H. Fangfang1,2, H. Xiao1, Z. Shuai1, W. Qiong1, Z. Jingya1, S. Guodong2,3, Z. Yan1,2
1. School of Health Service Management, Anhui Medical University, Hefei 230032, China; 2. Gerontology Institute of Anhui Province, Hefei 230001, P.R. China;
3. Department of Geriatrics, The First Affiliated Hospital of USTC, Division of Life Sciences and Medicine, University of Science and Technology of China, Hefei, Anhui, 230001, P.R. China
Corresponding Author: Prof. Shen Guodong, Department of Geriatrics, The First Affiliated Hospital of USTC, Division of Life Sciences and Medicine, University of Science and Technology of China, 17-Lujiang Road, Hefei, Anhui 230001, P.R. China, E-mail: gdshen@ustc.edu.cn, Tel: 86-551-62282371; Dr. Zhang Yan, School of Health Service Management, Anhui Medical University, 81-Meishan Road, Hefei, Anhui 230032, P.R. China, E-mail: zhangymail@yahoo.com, Tel: 86-551-65161220
J Prev Alz Dis 2022;1(9):126-135
Published online October 23, 2021, http://dx.doi.org/10.14283/jpad.2021.59
Abstract
Background: Many studies have addressed the relationship between environmental factors and cognitive function; however, evidence from China is very rare.
Objectives: To discuss the relationship between the living and built environment and cognitive function among older Chinese adults.
Design: The Anhui Healthy Longevity Survey (AHLS) was used to investigate the ability to control major non-communicable diseases through behavioural techniques among adults aged 60 or older dwelling in Anhui Province. A multistage sampling strategy was used to obtain a representative sample. Cross-sectional data were collected for the analyses.
Setting: The included participants were recruited from four selected cities in Anhui Province, China.
Participants: The participants were adults aged 60 or older and resided in the selected urban or rural communities.
Measurements: The Mini Mental State Examination was used to measure the cognitive status of the participants. Mild cognitive impairment (MCI) was defined as illiteracy with MMSE scores lower than 18, MMSE scores lower than 21 among those educated for 0–6 years, or MMSE scores lower than 25 among those with 6 or more years of education. The living environment was assessed by asking the participants about their daily living conditions. The distances between the participants’ dwellings and the nearest facilities and the proportions of green/blue spaces within 800 m buffers were calculated based on the textural address to indicate the built environment.
Results: The male participants who lived in a non-dusty environment had higher MMSE scores (β=0.828, 95% CI: 0.240, 1.416, p=0.006) and lower risks of mild cognitive impairment (MCI) (OR=0.651, 95% CI: 0.488, 0.868, p=0.003), and the male participants with no access to recreation spaces had lower MMSE scores (β=-1.107, 95% CI: -1.531, -0.684, p<0.001) and higher risks of MCI (OR=1.403, 95% CI: 1.134, 1.737, p=0.002). The female participants who lived far from a supermarket had significantly lower MMSE scores (Q3:β=-0.750, 95% CI: -1.266, -0.233, p adjusted=0.036; Q4: β=-1.184, 95% CI: -1.745, -0.624, p adjusted<0.001) than those who lived near a supermarket (Q1).
Conclusions: The living environment and built environment might have sex-specific associations with cognitive function among older adults.
Key words: Environment, cognitive function, older adults.
Introduction
Dementia, which is responsible for a large burden on both society and families, is receiving increasing attention worldwide. Globally, 47 million people are currently living with dementia, and it has been estimated that this number will increase to 74.7 million in 2030 and reach 131 million in 2050 (1). Stable or declining dementia incidence has been reported in some high-income countries (2); however, due to long survival times, the absolute number of people who have dementia or mild cognitive function (MCI) has increased with population ageing (3, 4). Given that dementia cannot be cured with existing therapy, early prevention, which focuses on delaying the progression of cognitive function decline, has been considered a priority to decrease the disease burden of dementia in the future (1, 4, 5).
Factors at the individual level are usually considered the main cause of the occurrence of cognitive function decline. Those risk factors might be genetic (i.e., APOE gene (6)), sociodemographic (i.e., sex, rural area dwelling (7, 8)) and behavioural (i.e., physical inactivity (9, 10), lack of social interaction (11)). Recently, the living environment, which refers to the surroundings of individuals (e.g., breathable clear air), (12), and the built environment, which refers to structures built by humans (e.g., parks and buildings) (13), have attracted the attention of researchers. Accumulating evidence has revealed the links between these environmental factors and cognitive function (14). Many studies have reported that living environmental factors, such as air pollution (15-17), traffic noise (18, 19), or living near major roads (20), might be related to higher risks of dementia. Additionally, the availability of some built environmental features (e.g., green spaces and amenities) are thought to have protective effects against cognitive decline. For example, some studies suggest that convenience in daily life (21, 22) and natural environment availability (e.g., green spaces and gardens) (23) are related to delayed cognitive decline. The mechanisms linking these environmental factors and cognition might be explained by increased physical activity and social engagement and reduced negative feelings such as depression (24). Interestingly, sex differences were often suggested to exist in these health-related behaviours or psychological well-being status (25-28); however, thus far, sex as a potential modifier in the relationship between environmental factors and cognition has not been well investigated in the existing literature.
Although some research has indicated the possible influence of environmental factors on cognitive function, the strength of the associations seems to vary by setting. For example, local facilities were found to have different relationships with the cognitive function of older adults in countries with different national income levels (29). China is an upper-middle income country that is currently experiencing rapid economic growth, and subsequent rapid urbanization progress along with major environmental change might have the potential to impact cognitive function among older adults. However, research addressing the relationship between environmental factors and cognitive function in China is still very rare.
The objective of the current study was to explore the potential relationships between environmental factors, including the living environment and built environment, and cognitive function among aged Chinese adults. In the context of the predicable dementia burden resulting from the dramatic increase in the number of adults over 60 years of age, the results of this study might provide clues for policymakers to build an age- and dementia-friendly community (30) that can have potential benefit for the maintenance of cognitive function among older adults.
