails to capture longitudinal shifts in brain atrophy, which could provide invaluable insights into forecasting the progression to more advanced disease stages. The inclusion of longitudinal MRI analyses would allow more comprehensive understanding of the timing, spatial distribution, and advancement of hippocampal and amygdala involvement in AD. Second, a more precise segmentation could be achieved using scanners with higher fields (e.g., 7T). However, no patient database with this critical feature is currently available. Third, the sample size used in the present study was relatively small. Our results must be replicated in a greater sample when larger databases are made available. Notably, we deliberately chose to include only subjects with complete information at baseline and 48 months. We believe that our stringent inclusion criteria enhance our database’s quality and our findings’ accuracy. Moreover, we acknowledge that the small sample size of s-MCI might influence the negative results. Nevertheless, the presence of a significant (and robust) effect between s-MCI and c-MCI, which is the key finding of our study, suggests that the numerosity of the s-MCI, affected by our stringent inclusion criteria, does not hinder the detection of structural differences, when present.
In conclusion, understanding the intricate dynamics of cortico-subcortical dysfunction upon the clinical transition to AD provides valuable information for optimizing clinical approaches and identifying potential MCI subjects at risk of AD progression.
*Data used in preparation of this article were obtained from the Alzheimer’s Disease Neuroimaging Initiative (ADNI) database (adni.loni.usc.edu). As such, the investigators within the ADNI contributed to the design and implementation of ADNI and/or provided data but did not participate in the analysis or writing of this report. A complete listing of ADNI investigators can be found at: http://adni.loni.usc.edu/wp-content/uploads/how_to_apply/ADNI_Acknowledgement_List.pdf
Acknowledgments: Data collection and sharing for this project was funded by the Alzheimer’s Disease Neuroimaging Initiative (ADNI) (National Institutes of Health Grant U01 AG024904) and DOD ADNI (Department of Defense award number W81XWH-12-2-0012). ADNI is funded by the National Institute on Aging, the National Institute of Biomedical Imaging and Bioengineering, and through generous contributions from AbbVie, Alzheimer’s Association, Alzheimer’s Drug Discovery Foundation, Araclon Biotech, and BioClinica. Inc.; Biogen; Bristol-Myers Squibb Company; CereSpir. Inc.; Cogstate; Eisai Inc.; Elan Pharmaceuticals. Inc.; Eli Lilly and Company; EuroImmun; F. Hoffmann-La Roche Ltd and its affiliated company Genentech. Inc.; Fujirebio; GE Healthcare; IXICO Ltd.; Janssen Alzheimer Immunotherapy Research & Development. LLC.; Johnson & Johnson Pharmaceutical Research & Development LLC.; Lumosity; Lundbeck; Merck & Co. Inc.; Meso Scale Diagnostics. LLC.; NeuroRx Research; Neurotrack Technologies; Novartis Pharmaceuticals Corporation; Pfizer Inc.; Piramal Imaging; Servier; Takeda Pharmaceutical Company; and Transition Therapeutics. The Canadian Institutes of Health Research provides funds to support ADNI clinical sites in Canada. Private sector contributions are facilitated by the Foundation for the National Institutes of Health (www.fnih.org). The grantee organization is the Northern California Institute for Research and Education. and the study is coordinated by the Alzheimer’s Therapeutic Research Institute at the University of Southern California. ADNI data are disseminated by the Laboratory for Neuro Imaging at the University of Southern California.
Funding: This work was supported by Search of Excellence (University «G. d’Annunzio» of Chieti- Pescara; Dr. Stefano Delli Pizzi); the Italian Ministry of Health. the AIRAlzh Onlus (ANCC-COOP. Stefano L Sensi); the Alzheimer’s Association – Part the Cloud: Translational Research Funding for Alzheimer’s Disease (18PTC-19-602325; Stefano L Sensi) and the Alzheimer’s Association – GAAIN Exploration to Evaluate Novel Alzheimer’s Queries (GEENA-Q-19-596282; Stefano L Sensi). Open access funding provided by Università degli Studi G. D’Annunzio Chieti Pescara within the CRUI-CARE Agreement.
Disclosure statement: Valentina Tomassini has received honoraria, travel grants, and research grant support from FISM, the Italian Ministry of Health, Alexion, Roche, Merck, Biogen, Novartis, Viatris, Bristol Myers Squibb, Almirall, Horizon, Lundbeck, Sanofi, Janssen.
