W. Yang1, J. Wang2, J. Guo3,4, A. Dove4, X. Qi1, D.A. Bennett5, W. Xu1,4
1. Department of Epidemiology and Biostatistics, School of Public Health, Tianjin Medical University, Tianjin, China; 2. Department of Epidemiology, College of Preventive Medicine, Army Medical University (Third Military Medical University), Chongqing, China; 3. Department of Nutrition and Health, China Agricultural University, Beijing, China; 4. Aging Research Center, Department of Neurobiology, Care Sciences and Society, Karolinska Institutet, Stockholm, Sweden; 5. Rush Alzheimer’s Disease Center, Rush University Medical Center, Chicago, Illinois, USA
Corresponding Author: Weili Xu, MD, PhD, Dept. of Epidemiology & Biostatistics, School of Public Health, Tianjin Medical University, Qixiangtai Road 22, Heping District, 300070, Tianjin, P.R. China; Aging Research Center, Karolinska Institutet, Tomtebodavägen 18A Floor 10, SE-171 65 Solna, Stockholm, Sweden. Phone: +46 8 524 858 26; Email: weili.xu@ki.se; Xiuying Qi, PhD, Dept. of Epidemiology & Biostatistics, School of Public Health, Tianjin Medical University, Qixiangtai Road 22, Heping District, 300070, Tianjin, P.R. China. Email: qixiuying@tmu.edu.cn
J Prev Alz Dis 2024;3(11):739-748
Published online March 19, 2024, http://dx.doi.org/10.14283/jpad.2024.54
Abstract
BACKGROUND: Cognitive reserve (CR) contributes to preserving cognition when facing brain aging and damage. CR has been linked to dementia risk in late life. However, the association between CR and cognitive changes and brain imaging measures, especially in midlife, is unclear.
OBJECTIVE: We aimed to explore the association of CR with cognitive decline and structural brain differences in middle and older age.
DESIGN: This longitudinal study was from the UK Biobank project where participants completed baseline surveys between 2006 to 2010 and were followed (mean follow-up: 9 years).
SETTING: A population-based study.
PARTICIPANTS: A total of 42,301 dementia-free participants aged 40–70 were followed-up to detect cognitive changes. A subsample (n=34,041) underwent brain magnetic resonance imaging scans.
MEASUREMENTS: We used latent class analysis to generate a CR indicator (categorized as high, moderate, and low) based on education, occupation, and multiple cognitively stimulating activities. Cognitive tests for global and domain-specific cognition were administrated at baseline and follow-up. Total brain, white matter, grey matter, hippocampal, and white matter hyperintensity volumes (TBV, WMV, GMV, HV, and WMHV) were assessed at the follow-up examination. Data were analyzed using mixed-effects models and analysis of covariance.
RESULTS: At baseline, 16,032 (37.9%), 10,709 (25.3%), and 15,560 (36.8%) participants had low, moderate, and high levels of CR, respectively. Compared with low CR, high CR was associated with slower declines in global cognition (β [95% confidence interval]: 0.10 [0.08, 0.11]), prospective memory (0.10 [0.06, 0.15]), fluid intelligence (0.07 [0.04, 0.10]), and reaction time (0.04 [0.02, 0.06]). Participants with high CR had lower TBV, WMV, GMV, and WMHV, but higher HV when controlling for global cognition (corrected P <0.01 for all). The significant relationships between CR and cognition and TBV were present among both middle-aged (<60 years) and older (≥60 years) participants. The CR-cognition association remained significant despite reductions in brain structural properties.
CONCLUSIONS: Higher CR is associated with slower cognitive decline, higher HV, and lower microvascular burden, especially in middle age. Individuals with high CR could tolerate smaller brain volumes while maintaining cognition. The benefit of CR for cognition is independent of structural brain differences. Our findings highlight the contribution of enhancing CR to helping compensate for neuroimaging alterations and ultimately prevent cognitive decline.
Key words: Cognitive function, cognitive reserve, latent class analysis, magnetic resonance imaging, UK Biobank.
Introduction
There is vast individual variability in the trajectories of cognitive aging and susceptibility to age-related brain changes. A proposed explanation for this is the cognitive reserve (CR) hypothesis, which contends that relevant lifetime exposures enhancing CR can foster more adaptability in cognitive processing, providing a buffer against age-related brain damage (1, 2). However, CR, as a latent construct, cannot be assessed directly, and its quantification and measurement have been a challenge.
For practical purposes, CR is commonly defined in terms of proxy indicators based on socio-behavioral variables. Education level is the most widely used proxy measure of CR, and arguably the easiest to measure. Nevertheless, the measure with a single factor seems incomplete and could not fully reflect CR influenced by various experiences (3, 4). Occupational status and engagement in cognitively-loaded activities have been considered as two other key proxy measures of CR recently (5). Similarly, evidence from epidemiological studies has highlighted the benefits of leisure activity (6) and minimal engagement in mentally passive sedentary behavior, such as television viewing (7), for cognitive health. As such, a comprehensive measure of CR should combine multiple proxy measures to consider a wider range of contributions to CR.
In CR-related research, three components are required including a measure of CR (e.g., a proxy), cognitive outcomes, and a measure of brain lesion burden (1). Several previous studies have linked composite indicators of CR to better cognitive functioning in older age (8-11). Magnetic resonance imaging (MRI) scans are widely applied to measure structural alterations in the brain, where differences in regional brain volumes may reflect the traits of vascular neuropathology and neurodegeneration. However, literature on the association between CR and brain structural measures is limited due to the cost of MRI and has shown inconsistent findings. Among cognitively healthy older adults, some studies have suggested that high levels of CR-related factors are associated with larger brain volumes (12-15), while others reported that high CR is related to more brain atrophy (16-18) and still others indicated no significant association (19, 20). So far, open questions remain about whether CR is associated with brain MRI markers and whether the association between CR and cognition varies by brain MRI markers. Moreover, older adults compared with young ones tend to have faster cognitive decline and worse brain health (21, 22). Studies addressing the above associations have focused mostly on older people. However, few studies have so far examined whether CR may also influence cognition and brain status among younger adults.
We hypothesized that a high level of CR is related to slower cognitive decline in both older (≥60) and middle (40–60) age, independent of brain MRI measures. In the current study, we aimed to verify this hypothesis by examining the association between an indicator of lifelong CR and cognitive decline and exploring the relationship between CR and structural brain MRI measures in middle and older age using data from the UK Biobank.