Methods
Study design
The present study was based on data collected from the Anhui Healthy Longevity Survey (AHLS), which was designed to investigate the ability to control major non-communicable diseases through behavioural techniques among adults aged 60 or older who were dwelling in Anhui Province. A multistage sampling strategy was used to obtain a representative sample that considers urban-rural differences. First, four cities (regions) from different geographic locations in Anhui Province were selected purposively. To be more precise, Chuzhou, Lu’an, Xuancheng and Fuyang were chosen to represent the eastern, western, southern and northern geographic locations of Anhui (Supplemental Fig.1), respectively. Second, the geographic jurisdictions of the selected cities (regions) were labelled “urban” or “rural” according to their administrative attributes, and 3-5 communities from “urban” and “rural” areas were selected. Third, the dwellers in the selected urban or rural communities were invited to participate in the current study. The enrolment stopped when the sample size of each city reached approximately 1500, with dwellers from urban and rural communities accounting for equal shares. The data collection was conducted from July to August 2019. In total, 6211 residents from the four cities were invited.
Participants
In the current study, we excluded participants if they 1) were not able to finish the cognitive function assessment (n=32) or 2) had any missing covariate data (n=331). Finally, 5848 (response rate 94.16%) participants were included in the study. Informed consent was obtained from all participants prior to the survey. All procedures complied with the ethical standards of the Anhui Medical University committee (No. 2020H011).
Cognitive function assessment and the mild cognitive impairment criterion
The Mini Mental State Examination (MMSE) was used to measure the cognitive status of the participants. The total possible score of the MMSE scale was 30 points, with higher scores reflecting better cognitive function (31). MCI had been well accepted as a pre-dementia status with a higher risk of developing dementia (32). Since it has been reported that the education level has a significant impact on MMSE performance (33), the education-based criteria for MCI developed by Zhang et al (34) were employed because almost half of the participants in the current study (49.4%) were illiterate. Specifically, MCI was defined as illiteracy with MMSE scores lower than 18, MMSE scores lower than 21 among those educated for 0–6 years, or MMSE scores lower than 25 among those with 6 or more years of education. The MMSE score and presence of MCI were both employed as dependent variables in the current study (35).
Assessment of the living environment and built environment
In the current study, the living environment was assessed by asking the participants about their daily living conditions. The participants were asked whether they were exposed to noise (i.e., traffic noise (19)) and house dust (36), which cause discomfort and interfere with their daily lives. Moreover, the participants were asked whether they had access to recreational space (i.e., activity and fitness facilities for the elderly) within 1 kilometre of their dwellings.
The distances between participants’ dwellings and the nearest supermarket, hospital, restaurant, and park were calculated to indicate the built environment in the current study. First, the full address information of each participant was inputted to an online map tool (https://www.17ditu.com) and then converted into latitude and longitude coordinates. Second, information on parks, restaurants, hospitals and supermarkets were obtained by using Baidu Map and its application designed for locating points of interest (https://lbsyun.baidu.com/index.php?title=jspopular). The four facilities, i.e., parks, restaurants, hospitals and supermarkets, were selected as points of interest because in the context of China, these four facilities might support physical activity, social interactions, medication and daily life of the residents, respectively. Third, the distances from the dwellings to the nearest above-mentioned facilities were calculated based on the coordinates of the two points.
In the current study, green space (e.g., park, natural forest) and blue spaces (e.g., lake, river) (29) were also identified based on the land use layer information provided by the Open Street Map (https://www.openstreetmap.org). The percentages of green and blue spaces within 800 m buffers were calculated using ArcGIS 10.6 to reflect the environment within a 10-minute walking distance of the older adults.
The distribution of the data was checked by drawing histograms. Because of the nonlinear distributions of the measures of the distance to the nearest facilities, quartile-split variables were used from Q1 (reflecting the shortest distance) to Q4 (reflecting the longest distance). Since the distributions of the percentages of green/blue spaces were extremely skewed, those two environmental features were categorized into “any and none”.
Covariates
The covariates in the current study included sociodemographic characteristics (age, sex, city, urban/rural, years of education, marital status, and income), health-related behaviours (smoking, alcohol consumption, sleep quality and physical activity), chronic diseases (hypertension and diabetes) and mental status (depression). The continuous ages of the participants were self-reported. Years of education were classified into three groups (0, 0–6 years and more than 6 years). Marital status was divided into married and not married (including widowed, divorced, or never married). Participants’ yearly income was classified into two groups (lower than 6500 RMB and 6500 RMB or higher).
The participants were categorized into two smoking groups (current smoker, noncurrent smoker) and three alcohol consumption groups (never, former and current). Sleeping quality was assessed by asking the participants “How do you evaluate your sleeping quality in the last month?” The options were “very good”, “good”, “bad” and “very bad”. The continuous daily sedentary hours (37) were self-reported and used as an indicator reflecting the physical activity status of the participants.
Two chronic conditions (hypertension and diabetes) were considered covariates in the current study. The participants were asked whether they had been diagnosed with hypertension/diabetes at a hospital at the county level or above. The participants were classified into two groups (yes/no).
Depression symptoms of the participants were evaluated by the 9-item depression screening instrument—Patient Health Questionnaire (PHQ-9). The instrument assessed the severity of depression by asking about the frequency of occurrence of the major symptoms of depression in the last 2 weeks. The total score of the PHQ-9 was 30, with higher scores indicating more severe depression symptoms. For the current analysis, total PHQ-9 scores were categorized as “not depressed” (0–4) and “depressed” (5–30). Body mass index (BMI) was calculated with the measured height and weight (weight (kg)/height (m)2). According to the Chinese guidelines for the prevention and control of overweight and obesity for adults (38), overweight was defined as a BMI of 24 or higher.