Ethical standards: ADNI obtained all IRB approvals and met all ethical standards in the collection of data. The following are the ethics committees and IRB boards that provided approval.
Open Access: This article is distributed under the terms of the Creative Commons Attribution 4.0 International License (http://creativecommons.org/licenses/by/4.0/), which permits use, duplication, adaptation, distribution and reproduction in any medium or format, as long as you give appropriate credit to the original author(s) and the source, provide a link to the Creative Commons license and indicate if changes were made.
References
1. Jack CR, Knopman DS, Jagust WJ, Petersen RC, Weiner MW, Aisen PS, et al. Tracking pathophysiological processes in Alzheimer’s disease: an updated hypothetical model of dynamic biomarkers. The Lancet Neurology 2013;12:207–16. https://doi.org/10.1016/S1474-4422(12)70291-0.
2. Delli Pizzi S, Punzi M, Sensi SL. Functional signature of conversion of patients with mild cognitive impairment. Neurobiology of Aging 2019;74:21–37. https://doi.org/10.1016/j.neurobiolaging.2018.10.004.
3. Chandra A, Dervenoulas G, Politis M, for the Alzheimer’s Disease Neuroimaging Initiative. Magnetic resonance imaging in Alzheimer’s disease and mild cognitive impairment. J Neurol 2019;266:1293–302. https://doi.org/10.1007/s00415-018-9016-3.
4. Delli Pizzi S, Granzotto A, Bomba M, Frazzini V, Onofrj M, Sensi SL. Acting Before; A Combined Strategy to Counteract the Onset and Progression of Dementia. CAR 2021;17:790–804. https://doi.org/10.2174/1567205017666201203085524.
5. Aschenbrenner AJ, Gordon BA, Benzinger TLS, Morris JC, Hassenstab JJ. Influence of tau PET, amyloid PET, and hippocampal volume on cognition in Alzheimer disease. Neurology 2018;91:e859–66. https://doi.org/10.1212/WNL.0000000000006075.
6. Igarashi KM. Entorhinal cortex dysfunction in Alzheimer’s disease. Trends in Neurosciences 2023;46:124–36. https://doi.org/10.1016/j.tins.2022.11.006.
7. Schröder J, Pantel J. Neuroimaging of hippocampal atrophy in early recognition of Alzheimer´s disease – a critical appraisal after two decades of research. Psychiatry Research: Neuroimaging 2016;247:71–8. https://doi.org/10.1016/j.pscychresns.2015.08.014.
8. Ekstrom AD, Ranganath C. Space, time, and episodic memory: The hippocampus is all over the cognitive map. Hippocampus 2018;28:680–7. https://doi.org/10.1002/hipo.22750.
9. Aggleton JP, Pralus A, Nelson AJD, Hornberger M. Thalamic pathology and memory loss in early Alzheimer’s disease: moving the focus from the medial temporal lobe to Papez circuit. Brain 2016;139:1877–90. https://doi.org/10.1093/brain/aww083.
10. Delli Pizzi S, Maruotti V, Taylor J-P, Franciotti R, Caulo M, Tartaro A, et al. Relevance of subcortical visual pathways disruption to visual symptoms in dementia with Lewy bodies. Cortex 2014;59:12–21. https://doi.org/10.1016/j.cortex.2014.07.003.
11. Van De Mortel LA, Thomas RM, Van Wingen GA, for the Alzheimer’s Disease Neuroimaging Initiative. Grey Matter Loss at Different Stages of Cognitive Decline: A Role for the Thalamus in Developing Alzheimer’s Disease. JAD 2021;83:705–20. https://doi.org/10.3233/JAD-210173.
12. Forno G, Saranathan M, Contador J, Guillen N, Falgàs N, Tort-Merino A, et al. Thalamic nuclei changes in early and late onset Alzheimer’s disease. Current Research in Neurobiology 2023;4:100084. https://doi.org/10.1016/j.crneur.2023.100084.
13. Biesbroek JM, Verhagen MG, Van Der Stigchel S, Biessels GJ. When the central integrator disintegrates: A review of the role of the thalamus in cognition and dementia. Alzheimer’s & Dementia 2023:alz.13563. https://doi.org/10.1002/alz.13563.