Methods
Study population
The UK Biobank is a community-based prospective cohort study comprising over half a million participants aged 37–73 years recruited from 22 sites across the UK. Between 2006 and 2010, 502,412 individuals participated in the baseline survey and underwent sociodemographic, physical, cognitive, and medical assessments. Between 2014 and 2020 (approximately 9 years later), a subsample (n=48,188) of the population participated in the follow-up examination (including repeated cognitive tests).
Of those 48,188 participants, we excluded 5 with prevalent dementia and 5882 with missing data on CR-related variables, leaving 42,301 dementia-free participants for the analysis of the association between CR and cognitive decline. Next, we additionally excluded 6447 who did not undergo MRI assessments and 1813 with chronic neurological diseases (including Parkinson’s disease, brain cancer, brain hemorrhage, brain abscess, aneurysm, cerebral palsy, encephalitis, head injury, nervous system infection, head or neurological injury, and stroke; see Appendix 1 for more details), leaving 34,041 participants for the analysis of the association between CR and structural brain MRI measures (Figure 1).
Abbreviations: MRI, magnetic resonance imaging
The UK Biobank study received ethical approval from the North West Multi-Centre Research Ethics Committee (21/NW/0157). All enrolled participants provided informed and written consent, and all data used in this study were obtained from the UK Biobank (http://www.ukbiobank.ac.uk) through application 67048.
Data collection
At baseline, information on age, sex, ethnicity, smoking, alcohol consumption, and physical activity was self-reported through computerized touch-screen questionnaires.
Ethnicity was dichotomized as white vs. non-white. Cigarette smoking status was grouped as never, previous, or current smoking. Alcohol consumption was categorized as never, previous, or current drinking. Physical activity was measured in terms of metabolic equivalents (MET) per week using the International Physical Activity Questionnaire short form and classified as low (<600 MET-min/week), moderate (600 to <3000 MET-min/week), and high (≥3000 MET-min/week) (23). Body mass index (BMI; kg/m2) was calculated as weight (kg) divided by the square of height (m). Heart disease was ascertained through medical records and self-reported medical history. Diabetes was identified based on hemoglobin A1c ≥6.5%, fasting plasma glucose ≥126 mg/dl, self-reported history of diabetes, use of glucose-lowering medications, or medical records. Hypertension was identified based on systolic blood pressure ≥140 mm Hg, diastolic blood pressure ≥90 mm Hg, self-reported history of hypertension, use of antihypertensive drugs, or medical records (see Appendix 1 for details on the ascertainment of heart disease, diabetes, and hypertension). The apolipoprotein E (APOE) gene was genotyped and dichotomized as carriers vs. non-carriers of the ε4 allele. Missing values for smoking status (0.2%), alcohol consumption (0.1%), physical activity (12.8%), BMI (0.1%), and APOE ε4 carrier status (15.8%) were assigned a separate category.
Information on the following CR-related factors was collected at baseline based on self-report: education level, occupation, TV viewing time, frequency of confiding, frequency of social connection, and number of leisure activities.
Education. Education was operationalized as the number of years of formal schooling (24, 25) and was classified as 1) no educational qualifications, Certificate of Secondary Education or equivalent, or O levels/General Certificate of Secondary Education or equivalent, 2) A/AS levels or equivalent, or other professional qualifications, 3) National Vocational Qualification, Higher National Diploma, Higher National Certificate or equivalent, or 4) college/university degree.
Occupation. Participants self-reported their employment status and job titles. Each job title was matched to a job code derived from the Standard Occupational Classification 2000 system which was developed by the UK Office of National Statistics [26]. A simplified socio-economic classification (SEC) was directly derivable from a four-digit job code and thus, SEC was an occupationally-based classification and reflected the conditions of occupations. SEC is coded as ordinal variables ranging from 1.1, 1.2, and 2 to 7, with lower values reflecting greater occupational attainment (i.e., jobs requiring higher skill levels and more training) (27, 28). Since about one third of participants were retired at baseline with unavailable job codes, additional data at follow-up on codes of the historical longest-held job were used. Occupational attainment was categorized into four levels: 1) unemployed, looking after home and/or family, unable to work because of sickness or disability, or SEC 5–7, 2) SEC 4 or SEC 3, 3) SEC 2, or 4) SEC 1.2 or SEC 1.1.
Television (TV). TV viewing time (in hours/day) was assessed based on self-reported total hours of daily TV watching and quartiled as 1) ≥4, 2) 3–3.9, 3) 2–2.9, or 4) <2.
Confiding. Frequency of confiding was assessed based on participants’ responses to a question about how often they were able to confide in acquaintances and defined as 1) never, 2) less than about once a month, 3) about once a week, 4) 2–4 times a week, or 5) almost daily.
Social connection. Frequency of social connection was assessed based on participants’ responses to a question about how often they made or received friend/family visits and classified as 1) less than about once a month, 2) about once a week, or 3) more than twice a week.
Leisure activity. Leisure activities included involvement in a sports club or gym, pub or social club, religious group, adult education class, or other group activity. Participants were asked to indicate which activities they engaged in once a week or more often. The level of leisure activity was defined as the number of leisure activities engaged in per week and classified as 1) 0, 2) 1, or 3) 2–5.
All categories with <10% of the sample in the above six variables had been merged with the closest category to yield more informative ones (29), as classified above.
Generation of CR indicator
A CR indicator was created using latent class analysis (LCA) based on education level, occupational attainment, TV viewing time, frequency of confiding, frequency of social connection, and number of leisure activities. LCA is a well-validated statistical approach that uses mixture modeling to find the optimal fitting model for a set of data. This allows for the identification of hidden clusters by grouping multiple observed variables into a latent variable with mutually exclusive latent classes [30]. We identified a three-latent-class model after comprehensively considering statistics regarding model selection and the uncertainty of posterior classification (Bayesian information criterion 5612.19, mean posterior probabilities=0.78).
Latent class 1 was characterized by high levels of CR-related factors in general. Specifically, there were more favorable levels of education, occupational attainment, and TV viewing time. Latent class 2 represented moderate levels of CR-related factors. In this class, frequency of confiding in others and social connection and the number of leisure activities were relatively higher. Latent class 3 was characterized by less favorable levels of all CR-related factors (Supplementary Table 1). More detailed descriptions of the LCA and the six proxy measures of CR are available in Appendix 1.