Statistical analysis
A t-test and an analysis of variance were used to test the hypothesis and compare the means of the MMSE score between the groups. Multilevel regression modelling was first considered to analyse the associations between living and built environmental factors and cognitive function, with a random intercept included to account for the nested data structures, as the individuals might live in very similar community settings, for example, in the same building or village. Therefore, a null (empty) model that simultaneously considered community-level and individual-level factors without any exploratory variable was performed to test the between-community variance. However, the results showed non-significant variance, indicating that the clustering of the cognitive function data might be negligible. Thus, multiple logistic regression and linear regression strategies were employed to assess the associations between living environment, built environment and cognitive function by including MMSE score and MCI as dependent variables. Many potential confounding variables, including sociodemographic factors, behavioural factors, chronic disease and mental health status, were introduced as covariates. Specifically, the living environmental factors (noise, dust and recreation spaces within 1 kilometre) and built environmental factors (distance to the nearest parks, restaurants, hospitals, and supermarkets and the presence of green/blue spaces) were introduced into the regression models one-by-one to test their relationships (Model 1). To test the associations between the studied environmental factors and cognition, those with significant associations with the MMSE or MCI were fit into the fully adjusted models (Model 2). Since many studies have indicated that sex might be related to cognitive decline (39-42) and the environment (43, 44) and that some behaviours (e.g., physical activity and social engagement) that may link environmental factors and cognition vary by sex (25-28), sex-stratified analyses were also performed to explore the possible role of sex as a modifier.
To test the robustness of the results, multilevel analyses were also performed as sensitivity analyses to simultaneously explore the community-level and individual-level factors. Therefore, a multilevel data structure comprising 5848 individuals (at level 1) nested within 21 communities (at level 2) was used in the analysis. Multilevel linear and logistic regression analyses were conducted by introducing the continuous MMSE and MCI as dependent variables.
All analyses were conducted by using Stata 15.0 for Windows (Stata Corp, College Station, TX) and IBM SPSS 24.0 for Windows (IBM Corporation, Armonk, NY, USA). Microsoft Excel was employed for the preparation of the figures and graphs. To decrease the type I error, the adjusted p values were calculated (Bonferroni correction) when multiple comparisons were performed. The significance level was set at a p value less than 0.05.
Results
Table 1 shows the descriptive information of the participants enrolled in the current study. The mean age of the participants (with a slightly higher proportion of females) was 71.01 years. Some participants lived in a dusty environment (9.8%). Almost half of the participants (49.11%) enrolled in the current study were illiterate. Half of the participants had high blood pressure (50.24%) or were overweight (50.47%). The mean MMSE score was 21.58.