14. Pardilla-Delgado E, Torrico-Teave H, Sanchez JS, Ramirez-Gomez LA, Baena A, Bocanegra Y, et al. Associations between subregional thalamic volume and brain pathology in autosomal dominant Alzheimer’s disease. Brain Communications 2021;3:fcab101. https://doi.org/10.1093/braincomms/fcab101.
15. Ward LM. The thalamus: gateway to the mind. WIRES Cognitive Science 2013;4:609–22. https://doi.org/10.1002/wcs.1256.
16. Rüb U, Stratmann K, Heinsen H, Del Turco D, Ghebremedhin E, Seidel K, et al. Hierarchical Distribution of the Tau Cytoskeletal Pathology in the Thalamus of Alzheimer’s Disease Patients. JAD 2016;49:905–15. https://doi.org/10.3233/JAD-150639.
17. Kepp KP, Robakis NK, Høilund-Carlsen PF, Sensi SL, Vissel B. The amyloid cascade hypothesis: an updated critical review. Brain 2023:awad159. https://doi.org/10.1093/brain/awad159.
18. Granzotto A, Sensi SL. Once upon a time, the Amyloid Cascade Hypothesis. Ageing Research Reviews 2024;93:102161. https://doi.org/10.1016/j.arr.2023.102161.
19. Braak H, Braak E. Alzheimer’s disease affects limbic nuclei of the thalamus. Acta Neuropathol 1991;81:261–8. https://doi.org/10.1007/BF00305867.
20. Braak H, Braak E. Neuropathological stageing of Alzheimer-related changes. Acta Neuropathol 1991;82:239–59. https://doi.org/10.1007/BF00308809.
21. Dolleman-van Der Weel MJ, Griffin AL, Ito HT, Shapiro ML, Witter MP, Vertes RP, et al. The nucleus reuniens of the thalamus sits at the nexus of a hippocampus and medial prefrontal cortex circuit enabling memory and behavior. Learn Mem 2019;26:191–205. https://doi.org/10.1101/lm.048389.118.
22. Iglesias JE, Insausti R, Lerma-Usabiaga G, Bocchetta M, Van Leemput K, Greve DN, et al. A probabilistic atlas of the human thalamic nuclei combining ex vivo MRI and histology. NeuroImage 2018;183:314–26. https://doi.org/10.1016/j.neuroimage.2018.08.012.
23. Choi EY, Tian L, Su JH, Radovan MT, Tourdias T, Tran TT, et al. Thalamic nuclei atrophy at high and heterogenous rates during cognitively unimpaired human aging. NeuroImage 2022;262:119584. https://doi.org/10.1016/j.neuroimage.2022.119584.
24. Folstein MF, Folstein SE, McHugh PR. “Mini-mental state.” Journal of Psychiatric Research 1975;12:189–98. https://doi.org/10.1016/0022-3956(75)90026-6.
25. Nasreddine ZS, Phillips NA, Bédirian V, Charbonneau S, Whitehead V, Collin I, et al. The Montreal Cognitive Assessment, MoCA: A Brief Screening Tool For Mild Cognitive Impairment: MOCA: A BRIEF SCREENING TOOL FOR MCI. Journal of the American Geriatrics Society 2005;53:695–9. https://doi.org/10.1111/j.1532-5415.2005.53221.x.
26. Pfeffer RI, Kurosaki TT, Harrah CH, Chance JM, Filos S. Measurement of Functional Activities in Older Adults in the Community. Journal of Gerontology 1982;37:323–9. https://doi.org/10.1093/geronj/37.3.323.
27. Mohs RC, Knopman D, Petersen RC, Ferris SH, Ernesto C, Grundman M, et al. Development of cognitive instruments for use in clinical trials of antidementia drugs: additions to the Alzheimer’s Disease Assessment Scale that broaden its scope. The Alzheimer’s Disease Cooperative Study. Alzheimer Dis Assoc Disord 1997;11 Suppl 2:S13-21.
28. Mohs RC, Cohen L. Alzheimer’s Disease Assessment Scale (ADAS). Psychopharmacol Bull 1988;24:627–8.
29. Mainland BJ, Amodeo S, Shulman KI. Multiple clock drawing scoring systems: simpler is better. Int J Geriat Psychiatry 2014;29:127–36. https://doi.org/10.1002/gps.3992.