Assessment of cognitive function
Cognitive function at baseline and follow-up was evaluated via five tests administered through a touchscreen interface, including numeric memory (the longest numeric string correctly recalled), prospective memory (successfully carrying out an instruction after a filled delay), pairs matching (the number of errors when recalling positions of matching cards), fluid intelligence (the number of correct answers to logic/reasoning-type questions), and reaction time (mean time to correctly match cards). More details on the cognitive function tests can be found at https://biobank.ctsu.ox.ac.uk/crystal/label.cgi?id=100026. For the numeric memory and fluid intelligence tests, higher raw scores indicate better cognitive performance. For the pairs matching and reaction time tests, higher raw scores indicate worse cognitive performance, so values were reversed for ease of comprehension. Prospective memory performance was scored as 2 for a correct response on the first attempt, 1 for a correct response on the second attempt, and 0 otherwise. A natural log transformation+1 (ln(x+1)) was applied for the pairs matching test in which scores were zero-inflated and positively skewed. Reaction time was natural-log-transformed to correct a positively skewed distribution. These values from all cognitive tests were individually converted to z-scores, and these were further averaged to yield a measure of global cognition, with higher values reflecting better global cognitive function.
MRI acquisition and processing
The procedure for brain MRI acquisition and processing of imaging-derived phenotypes (IDPs) in the UK Biobank has been described in detail previously (31, 32). Briefly, participants were scanned with a Siemens Skyra 3T scanner with a standard Siemens 32-channel head coil. T1-weighted imaging (resolution: 1.0×1.0×1.0 mm; field-of-view: 208×256×256 matrix) and T2 FLAIR imaging (resolution: 1.05×1.0×1.0 mm; field-of-view: 192×256×256 matrix) were performed to provide volumes of brain tissues and structures. Summary measures of brain structure were generated by an image-processing pipeline developed and run on behalf of the UK Biobank, using publicly available image processing tools including the FMRIB Software Library (FSL) version 5.0.10 and FreeSurfer version 6.0 (33).
In the current study, MRI measures included total brain volume (TBV), white matter volume (WMV), grey matter volume (GMV), hippocampal volume (HV), and white matter hyperintensity volume (WMHV), with the unit in mm3. Values for WMHV were natural-log-transformed due to a positively skewed distribution. Extreme outlying data points (further than ±4 standard deviations [SD] from the mean) were excluded (0.002% of the total IDP data analyzed).
Statistical analysis
All analyses were performed using SAS 9.4 (SAS institute, Cary, NC) and STATA SE 15.0 (StataCorp, College Station, TX, USA). Baseline characteristics of the study population were compared according to CR level, and differences among the CR groups were tested using Chi-square tests for categorical variables and one-way analysis of variance followed by pairwise comparison with Bonferroni correction for continuous variables.
Linear mixed-effects models were used to examine the association of CR level (low CR as reference) with changes in global and domain-specific cognitive function. The fixed effect included the CR indicator, follow-up time, and their interaction. The random effect included random intercept and slope, allowing individual differences to be reflected at baseline and across follow-up. All analyses were adjusted for age, sex, ethnicity, smoking status, alcohol consumption, physical activity level, BMI, heart disease, diabetes, hypertension, and APOE ε4 carrier status in multi-adjusted models. In MRI data analysis, analysis of covariance models were used to examine the differences in the least-squares mean (LSM) of brain structural measures between CR levels, additionally adjusting for head size, head position (in terms of x-, y-, and z-axis position coordinates), table position, and baseline levels of global cognitive function. A post-hoc analysis tested pairwise differences between CR levels using Dunnett’s correction (reference: low CR). In addition, we performed stratified analyses according to age at baseline (middle-aged [<60 years] and older [≥60 years]).
To further explore the role of structural brain differences in the association between CR and global cognitive changes, we additionally adjusted for TBV, WMV, GMV, HV, and WMHV in linear mixed-effects models, respectively. These regional brain volumes were further dichotomized as smaller vs. larger volume categories based on their medians corrected for age, sex, and head size. Smaller TBV, WMV, GMV, and HV as well as larger WMHV indicated relatively worse brain structural properties. Interactions between CR level and volume category for each brain MRI measure on global cognitive decline were tested, and models were then stratified by brain volume category to aid the interpretation of the interaction.
In the supplementary analysis, we repeated the analyses in linear mixed-effects models 1) in the MRI subsample (n=34,041), 2) after excluding participants who developed dementia during the follow-up (n=495) to reduce reverse causality, 3) further adjusting for the Townsend deprivation index, a variable reflecting neighborhood-level socio-economic status, and 4) stratifying by sex to examine potential sex differences in the CR-cognition association. Statistical tests were two-tailed and P-values <0.05 were considered statistically significant.
Results
Characteristics of the study population
At baseline, the mean age of the 42,301 participants was 54.38±7.43 years (range: 40 to 70 years), and 51.8% were female. Of all participants, 16,032 (37.9%) had low CR, 10,709 (25.3%) had moderate CR, and 15,560 (36.8%) had high CR. Compared with those with moderate or high CR, participants with low CR were more likely to be white, current/former smokers, and non-drinkers and to have high levels of physical activity, higher BMI, and a history of heart disease/diabetes/hypertension. In addition, those with low CR tended to have worse cognitive performances on global cognition, numeric memory, prospective memory, pairs matching, fluid intelligence, and reaction time tests. The proportion of APOE ε4 carriers did not differ significantly between the three CR groups (Table 1). The distribution of baseline characteristics according to CR level was similar among the subsample with MRI data (Supplementary Table 2).
Data are presented as means ± standard deviations or number (proportion, %). Missing data: 72 for cigarette smoking; 10 for alcohol consumption; 54 for body mass index; 5425 for physical activity; and 6709 for apolipoprotein E ε4 status.
Association between CR and cognitive decline
Over the follow-up period (median 9.30 years, interquartile range 7.64 to 10.44 years), compared with low CR, moderate/high CR was associated with slower declines in global cognition (β 0.06, 95% confidence interval [CI]: 0.04 to 0.08 / β 0.10, 95% CI: 0.08 to 0.11) and prospective memory (β 0.08, 95% CI: 0.03 to 0.13 / β 0.10, 95% CI: 0.06 to 0.15) in multi-adjusted mixed-effects models. These associations were significant among middle-aged as well as older participants. In addition, high CR was associated with a slower decline in fluid intelligence and reaction time, especially in middle age. No significant association between CR and numeric memory or pairs matching was detected (Figure 2 and Supplementary Table 3). In stratified analysis by APOE genotype, the CR-cognitive decline association was similar in both ε4 carriers and non-carriers (Supplementary Table 4).