a. Median
Supplemental Fig 2A shows the median distance from the participants’ residence to the nearest facilities (including supermarkets, parks, restaurants and hospitals) in the four selected cities. The median distance to the nearest supermarket did not vary among the four cities (all less than 1 km); however, the distances to the other facilities had larger variations among cities. The longest median distances to the nearest restaurant, hospital and park were longest in Chuzhou. Among the four facilities, parks seem to be most remote from the participants’ residences. Supplemental Fig 2B reports the proportions of participants with or without green and blue spaces within 800 m buffers. The proportions of participants with access to green spaces were highest in Xuancheng (64%) and lowest in Chuzhou (19.3%). The proportions of participants with access to blue space were relatively low in Chuzhou (20.3%) and higher in Fuyang (45.7%) and Lu’an (43.9%). However, none of the participants lived with blue space within 800 m buffers in Xuancheng.
The MMSE score distribution comparison among different categories of the studied living environment factors is shown in box plots (Fig. 1A). The participants who had a recreation space near their dwellings exhibited better MMSE scores (t=-9.148, p<0.001). Fig. 1B shows the MMSE score distribution comparison among different categories of the studied built environmental factors. The environmental factors distributions by MCI categories are shown in Supplemental Table 1.
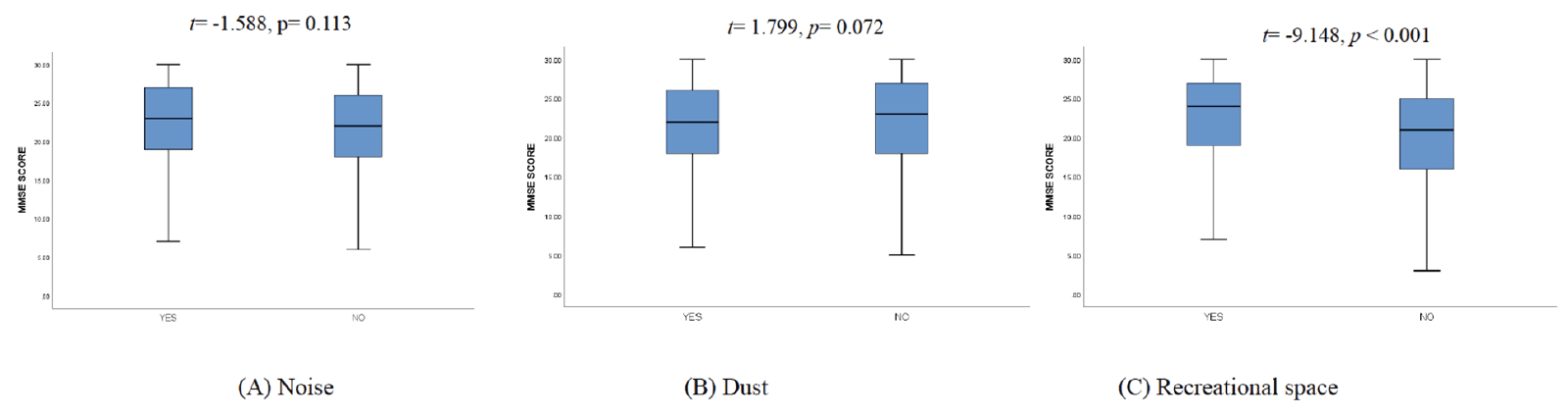
Figure 1A. Box plot showing the MMSE score distribution comparison of different category of the studying living environmental factors
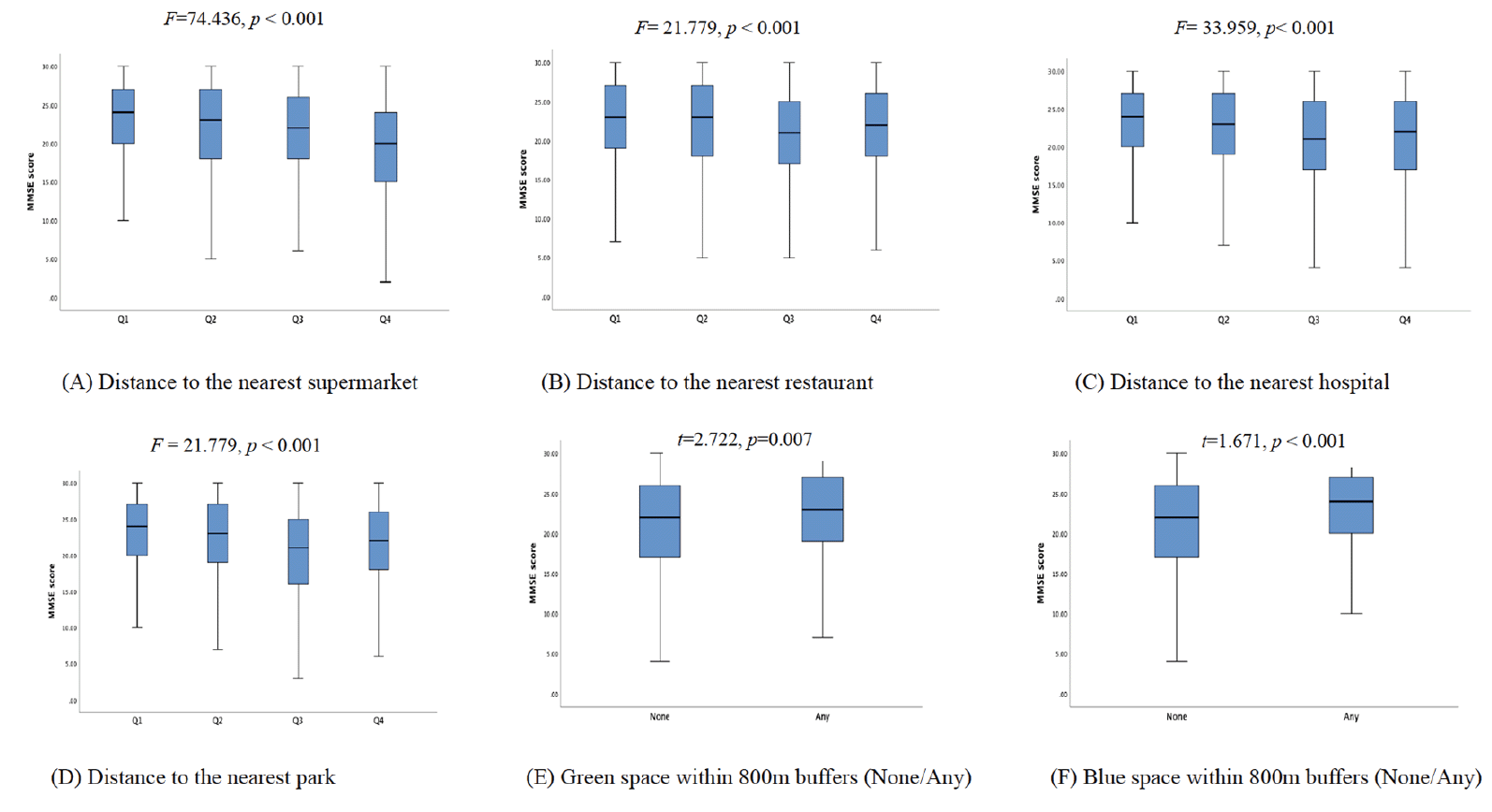
Figure 1B. Box plot showing the MMSE score distribution comparison of different category of the studying living environmental factors
The associations between living environment, built environment factors and cognitive function are shown in Table 2. Among both sexes, the participants without a recreational space near their residence had lower MMSE scores (β==-0.673, 95% CI: -0.969, -0.377, p adjusted<0.001). However, the results of the sex-stratified analysis showed that such associations were found only among male participants. The associations between the built environment factors and cognitive function were generally weak, except for the nearest distance to supermarkets. Among females, the participants living far from the supermarkets had lower MMSE scores. For example, it was estimated that the female participants who lived furthest from a supermarket (Q4) had a lower MMSE score (β=-1.184, 95% CI: -1.745, -0.624, p adjusted<0.001) than those living nearest to a supermarket (Q1). The presence of local blue spaces was associated with the MMSE scores but not MCI. The participants with any blue space within 800 m buffers around the residence were likely to have higher MMSE scores (β=0.594, 95% CI: 0.232, 0.957, p adjusted=0.009) than those who had no blue space nearby. However, the results of the sex-stratified analysis showed that such associations could only be found among females.