30. Moms JC, Heyman A, Mohs RC, Hughes JP, Van Belle G, Fillenbaum G, et al. The Consortium to Establish a Registry for Alzheimer’s Disease (CERAD). Part I. Clinical and neuropsychological assesment of Alzheimer’s disease. Neurology 1989;39:1159–1159. https://doi.org/10.1212/WNL.39.9.1159.
31. Gollan TH, Weissberger GH, Runnqvist E, Montoya RI, Cera CM. Self-ratings of spoken language dominance: A Multilingual Naming Test (MINT) and preliminary norms for young and aging Spanish–English bilinguals. Bilingualism 2012;15:594–615. https://doi.org/10.1017/S1366728911000332.
32. Spreen O, Strauss E. Compendium of Neuropsychological Test. New York: Oxxford University Press; 1998.
33. Rey A. L’examen clinique en psychologie (The Clinical Psychological Examination). Paris: Presse Universitaires de France; 1964.
34. Wechsler D. WMS-R: Wechsler Memory Scale-Revised: Manual. San Antonio, TX: Psychological Corporation; 1987.
35. Cummings J. The Neuropsychiatric Inventory: Development and Applications. J Geriatr Psychiatry Neurol 2020;33:73–84. https://doi.org/10.1177/0891988719882102.
36. Yesavage JA, Brink TL, Rose TL, Lum O, Huang V, Adey M, et al. Development and validation of a geriatric depression screening scale: A preliminary report. Journal of Psychiatric Research 1982;17:37–49. https://doi.org/10.1016/0022-3956(82)90033-4.
37. Morris JC. The Clinical Dementia Rating (CDR): Current version and scoring rules. Neurology 1993;43:2412.2-2412-a. https://doi.org/10.1212/WNL.43.11.2412-a.
38. Feng X, Deistung A, Dwyer MG, Hagemeier J, Polak P, Lebenberg J, et al. An improved FSL-FIRST pipeline for subcortical gray matter segmentation to study abnormal brain anatomy using quantitative susceptibility mapping (QSM). Magnetic Resonance Imaging 2017;39:110–22. https://doi.org/10.1016/j.mri.2017.02.002.
39. Braak H, Braak E. Evolution of the neuropathology of Alzheimer’s disease. Acta Neurologica Scandinavica 1996;94:3–12. https://doi.org/10.1111/j.1600-0404.1996.tb05866.x.
40. Chipika RH, Finegan E, Li Hi Shing S, McKenna MC, Christidi F, Chang KM, et al. “Switchboard” malfunction in motor neuron diseases: Selective pathology of thalamic nuclei in amyotrophic lateral sclerosis and primary lateral sclerosis. NeuroImage: Clinical 2020;27:102300. https://doi.org/10.1016/j.nicl.2020.102300.
41. Dolleman-van Der Weel MJ, Witter MP. The thalamic midline nucleus reuniens: potential relevance for schizophrenia and epilepsy. Neuroscience & Biobehavioral Reviews 2020;119:422–39. https://doi.org/10.1016/j.neubiorev.2020.09.033.
42. Rüb U, Del Tredici K, Schultz C, Ghebremedhin E, De Vos RAI, Jansen Steur E, et al. Parkinson’s disease: the thalamic components of the limbic loop are severely impaired by α-synuclein immunopositive inclusion body pathology. Neurobiology of Aging 2002;23:245–54. https://doi.org/10.1016/S0197-4580(01)00269-X.
43. Sampath D, Sathyanesan M, Newton S. Cognitive dysfunction in major depression and Alzheimer's disease is associated with hippocampus–prefrontal cortex dysconnectivity. NDT 2017;Volume 13:1509–19. https://doi.org/10.2147/NDT.S136122.
44. Segobin S, Laniepce A, Ritz L, Lannuzel C, Boudehent C, Cabé N, et al. Dissociating thalamic alterations in alcohol use disorder defines specificity of Korsakoff’s syndrome. Brain 2019;142:1458–70. https://doi.org/10.1093/brain/awz056.
45. Aggleton JP, Nelson AJD, O’Mara SM. Time to retire the serial Papez circuit: Implications for space, memory, and attention. Neuroscience & Biobehavioral Reviews 2022;140:104813. https://doi.org/10.1016/j.neubiorev.2022.104813.