Association between CR and structural brain differences
The median of the intervals between the baseline survey and the MRI scan was 9.17 (range 4.23 to 12.85) years. In analysis of covariance models, participants with moderate or high compared with low CR had smaller TBV (LSM difference: -2795, 95% CI: -4240 to -1351 for moderate CR; LSM difference: -3222, 95% CI: -4553 to -1892 for high CR) and WMV (LSM difference: -2152, 95% CI: -3101 to -1203 for moderate CR; LSM difference: -1943, 95% CI: -2817 to -1068 for high CR) along with relatively preserved cognitive function. Furthermore, those with high CR had smaller GMV (LSM difference: -1280, 95% CI: -2034 to -525) and WHMV (LSM difference: -0.05, 95% CI: -0.07 to -0.02) as well as larger HV (LSM difference: 35, 95% CI: 12 to 57). In age-stratified analyses, the associations of high CR with TBV, WMV, and GMV remained significant in both age groups, while the associations of high CR with HV and WMHV were only significant among middle-aged participants (Figure 3 and Supplementary Table 5).
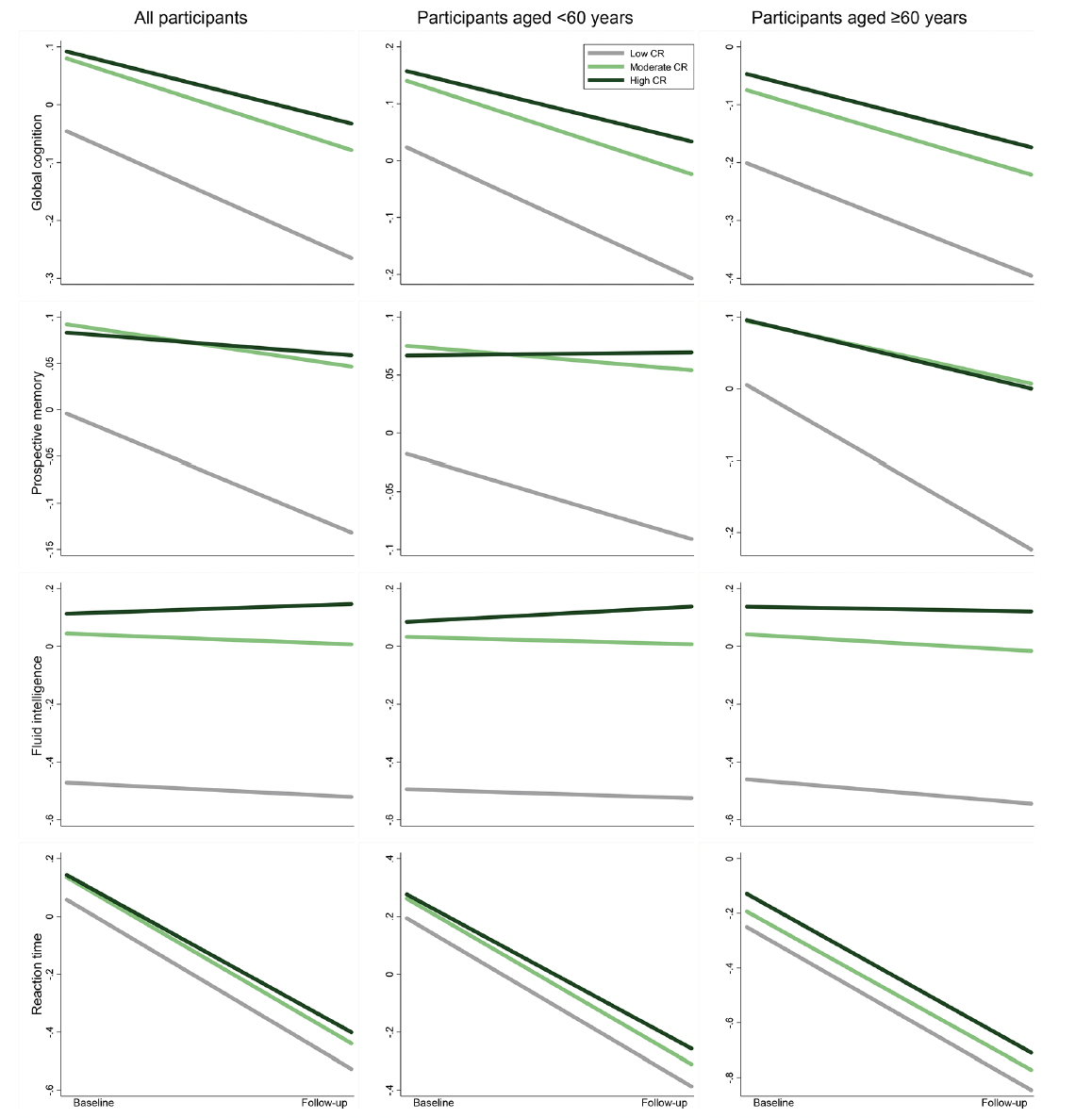
Figure 2. Cognitive trajectories in global cognition and different domains by cognitive reserve (CR) level: results from linear mixed-effects models
The trajectories represent β coefficients adjusted for age, sex, ethnicity, cigarette smoking, alcohol consumption, physical activity, body mass index, heart disease, diabetes, hypertension, and apolipoprotein E ε4.