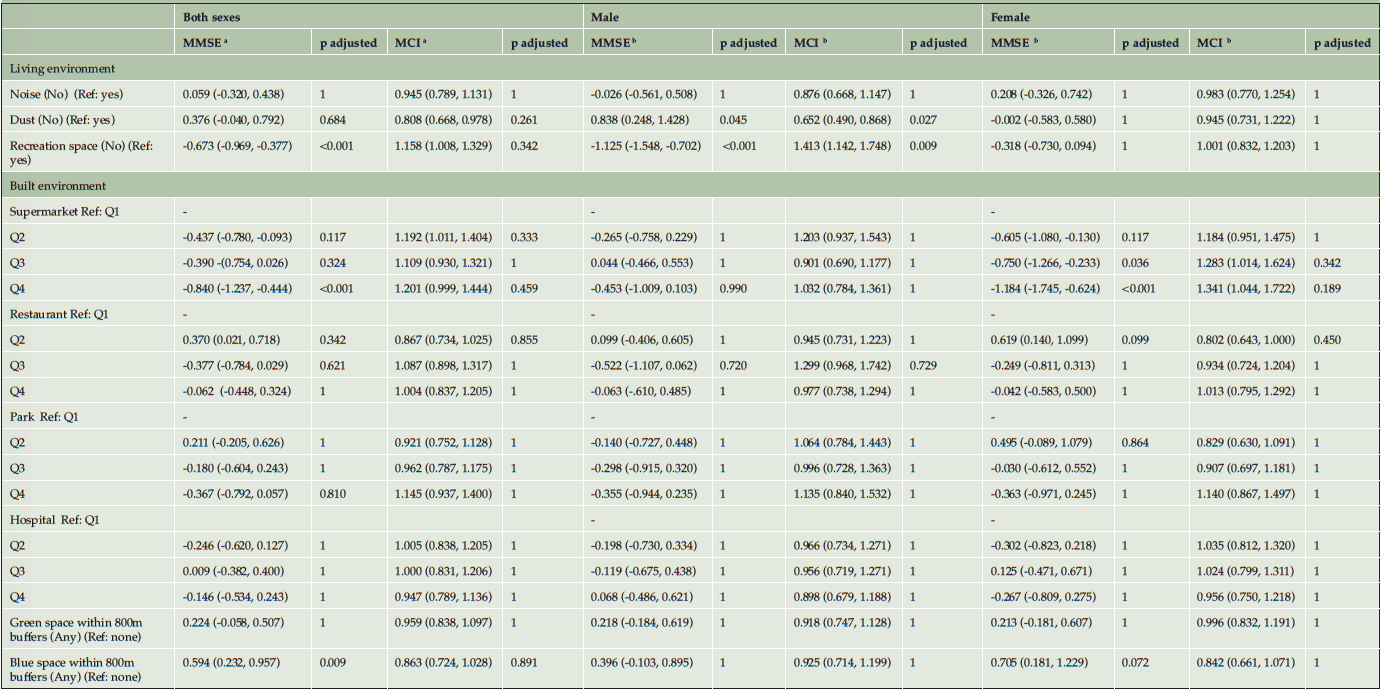
Table 2. The associations between living environment, built environment factors and cognitive function by sexes: Coefficients and 95% CIs for MMSE and Odds Ratios for MCI
a. The model adjusted sex (male and female), age (continuous), location (urban and rural), city (Chuzhou, Lu’an, Xuancheng and Fuyang), education (0, 0–6 years and more than 6 years), marital status (married and not married), income (lower than 6500 RMB and 6500 RMB or higher), diabetes (yes and no), hypertension (yes and no), drinking status (never, former and current), smoking (current smoker, noncurrent smoker), depression (yes and no), overweight (yes and no), sleep quality (very good, good, bad and very bad), and sedentary hours (continuous); b. The model adjusted age (continuous), location (urban and rural), city (Chuzhou, Lu’an, Xuancheng and Fuyang), education (0, 0–6 years and more than 6 years), marital status (married and not married), income (lower than 6500 RMB and 6500 RMB or higher), diabetes (yes and no), hypertension (yes and no), drinking status (never, former and current), smoking (current smoker, noncurrent smoker), depression (yes and no), overweight (yes and no), sleep quality (very good, good, bad and very bad), and sedentary hours (continuous).
The associations between multiple environmental factors and cognitive function among the male participants are presented in Table 3. Among the male participants, those who lived in a non-dusty environment had higher MMSE scores (β==0.828, 95% CI:0.240, 1.416, p=0.006) and a lower risk of MCI (OR=0.651, 95% CI: 0.488, 0.868, p=0.003). The male participants with no access to a recreation space had lower MMSE scores (β=-1.107, 95% CI:-1.531, -0.684, p<0.001) and a higher risk of MCI (OR=1.403, 95% CI: 1.134, 1.737, p=0.002).

Table 3. The associations between multiple environments factors and cognitive function among males: Coefficients and 95% CIs for MMSE and Odds Ratios for MCI
a. The model adjusted age (continuous), location (urban and rural), city (Chuzhou, Lu’an, Xuancheng and Fuyang), education (0, 0–6 years and more than 6 years), marital status (married and not married), income (lower than 6500 RMB and 6500 RMB or higher), diabetes (yes and no), hypertension (yes and no), drinking status (never, former and current), smoking (current smoker, noncurrent smoker), depression (yes and no), overweight (yes and no), sleep quality (very good, good, bad and very bad), and sedentary hours (continuous).
The sensitivity analysis performed using multi-level regression models revealed similar results to the main analysis (Supplemental Table 2). The male participants living in a non-dusty environment had a lower risk of MCI (OR=0.649, 95% CI: 0.487, 0.865, p adjusted=0.027) than those living in a dusty environment. Living without a recreational space near their residence was associated with lower MMSE scores (β=-0.589, 95% CI: -0.892, -0.285, p adjusted<0.001) among both sexes. However, such associations were found only among the male participants. The associations between the built environment factors and cognitive function were generally weak, except for the nearest distance to supermarkets, and such associations were found only among the female participants. The female participants living furthest from a supermarket had lower MMSE scores (β=-0.972, 95% CI: -1.613, -0.332, p adjusted=0.027). The associations between multiple environmental factors and cognitive function among the male participants are presented in Supplemental Table 3. The male participants who lived in a non-dusty environment had higher MMSE scores (β=0.828, 95% CI: 0.243, 1.413, p=0.006) and a lower risk of MCI (OR=0.651, 95% CI: 0.488, 0.868, p=0.003). The male participants with no access to a recreational space had lower MMSE scores (β=-1.107, 95% CI:-1.529, -0.686, p<0.001) and a higher risk of MCI (OR=1.403, 95% CI: 1.134, 1.737, p=0.002).
Discussion
In the current study, we assessed the potential impact of the living environment and built environment on cognitive function among older people who dwell in urban and rural areas of Anhui Province, China. The results of the current study indicate that the living environment and built environment might have the potential to influence the cognitive function of older adults, although the associations seem to be sex specific. Explanations for these potential sex differences should be further explored in future studies. To the best of our knowledge, the current study is the first to assess the associations between environmental factors and cognitive function among older adults dwelling in Anhui, China.