46. Papez JW. A PROPOSED MECHANISM OF EMOTION. Arch NeurPsych 1937;38:725. https://doi.org/10.1001/archneurpsyc.1937.02260220069003.
47. Vertes RP, Hoover WB, Szigeti-Buck K, Leranth C. Nucleus reuniens of the midline thalamus: Link between the medial prefrontal cortex and the hippocampus. Brain Research Bulletin 2007;71:601–9. https://doi.org/10.1016/j.brainresbull.2006.12.002.
48. Bozic I, Rusterholz T, Mikutta C, Del Rio-Bermudez C, Nissen C, Adamantidis A. Coupling between the prelimbic cortex, nucleus reuniens, and hippocampus during NREM sleep remains stable under cognitive and homeostatic demands. Eur J of Neuroscience 2023;57:106–28. https://doi.org/10.1111/ejn.15853.
49. Ferraris M, Ghestem A, Vicente AF, Nallet-Khosrofian L, Bernard C, Quilichini PP. The Nucleus Reuniens Controls Long-Range Hippocampo–Prefrontal Gamma Synchronization during Slow Oscillations. J Neurosci 2018;38:3026–38. https://doi.org/10.1523/JNEUROSCI.3058-17.2018.
50. Buée-Scherrer V, Buée L, Hof PR, Leveugle B, Gilles C, Loerzel AJ, et al. Neurofibrillary degeneration in amyotrophic lateral sclerosis/parkinsonism-dementia complex of Guam. Immunochemical characterization of tau proteins. Am J Pathol 1995;146:924–32.
51. Shoob S, Buchbinder N, Shinikamin O, Gold O, Baeloha H, Langberg T, et al. Deep brain stimulation of thalamic nucleus reuniens promotes neuronal and cognitive resilience in an Alzheimer’s disease mouse model. Nat Commun 2023;14:7002. https://doi.org/10.1038/s41467-023-42721-5.
52. Corbetta M, Siegel JS, Shulman GL. On the low dimensionality of behavioral deficits and alterations of brain network connectivity after focal injury. Cortex 2018;107:229–37. https://doi.org/10.1016/j.cortex.2017.12.017.
53. Simons M, Levin J, Dichgans M. Tipping points in neurodegeneration. Neuron 2023:S0896627323004336. https://doi.org/10.1016/j.neuron.2023.05.031.
54. Nelson PT, Braak H, Markesbery WR. Neuropathology and Cognitive Impairment in Alzheimer Disease: A Complex but Coherent Relationship. J Neuropathol Exp Neurol 2009;68:1–14. https://doi.org/10.1097/NEN.0b013e3181919a48.
55. Nelson PT, Alafuzoff I, Bigio EH, Bouras C, Braak H, Cairns NJ, et al. Correlation of Alzheimer Disease Neuropathologic Changes With Cognitive Status: A Review of the Literature. J Neuropathol Exp Neurol 2012;71:362–81. https://doi.org/10.1097/NEN.0b013e31825018f7.
56. Mathiasen ML, O’Mara SM, Aggleton JP. The anterior thalamic nuclei and nucleus reuniens: So similar but so different. Neuroscience & Biobehavioral Reviews 2020;119:268–80. https://doi.org/10.1016/j.neubiorev.2020.10.006.
57. Lisman J, Buzsáki G, Eichenbaum H, Nadel L, Ranganath C, Redish AD. Viewpoints: how the hippocampus contributes to memory, navigation and cognition. Nat Neurosci 2017;20:1434–47. https://doi.org/10.1038/nn.4661.
58. Desikan RS, Ségonne F, Fischl B, Quinn BT, Dickerson BC, Blacker D, et al. An automated labeling system for subdividing the human cerebral cortex on MRI scans into gyral based regions of interest. NeuroImage 2006;31:968–80. https://doi.org/10.1016/j.neuroimage.2006.01.021.
59. Fischl B. Automatically Parcellating the Human Cerebral Cortex. Cerebral Cortex 2004;14:11–22. https://doi.org/10.1093/cercor/bhg087.
60. Fischl B, Salat DH, Busa E, Albert M, Dieterich M, Haselgrove C, et al. Whole Brain Segmentation. Neuron 2002;33:341–55. https://doi.org/10.1016/S0896-6273(02)00569-X.
© The Authors 2024