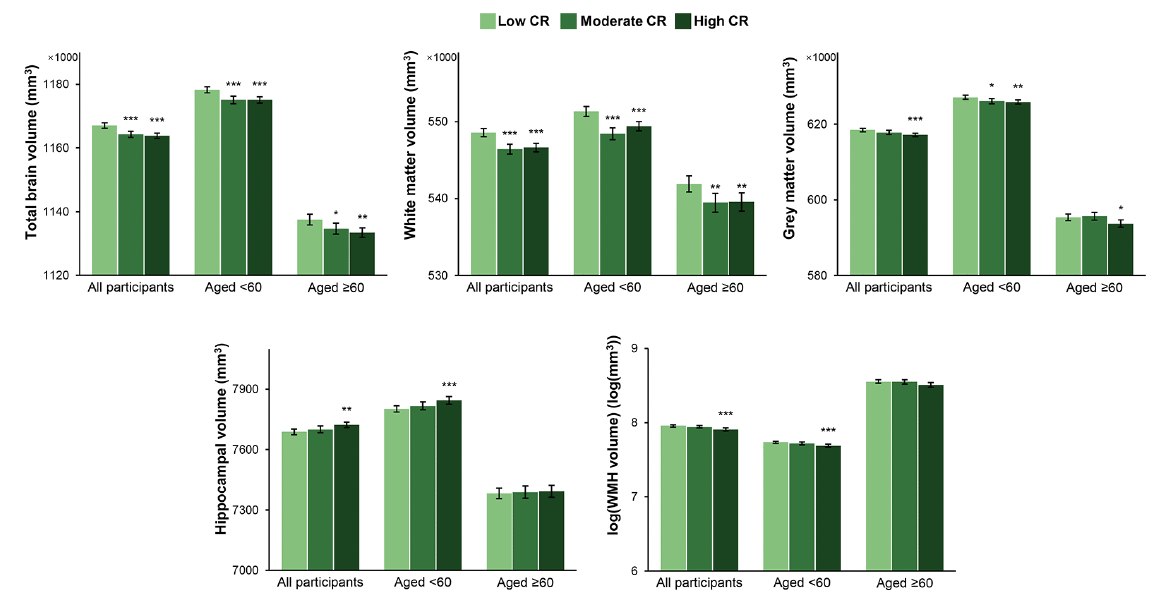
Figure 3. Brain structural magnetic resonance imaging parameters by cognitive reserve (CR) level: results from analysis of covariance models
The bars represent the values of least-squares means (95% confidence intervals) of regional brain volumes adjusted for age, sex, ethnicity, cigarette smoking, alcohol consumption, physical activity, body mass index, heart disease, diabetes, apolipoprotein E ε4, head size, position magnetic resonance imaging confounds, and baseline level of global cognitive function. Asterisks indicate a significant difference compared with the low CR group with Dunnett’s correction (*** P <0.001; ** P <0.01; * P <0.05). Abbreviations: WMH, white matter hyperintensity
Role of structural brain differences in the CR-cognition association
The results on the association between CR and global cognitive function were not substantially altered after additional adjustment for regional brain volumes (Supplementary Table 6). Furthermore, there was no significant interaction between CR and regional brain volumes on global cognition (P >0.05 for all). The effects of moderate and high CR on slower declines in global cognition remained significant among participants with different levels of regional brain volumes (Figure 4 and Supplementary Table 7). These results suggest that the association between CR and global cognitive changes is independent of structural brain MRI measures.
Supplementary analysis
The results were not much altered when we repeated the analysis of CR-cognition association after 1) restricting the study population to just the MRI subsample (Supplementary Table 8), 2) excluding incident dementia cases (Supplementary Table 9), and 3) additionally adjusting for the Townsend deprivation index (Supplementary Table 10). In addition, the association between CR and cognitive decline remained significant in both male and female participants (Supplementary Table 11).
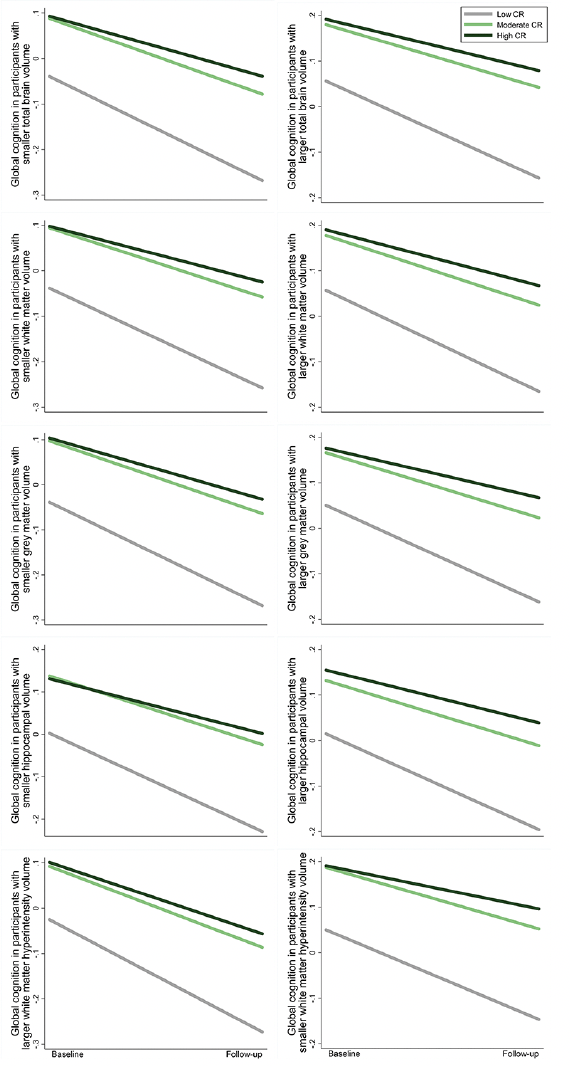
Figure 4. Cognitive trajectories in global cognition by cognitive reserve (CR) level, stratified by brain volume category: results from linear mixed-effects models
The trajectories represent β coefficients adjusted for age, sex, ethnicity, cigarette smoking, alcohol consumption, physical activity, body mass index, heart disease, diabetes, hypertension, and apolipoprotein E ε4.
Discussion
In this large community-based cohort from the UK Biobank, using a comprehensive CR indicator constructed from multiple socio-behavioral proxies, we found that 1) high CR was associated with slower declines in global cognitive function and prospective memory across middle and older ages, and with slower declines in fluid intelligence and reaction time in middle age; 2) high CR was related to smaller TBV but greater HV and less microvascular burden, especially in middle age; and 3) the association between high CR and slower global cognitive decline was independent of structural brain differences.
Accumulating evidence suggests that individual socio-behavioral measures including higher education (34), higher occupational attainment (35), less time spent watching TV (36), and more frequent social interaction (37, 38) are related to better cognitive function. Individually, however, these single components may not fully reflect the CR construct which can be influenced by a range of experiences in life. So far, several studies have evaluated the relationship between CR and changes in cognitive function using a composite index of CR (8, 11, 39). Two of them have shown that higher CR (indexed by education, reading, and vocabulary measures (11) or education and occupation level (39)) is associated with better cognitive performance but not a change in cognition during the follow-up. In our previous work, higher CR (indexed by education, cognitive activity, and social activity) might predict slower declines in global cognitive function, episodic memory, and working memory in older adults (8). The present study used LCA to construct a comprehensive CR indicator, including education level, cognitively stimulating activities, and previously unmeasured proxies like occupational attainment and screen-based sedentary behavior. We also took the further step of evaluating the association between CR and cognition in both middle and older ages. We found that high CR was associated with a slower decline in global cognition, prospective memory, fluid intelligence, and reaction time, especially in middle-aged adults. This suggests that the beneficial effect of CR on some specific cognitive functions may be stronger in midlife and weaken in later life. Additionally, there was no significant sex difference in the observed associations. Our findings underscore the contribution of CR to the maintenance of cognitive function in both women and men, especially earlier in the life course.