Accumulating evidence suggests that environmental factors might play a role in cognitive function maintenance. For example, a high mix of land uses has been reported to have complex associations with cognitive impairment (45). Natural environments (e.g., gardens, green spaces) were associated with fewer mental disorders among older people (46), which in turn contributed to protecting cognitive function (47, 48). Similar to some previous studies (15, 17, 49), in the current study, male participants living in a non-dusty environment were found to have a lower risk of MCI. Air pollution may influence the physiological ageing process of the brain [50], which might also be linked to cognitive function decline. Generally, the presence of the recreational space might indicate an elevated level of physical activity (51-53), which contributes to the delay of cognitive function decline (54, 55). However, our results suggest that males might benefit more from recreational spaces than females, which might be explained by the higher likelihood of participating in physical activities among males (56, 57).
In this study, female participants who lived far away from supermarkets were more likely to have poorer cognitive function. In the Chinese context, aged housewives often walk to supermarkets with their friends, and such behaviours might have potential contributions to cognitive function maintenance by resulting in physical exercise and increasing the chances of interpersonal communication. However, such associations were not found among male participants. This may be explained by the different family roles of females and males. In China, females are often responsible for purchasing household goods and preparing cooking materials. Some studies had similar findings to our study results. A study conducted in Japan showed that lower food store availability was associated with poorer cognitive function (58), and a study conducted in the United States also showed that living far from convenience stores was associated with poorer cognitive function (29). Given that purchasing foods and other daily necessities at the market is necessary for daily life and is usually a main reason for going out for older Chinese adults, increasing the availability of food stores may contribute to cognitive function decline prevention (58).
This study had many strengths. First, the representativeness of the sample was guaranteed by a multistage sampling strategy and a large sample size. The sampling process considered geographic characteristics and urban–rural disparities. The large sample size of male and female participants and high responsive rate (94.2%) were also helpful in achieving our study objective. Second, we employed a number of covariates to test the independent role of living environment and built environment by controlling the other potential influencing factors so that the validity of our results was verified. Additionally, the measures of the built environment were objectively calculated instead of self-reported by participants so that the correctness of the measures could be ensured.
However, the study also had several limitations. First, the cross-sectional design made it difficult to draw any conclusions related to cause and effect. Future studies might investigate the association between the environment and cognitive function through a longitudinal design. Second, urbanization and urban expansion that have occurred in many cities in China might have resulted in a massive shift in the built environment (59). This process might mask the true associations between built environmental factors and the cognitive function of older adults. Third, the measures of environment employed in the current study might not reflect the real level of health-related behaviour of the participants. For example, people might ignore the recreational space even if it could be reached in minutes. Finally, political and interpersonal environmental factors, which are essential to cognitive function, were not included in the current study. For example, loneliness and isolation, which have been associated with poorer cognitive function among older adults (60), were not included in the current study. In the future, a more comprehensive framework might be employed to address more environmental factors, such as political and interpersonal environments.
Conclusions
In the current study, the living and built environments were associated with cognitive function among older adults, although such associations seem to be sex specific. Since cognitive function decline is highly prevalent among older adults, our findings might provide clues for building age- and dementia-friendly communities, especially in the context of community planning, and facilities, such as recreation spaces and supermarkets, should be considered. In the future, longitudinal studies with comprehensive measures of environmental exposures are required to verify these associations.
Acknowledgments: The authors thank all participants in the AHLS for providing their personal data. We also thank all colleagues involved in the study for their cooperation and efforts in data collection and management.
Funding: This work was supported by the National Natural Science Foundation of China (Grant No. 72004003) to Z.Y, and the Key Projects of Science and Technology of Anhui Province (202004b11020019, 202004j07020020, 2019b12030026, 2019b11030012) to S.G. The funders had no role in the study design,data collection and analysis, decision to publish, or preparation of the manuscript.
Authorship: All authors meet the criteria for authorship according to the COI form and their contributions to the manuscript. H.F. drafted the manuscript. GS and YZ framed the concept and designed the study. The data collection and material preparation were conducted by Z.S., W.Q. and Z.J., and the data analysis was performed by H.X.
Ethics approval and consent to participate: The study protocol was approved by the Ethics Review Committee of Anhui Medical University, Hefei, China (No. 2020H011). Informed consent was obtained from all participants included in the study.
Conflicts of interest: The authors have no conflicts of interest to report.
Open Access: This article is distributed under the terms of the Creative Commons Attribution 4.0 International License (http://creativecommons.org/licenses/by/4.0/), which permits use, duplication, adaptation, distribution and reproduction in any medium or format, as long as you give appropriate credit to the original author(s) and the source, provide a link to the Creative Commons license and indicate if changes were made.
References
1. Belanger M, Gray-Donald K, O’Loughlin J, Paradis G, Hanley J: Influence of weather conditions and season on physical activity in adolescents. Ann Epidemiol 2009, 19(3):180-186. https://dx.doi.org/10.1016/j.annepidem.2008.12.008.
2. Wu YT, Beiser AS, Breteler MMB, Fratiglioni L, Helmer C, Hendrie HC, Honda H, Ikram MA, Langa KM, Lobo A et al: The changing prevalence and incidence of dementia over time – current evidence. Nat Rev Neurol 2017, 13(6):327-339. https://dx.doi.org/10.1038/nrneurol.2017.63.
3. Prince M, Ali GC, Guerchet M, Prina AM, Albanese E, Wu YT: Recent global trends in the prevalence and incidence of dementia, and survival with dementia. Alzheimers Res Ther 2016, 8(1):23. https://dx.doi.org/10.1186/s13195-016-0188-8.
4. Anderson ND: State of the science on mild cognitive impairment (MCI). CNS Spectr 2019, 24(1):78-87. https://dx.doi.org/10.1017/S1092852918001347.
5. Jia J, Wei C, Chen S, Li F, Tang Y, Qin W, Zhao L, Jin H, Xu H, Wang F et al: The cost of Alzheimer’s disease in China and re-estimation of costs worldwide. Alzheimers Dement 2018, 14(4):483-491. https://dx.doi.org/10.1016/j.jalz.2017.12.006.