In the field of CR, three necessary components should be taken into account: measures of CR, cognitive function, and the burden of brain lesions (1, 40). Neuroimaging techniques have an excellent ability to estimate structural alterations in the brain, thereby allowing the exploration of possible mechanisms underlying the changes in cognition related to CR. Different brain structural changes could reflect different pathological processes. For instance, a reduction in WMV may be indicative of vascular neuropathology (41, 42), whereas a reduction in GMV and HV could reflect neurodegeneration (43). Furthermore, high WMH burden may suggest microvascular lesions in the brain (41).
So far, limited studies have addressed the association between CR and structural neuroimaging measures in dementia-free individuals, and with inconsistent results. Some studies have suggested a positive correlation between the level of CR-related factors and TBV (12, 15), GMV (13, 15, 44), and HV (45), while others have shown that individuals with higher education have lower cortical thickness and greater age-related brain atrophy (16, 17, 46), even for young adults. In addition, another literature has reported a decreased GMV in cognitively healthy older persons with high CR (indexed by education and cognitive activity) (18). The discrepancies could be due to differences in the age of the study population, assessment of CR-related factors, and inclusion or exclusion of participants with neurological diseases. Additionally, many of these previous studies were limited by relatively small sample sizes ranging from 16 to 348. In the current study including brain MRI data from over 30,000 dementia- and neurological disease-free participants, we found that high CR was related to smaller TBV, WMV, and GMV in both middle-aged and older adults, controlling for cognitive level. This did not necessarily imply, however, that high CR could lead to reductions in brain volumes. Instead, our findings of greater brain atrophy in conjunction with relatively well-preserved cognitive functioning for those possessing high CR are consistent with the reserve hypothesis, which suggests that individuals with higher lifelong engagement in various reserve-enhancing activities can tolerate or cope with more age-related brain changes without exhibiting cognitive impairment (1, 47). Thus, CR might play a neuro-compensatory role. Indeed, in our study, the association between high CR and preserved cognitive function remained significant in the presence of reductions in brain structural properties and was independent of brain MRI measures.
These results further suggest that the mechanism underlying the association between CR and cognition might not fully relate to structural brain changes but rather other pathways. For example, high CR might confer more efficient cognitive processing strategies, including the use of brain networks and the ability to call up alternate networks, which is beneficial to cognitive performance (2). High CR may also contribute to greater neuronal density and neurotrophic changes in the prefrontal lobe (48), which helps compensate for or cope with brain aging. On the other hand, consistent with earlier studies (45, 49, 50), we also found that high CR was related to a greater HV and less WMH burden in middle age. This suggests that high CR might have a neuroprotective effect in some brain regions; while with brain aging, there might be a continuous transition in the role of CR from neuroprotective to neuro-compensatory (51). Further population-based brain imaging studies with longitudinal assessments and different neuroimaging measures beyond structural MRI (such as the measurement and characterization of functional brain processes, thought as the closest direct measure of CR (1)) are warranted to better understand the relationship between CR and brain MRI measures.
The main strength of this study lies in the use of LCA to develop a composite CR indicator incorporating multiple CR-related factors with a good overall fit. Additionally, information on structural brain differences from MRI scans has been taken into consideration in this study, allowing us to assess possible mechanistic explanations for the association between CR and cognition. Nevertheless, the following limitations should be acknowledged. First, since the brain MRI scans were conducted about 9 years after baseline, we could not consider baseline levels of MRI measures and could not evaluate the longitudinal association between CR and long-term changes in brain structure. Second, because the assessment of cognitive trajectories did not occur after the MRI scans, it is challenging to fully examine whether CR can modulate the effect of structural brain differences on subsequent cognitive changes. However, the model as we conducted is also sufficient; it examines the association between the CR indicator and cognition after partialing out the role of brain changes and the association between the CR indicator and brain measures when controlling for cognitive level (1, 47). Third, we could not take into account a non-linear cognitive trajectory over the follow-up, as cognitive function was assessed at two time points. Fourth, the cognitive tests administered in the UK Biobank are brief and non-standard in nature, and some may have low reliability. Despite this, improvement in reliability has been shown when these cognitive tests are combined (52), hence our focus on global as well as domain-specific cognitive function. Finally, the UK Biobank population is predominantly white and highly educated, so our findings should be generalized to other populations with caution.
In conclusion, our study shows that higher CR is associated with slower declines in global and some domain-specific cognitive functions in both middle and older ages. Individuals with high CR are able to withstand or cope with smaller brain volumes without observable deficits in cognition. The protective role of CR on cognition is independent of structural brain differences. Our findings highlight that enhancing CR may help the acquisition of abilities to compensate for neuroimaging alterations and ultimately help in the prevention of cognitive decline. Further large population-based longitudinal studies are required to investigate the mechanisms underlying the association between CR and cognitive health.
Funding: This study was supported by grants from the Swedish Research Council (2021-01647), the Swedish Council for Health Working Life and Welfare (2021-01826), and Karolinska Institutet Research Foundation (2022). This work was supported in part by the National Institutes of Health (R01AG17917). The sponsors had no role in the design and conduct of the study; in the collection, analysis, and interpretation of data; in the preparation of the manuscript; or in the review or approval of the manuscript. Open Access funding provided by Karolinska Institute.
Acknowledgments: The authors would like to thank the UK Biobank participants for dedicating their time toward this project and the UK Biobank team for collecting, preparing, and providing data used in this work.
Author Contribution: W.X. and W.Y. contributed to the conception and design of the study. W.Y. conducted the statistical analyses with support from J.W., performed the literature search, and drafted the manuscript. J.W., J.G., A.D., X.Q., D.B., and W.X. reviewed and edited the manuscript. All authors critically revised the manuscript for important intellectual content. All authors made a significant contribution to finalizing the manuscript and approved the final version for publication.
Conflicts of Interest: The authors report no disclosures relevant to the manuscript.
Data Availability: Access to UK Biobank data can be requested through a standard data access procedure. Requests to access these datasets should be directed to http://www.ukbiobank.ac.uk/register-apply.
Ethical Standards: The authors assert that all procedures contributing to this work comply with the ethical standards of the relevant national and institutional committees on human experimentation and with the Helsinki Declaration of 1975, as revised in 2008.
Open Access: This article is distributed under the terms of the Creative Commons Attribution 4.0 International License (http://creativecommons.org/licenses/by/4.0/), which permits use, duplication, adaptation, distribution and reproduction in any medium or format, as long as you give appropriate credit to the original author(s) and the source, provide a link to the Creative Commons license and indicate if changes were made.