6. Colovati MES, Novais IP, Zampol M, Mendes GD, Cernach MCS, Zanesco A: Interaction between physical exercise and APOE gene polymorphism on cognitive function in older people. Braz J Med Biol Res 2021, 54(2). https://dx.doi.org/ARTN e1009810.1590/1414-431X202010098.
7. Guo Y, Yang M, Yan YQ, Wang L, Gong J: Sex differentials in relationships between functional fitness and cognitive performance in older adults: a canonical correlation analysis. Sci Rep-Uk 2018, 8. https://dx.doi.org/ARTN 414610.1038/s41598-018-22475-7.2018;8.
8. Nakamura K, Kitamura K, Watanabe Y, Shinoda H, Sato H, Someya T: Rural-urban differences in the prevalence of cognitive impairment in independent community-dwelling elderly residents of Ojiya city, Niigata Prefecture, Japan. Environ Health Prev Med 2016, 21(6):422-429. https://dx.doi.org/10.1007/s12199-016-0542-2.
9. Alty J, Farrow M, Lawler K: Exercise and dementia prevention. Pract Neurol 2020, 20(3):234-240. https://dx.doi.org/10.1136/practneurol-2019-002335.
10. Iuliano E, di Cagno A, Cristofano A, Angiolillo A, D’Aversa R, Ciccotelli S, Corbi G, Fiorilli G, Calcagno G, Di Costanzo A et al: Physical exercise for prevention of dementia (EPD) study: background, design and methods. BMC Public Health 2019, 19(1):659. https://dx.doi.org/10.1186/s12889-019-7027-3
11. Fu C, Li Z, Mao ZF: Association between Social Activities and Cognitive Function among the Elderly in China: A Cross-Sectional Study. Int J Env Res Pub He 2018, 15(2). https://dx.doi.org/ARTN 23110.3390/ijerph15020231.
12. Brugge D, Leong A, Averbach AR, Cheung FM: An environmental health survey of residents in Boston Chinatown. J Immigr Health 2000, 2(2):97-111. https://dx.doi:10.1023/A:1009538002661
13. Gilbert JA, Stephens B: Microbiology of the built environment. Nat Rev Microbiol 2018, 16(11):661-670. https://doi.org/10.1038/s41579-018-0065-5.
14. Cassarino M, Setti A: Environment as ‘Brain Training’: A review of geographical and physical environmental influences on cognitive ageing. Ageing Res Rev 2015, 23:167-182. https://doi.org/10.1016/j.arr.2015.06.003.
15. Carey IM, Anderson HR, Atkinson RW, Beevers SD, Cook DG, Strachan DP, Dajnak D, Gulliver J, Kelly FJ: Are noise and air pollution related to the incidence of dementia? A cohort study in London, England. BMJ Open 2018, 8(9):e022404. https://dx.doi.org/10.1136/bmjopen-2018-022404. https://dx.doi.org/10.1186/s12877-016-0342-y.
16. Killin LO, Starr JM, Shiue IJ, Russ TC: Environmental risk factors for dementia: a systematic review. BMC geriatrics 2016, 16(1):175. https://dx.doi.org/10.1186/s12877-016-0342-y.
17. Paul KC, Haan M, Mayeda ER, Ritz BR: Ambient Air Pollution, Noise, and Late-Life Cognitive Decline and Dementia Risk. Annu Rev Public Health 2019, 40:203-220. https://dx.doi.org/10.1146/annurev-publhealth-040218-044058.
18. Yu Y, Mayeda ER, Paul KC, Lee E, Jerrett M, Su J, Wu J, Shih IF, Haan M, Ritz B: Traffic-related Noise Exposure and Late-life Dementia and Cognitive Impairment in Mexican-Americans. Epidemiology 2020, 31(6):771-778. https://dx.doi.org/10.1097/ede.0000000000001249.
19. Cantuaria ML, Waldorff FB, Wermuth L, Pedersen ER, Poulsen AH, Thacher JD, Raaschou-Nielsen O, Ketzel M, Khan J, Valencia VH et al: Residential exposure to transportation noise in Denmark and incidence of dementia: national cohort study. BMJ 2021, 374:n1954. https://doi.org/10.1136/bmj.n1954.
20. Chen H, Kwong JC, Copes R, Tu K, Villeneuve PJ, van Donkelaar A, Hystad P, Martin RV, Murray BJ, Jessiman B et al: Living near major roads and the incidence of dementia, Parkinson’s disease, and multiple sclerosis: a population-based cohort study. Lancet (London, England) 2017, 389(10070):718-726. https://dx.doi.org/10.1016/s0140-6736(16)32399-6.
21. Clarke PJ, Weuve J, Barnes L, Evans DA, Mendes de Leon CF: Cognitive decline and the neighborhood environment. Ann Epidemiol 2015, 25(11):849-854. https://dx.doi.org/10.1016/j.annepidem.2015.07.001
22. Manson A, Ciro C, Williams KN, Maliski SL: Identity and perceptions of quality of life in Alzheimer’s disease. Appl Nurs Res 2020, 52:151225. https://dx.doi.org/10.1016/j.apnr.2019.151225
23. Wu YT, Prina AM, Jones A, Matthews FE, Brayne C, Medical Research Council Cognitive F, Ageing Study C: The Built Environment and Cognitive Disorders: Results From the Cognitive Function and Ageing Study II. Am J Prev Med 2017, 53(1):25-32. https://dx.doi.org/10.1016/j.amepre.2016.11.020.
24. Besser LM, McDonald NC, Song Y, Kukull WA, Rodriguez DA: Neighborhood Environment and Cognition in Older Adults: A Systematic Review. Am J Prev Med 2017, 53(2):241-251. https://doi.org/10.1016/j.amepre.2017.02.013.
25. Ye L, Xiao J, Fang Y: Heterogeneous Trajectory Classes of Social Engagement and Sex Differences for Older Adults in China. Int J Environ Res Public Health 2020, 17(22). https://doi.org/10.3390/ijerph17228322.
26. Kiely KM, Sutherland G, Butterworth P, Reavley NJ: Age and gender differences in the reciprocal relationship between social connectedness and mental health. Soc Psychiatry Psychiatr Epidemiol 2021, 56(6):1069-1081. https://doi.org/10.1007/s00127-020-01960-3.