References
1. Stern Y, Arenaza-Urquijo EM, Bartres-Faz D, et al. Whitepaper: Defining and investigating cognitive reserve, brain reserve, and brain maintenance. Alzheimers Dement 2020;16(9):1305-1311. doi:10.1016/j.jalz.2018.07.219
2. Stern Y. What is cognitive reserve? Theory and research application of the reserve concept. Journal of the International Neuropsychological Society : JINS 2002;8(3):448-460. doi: 10.1017.S1355617701020240
3. Stern Y. Cognitive reserve in ageing and Alzheimer’s disease. Lancet Neurol 2012;11(11):1006-1012. doi:10.1016/S1474-4422(12)70191-6
4. Bartres-Faz D, Arenaza-Urquijo EM. Structural and functional imaging correlates of cognitive and brain reserve hypotheses in healthy and pathological aging. Brain Topogr 2011;24(3-4):340-357. doi:10.1007/s10548-011-0195-9
5. Opdebeeck C, Martyr A, Clare L. Cognitive reserve and cognitive function in healthy older people: a meta-analysis. Neuropsychol Dev Cogn B Aging Neuropsychol Cogn 2016;23(1):40-60. doi:10.1080/13825585.2015.1041450
6. Wang HX, Jin Y, Hendrie HC, et al. Late life leisure activities and risk of cognitive decline. J Gerontol A Biol Sci Med Sci 2013;68(2):205-213. doi:10.1093/gerona/gls153
7. Hoang TD, Reis J, Zhu N, et al. Effect of Early Adult Patterns of Physical Activity and Television Viewing on Midlife Cognitive Function. JAMA Psychiatry 2016;73(1):73-79. doi:10.1001/jamapsychiatry.2015.2468
8. Li X, Song R, Qi X, et al. Influence of Cognitive Reserve on Cognitive Trajectories: Role of Brain Pathologies. Neurology 2021;97(17):e1695-e1706. doi:10.1212/WNL.0000000000012728
9. Chan D, Shafto M, Kievit R, et al. Lifestyle activities in mid-life contribute to cognitive reserve in late-life, independent of education, occupation, and late-life activities. Neurobiol Aging 2018;70:180-183. doi:10.1016/j.neurobiolaging.2018.06.012
10. Jefferson A, Gibbons L, Rentz D, et al. A life course model of cognitive activities, socioeconomic status, education, reading ability, and cognition. Journal of the American Geriatrics Society 2011;59(8):1403-1411. doi:10.1111/j.1532-5415.2011.03499.x
11. Soldan A, Pettigrew C, Cai Q, et al. Cognitive reserve and long-term change in cognition in aging and preclinical Alzheimer’s disease. Neurobiol Aging 2017;60:164-172. doi:10.1016/j.neurobiolaging.2017.09.002
12. Sole-Padulles C, Bartres-Faz D, Junque C, et al. Brain structure and function related to cognitive reserve variables in normal aging, mild cognitive impairment and Alzheimer’s disease. Neurobiol Aging 2009;30(7):1114-1124. doi:10.1016/j.neurobiolaging.2007.10.008
13. Arenaza-Urquijo EM, Landeau B, La Joie R, et al. Relationships between years of education and gray matter volume, metabolism and functional connectivity in healthy elders. Neuroimage 2013;83:450-457. doi:10.1016/j.neuroimage.2013.06.053
14. Foubert-Samier A, Catheline G, Amieva H, et al. Education, occupation, leisure activities, and brain reserve: a population-based study. Neurobiol Aging 2012;33(2):423 e415-425. doi:10.1016/j.neurobiolaging.2010.09.023
15. James BD, Glass TA, Caffo B, et al. Association of social engagement with brain volumes assessed by structural MRI. J Aging Res 2012;2012:512714. doi:10.1155/2012/512714
16. Coffey CE, Saxton JA, Ratcliff G, Bryan RN, Lucke JF. Relation of education to brain size in normal aging: implications for the reserve hypothesis. Neurology 1999;53(1):189-196. doi:10.1212/wnl.53.1.189
17. Querbes O, Aubry F, Pariente J, et al. Early diagnosis of Alzheimer’s disease using cortical thickness: impact of cognitive reserve. Brain 2009;132(Pt 8):2036-2047. doi:10.1093/brain/awp105
18. Arenaza-Urquijo EM, Molinuevo JL, Sala-Llonch R, et al. Cognitive reserve proxies relate to gray matter loss in cognitively healthy elderly with abnormal cerebrospinal fluid amyloid-beta levels. J Alzheimers Dis 2013;35(4):715-726. doi:10.3233/JAD-121906
19. Christensen H, Batterham PJ, Mackinnon AJ, Anstey KJ, Wen W, Sachdev PS. Education, atrophy, and cognitive change in an epidemiological sample in early old age. Am J Geriatr Psychiatry 2009;17(3):218-226. doi:10.1097/JGP.0b013e3181961a84
20. Kalzendorf J, Brueggen K, Teipel S. Cognitive Reserve Is Not Associated With Hippocampal Microstructure in Older Adults Without Dementia. Front Aging Neurosci 2019;11:380. doi:10.3389/fnagi.2019.00380
21. Singh-Manoux A, Kivimaki M, Glymour MM, et al. Timing of onset of cognitive decline: results from Whitehall II prospective cohort study. Brit Med J 2012;344:d7622. doi: 10.1136/bmj.d7622
22. Bethlehem RAI, Seidlitz J, White SR, et al. Brain charts for the human lifespan. Nature 2022;604(7906):525-533. doi:10.1038/s41586-022-04554-y
23. Chudasama YV, Khunti KK, Zaccardi F, et al. Physical activity, multimorbidity, and life expectancy: a UK Biobank longitudinal study. BMC Med 2019;17(1):108. doi:10.1186/s12916-019-1339-0
24. Okbay A, Beauchamp JP, Fontana MA, et al. Genome-wide association study identifies 74 loci associated with educational attainment. Nature 2016;533(7604):539-542. doi:10.1038/nature17671
25. Rietveld CA, Medland SE, Derringer J, et al. GWAS of 126,559 individuals identifies genetic variants associated with educational attainment. Science 2013;340(6139):1467-1471. doi:10.1126/science.1235488
26. Office for National Statistics. Standard Occupational Classification 2000, Volume 1: Structure and descriptions of unit groups. The Stationery Office; 2000. https://www.ons.gov.uk/file?uri=/methodology/classificationsandstandards/standardoccupationalclassificationsoc/socarchive/soc2000tcm771813161.pdf. Accessed 20 Dec. 2022
27. Office for National Statistics. The National Statistics Socio-economic Classification User Manual 2005. https://www.ons.gov.uk/ons/guide-method/classifications/archived-standard-classifications/soc-and-sec-archive/the-national-statistics-socio-economic-classification%2D%2Duser-manual.pdf. Accessed 20 Dec. 2022.
28. Ko H, Kim S, Kim K, et al. Genome-wide association study of occupational attainment as a proxy for cognitive reserve. Brain 2021. doi:10.1093/brain/awab351
29. Sinha P, Calfee CS, Delucchi KL. Practitioner’s Guide to Latent Class Analysis: Methodological Considerations and Common Pitfalls. Crit Care Med 2021;49(1):e63-e79. doi:10.1097/CCM.0000000000004710
30. Collins LM, Lanza ST. Latent class and latent transition analysis: With applications in the social, behavioral, and health sciences: John Wiley & Sons 2009. doi:10.1002/9780470567333
31. Miller KL, Alfaro-Almagro F, Bangerter NK, et al. Multimodal population brain imaging in the UK Biobank prospective epidemiological study. Nat Neurosci 2016;19(11):1523-1536. doi:10.1038/nn.4393
32. Smith S, Almagro FA, Miller K. UK Biobank Brain Imaging Documentation. https://biobank.ndph.ox.ac.uk/showcase/ukb/docs/brain_mri.pdf. Accessed 20 Dec. 2022.
33. Alfaro-Almagro F, Jenkinson M, Bangerter NK, et al. Image processing and Quality Control for the first 10,000 brain imaging datasets from UK Biobank. Neuroimage 2018;166:400-424. doi:10.1016/j.neuroimage.2017.10.034
34. Lovden M, Fratiglioni L, Glymour MM, Lindenberger U, Tucker-Drob EM. Education and Cognitive Functioning Across the Life Span. Psychol Sci Public Interest 2020;21(1):6-41. doi:10.1177/1529100620920576
35. Smart EL, Gow AJ, Deary IJ. Occupational complexity and lifetime cognitive abilities. Neurology 2014;83(24):2285-2291. doi:10.1212/WNL.0000000000001075
36. Fancourt D, Steptoe A. Television viewing and cognitive decline in older age: findings from the English Longitudinal Study of Ageing. Sci Rep 2019;9(1):2851. doi:10.1038/s41598-019-39354-4
37. Liao J, Muniz-Terrera G, Head J, Brunner EJ. Dynamic Longitudinal Associations Between Social Support and Cognitive Function: A Prospective Investigation of the Directionality of Associations. J Gerontol B Psychol Sci Soc Sci 2018;73(7):1233-1243. doi:10.1093/geronb/gbw135
38. Carlson MC, Parisi JM, Xia J, et al. Lifestyle activities and memory: variety may be the spice of life. The women’s health and aging study II. J Int Neuropsychol Soc 2012;18(2):286-294. doi:10.1017/S135561771100169X
39. Vemuri P, Lesnick TG, Przybelski SA, et al. Association of lifetime intellectual enrichment with cognitive decline in the older population. JAMA Neurol 2014;71(8):1017-1024. doi:10.1001/jamaneurol.2014.963
40. Christensen H, Anstey KJ, Leach LS, Mackinnon AJ. Intelligence, education, and the brain reserve hypothesis. The handbook of aging and cognition. Psychology Press 2007:133-188. doi: 10.4324/9780203837665
41. Wardlaw JM, Smith EE, Biessels GJ, et al. Neuroimaging standards for research into small vessel disease and its contribution to ageing and neurodegeneration. Lancet Neurology 2013;12(8):822-838. doi: 10.1016/S1474-4422(13)70124-8
42. Hase Y, Horsburgh K, Ihara M, Kalaria RN. White matter degeneration in vascular and other ageing-related dementias. Journal of Neurochemistry 2018;144(5):617-633. doi:10.1111/jnc.14271
43. Graham WV, Bonito-Oliva A, Sakmar TP. Update on Alzheimer’s Disease Therapy and Prevention Strategies. Annu Rev Med 2017;68:413-430. doi:10.1146/annurev-med-042915-103753
44. Conti L, Riccitelli GC, Preziosa P, et al. Effect of cognitive reserve on structural and functional MRI measures in healthy subjects: a multiparametric assessment. J Neurol 2021;268(5):1780-1791. doi:10.1007/s00415-020-10331-6
45. Valenzuela MJ, Sachdev P, Wen W, Chen X, Brodaty H. Lifespan mental activity predicts diminished rate of hippocampal atrophy. PLoS One 2008;3(7):e2598. doi:10.1371/journal.pone.0002598
46. Im K, Lee JM, Yoon U, et al. Fractal dimension in human cortical surface: multiple regression analysis with cortical thickness, sulcal depth, and folding area. Hum Brain Mapp 2006;27(12):994-1003. doi:10.1002/hbm.20238
47. Song S, Stern Y, Gu Y. Modifiable lifestyle factors and cognitive reserve: A systematic review of current evidence. Ageing Res Rev 2022;74:101551. doi:10.1016/j.arr.2021.101551
48. Valenzuela MJ, Matthews FE, Brayne C, et al. Multiple biological pathways link cognitive lifestyle to protection from dementia. Biol Psychiatry 2012;71(9):783-791. doi:10.1016/j.biopsych.2011.07.036
49. Pettigrew C, Soldan A, Zhu Y, et al. Cognitive reserve and rate of change in Alzheimer’s and cerebrovascular disease biomarkers among cognitively normal individuals. Neurobiol Aging 2020;88:33-41. doi:10.1016/j.neurobiolaging.2019.12.003
50. Suo C, Leon I, Brodaty H, et al. Supervisory experience at work is linked to low rate of hippocampal atrophy in late life. Neuroimage 2012;63(3):1542-1551. doi:10.1016/j.neuroimage.2012.08.015
51. Arenaza-Urquijo EM, Wirth M, Chetelat G. Cognitive reserve and lifestyle: moving towards preclinical Alzheimer’s disease. Frontiers in Aging Neuroscience 2015;7:134. doi: 10.3389/fnagi.2015.00134
52. Fawns-Ritchie C, Deary IJ. Reliability and validity of the UK Biobank cognitive tests. PLoS One 2020;15(4):e0231627. doi:10.1371/journal.pone.0231627
© The Authors 2024