27. Jun HJ, Park S: The Effect of Cross-Level Interaction between Community Factors and Social Capital among Individuals on Physical Activity: Considering Gender Difference. Int J Environ Res Public Health 2019, 16(3). https://doi.org/10.3390/ijerph16030495.
28. Liao YH, Kao TW, Peng TC, Chang YW: Gender differences in the association between physical activity and health-related quality of life among community-dwelling elders. Aging Clin Exp Res 2021, 33(4):901-908. https://doi.org/10.1007/s40520-020-01597-x.
29. Wu YT, Brayne C, Liu Z, Huang Y, Sosa AL, Acosta D, Prina M: Neighbourhood environment and dementia in older people from high-, middle- and low-income countries: results from two population-based cohort studies. BMC Public Health 2020, 20(1):1330. https://dx.doi.org/10.1186/s12889-020-09435-5
30. Wu SM, Huang HL, Chiu YC, Tang LY, Yang PS, Hsu JL, Liu CL, Wang WS, Shyu YL: Dementia-friendly community indicators from the perspectives of people living with dementia and dementia-family caregivers. J Adv Nurs 2019, 75(11):2878-2889. https://dx.doi.org/10.1111/jan.14123
31. Arevalo-Rodriguez I, Smailagic N, Roque IFM, Ciapponi A, Sanchez-Perez E, Giannakou A, Pedraza OL, Bonfill Cosp X, Cullum S: Mini-Mental State Examination (MMSE) for the detection of Alzheimer’s disease and other dementias in people with mild cognitive impairment (MCI). Cochrane Database Syst Rev 2015(3):CD010783. https://dx.doi.org/10.1002/14651858.CD010783.pub2.
32. Davis M, T OC, Johnson S, Cline S, Merikle E, Martenyi F, Simpson K: Estimating Alzheimer’s Disease Progression Rates from Normal Cognition Through Mild Cognitive Impairment and Stages of Dementia. Curr Alzheimer Res 2018, 15(8):777-788. https://doi.org/10.2174/1567205015666180119092427.
33. Bravo G, Hebert R: Age- and education-specific reference values for the Mini-Mental and modified Mini-Mental State Examinations derived from a non-demented elderly population. Int J Geriatr Psychiatry 1997, 12(10):1008-1018. https://dx.doi.org/10.1002/(sici)1099-1166(199710)12:10<1008::aid-gps676>3.0.co;2-a.
34. Zhang MY, Katzman R, Salmon D, Jin H, Cai GJ, Wang ZY, Qu GY, Grant I, Yu E, Levy P et al: The prevalence of dementia and Alzheimer’s disease in Shanghai, China: impact of age, gender, and education. Ann Neurol 1990, 27(4):428-437. https://dx.doi.org/10.1002/ana.410270412.
35. Jin X, Shu C, Zeng Y, Liang L, Ji JS: Interaction of greenness and polygenic risk score of Alzheimer’s disease on risk of cognitive impairment. Sci Total Environ 2021, 796:148767.
36. Araki A, Ait Bamai Y, Ketema RM, Kishi R: [House Dust and Its Adverse Health Effects]. Nihon Eiseigaku Zasshi 2018, 73(2):130-137. https://doi.org/10.1016/j.scitotenv.2021.148767.
37. Maasakkers CM, Claassen J, Scarlett S, Thijssen DHJ, Kenny RA, Feeney J, Melis RJF: Is there a bidirectional association between sedentary behaviour and cognitive decline in older adults? Findings from the Irish Longitudinal Study on Ageing. Prev Med Rep 2021, 23:101423. https://doi.org/10.1016/j.pmedr.2021.101423.
38. Belvederi Murri M, Respino M, Proietti L, Bugliani M, Pereira B, D’Amico E, Sangregorio F, Villa V, Trinchero V, Brugnolo A et al: Cognitive impairment in late life bipolar disorder: Risk factors and clinical outcomes. J Affect Disord 2019, 257:166-172. https://dx.doi.org/10.1016/j.jad.2019.07.052.
39. Cuoco S, Picillo M, Cappiello A, Carotenuto I, Erro R, Russillo MC, Abate F, Volpe G, Squillante M, Cozzolino A et al: Effects of gender on cognitive and behavioral manifestations in multiple system atrophy. J Neural Transm (Vienna) 2020, 127(6):925-934. https://dx.doi.org/10.1007/s00702-020-02169-z.
40. Wang J, Xiao LD, Wang K, Luo Y, Li XM: Gender Differences in Cognitive Impairment among Rural Elderly in China. Int J Env Res Pub He 2020, 17(10). https://dx.doi.org/ARTN 372410.3390/ijerph17103724.
41. Subramaniapillai S, Almey A, Natasha Rajah M, Einstein G: Sex and gender differences in cognitive and brain reserve: Implications for Alzheimer’s disease in women. Front Neuroendocrinol 2021, 60:100879. https://dx.doi.org/10.1016/j.yfrne.2020.100879.
42. Miyawaki CE, Liu MH: Gender differences in cognitive impairment among the old and the oldest-old in China. Geriatrics & Gerontology International 2019, 19(7):586-592. https://dx.doi.org/10.1111/ggi.13666
43. Menghini-Muller S, Studerus E, Ittig S, Valmaggia LR, Kempton MJ, van der Gaag M, de Haan L, Nelson B, Bressan RA, Barrantes-Vidal N et al: Sex differences in cognitive functioning of patients at-risk for psychosis and healthy controls: Results from the European Gene-Environment Interactions study. Eur Psychiatry 2020, 63(1):e25. https://dx.doi.org/10.1192/j.eurpsy.2019.10.
44. Toccaceli V, Fagnani C, Eisenberg N, Alessandri G, Vitale A, Stazi MA: Adult Empathy: Possible Gender Differences in Gene-Environment Architecture for Cognitive and Emotional Components in a Large Italian Twin Sample. Twin Res Hum Genet 2018, 21(3):214-226. https://dx.doi.org/10.1017/thg.2018.19.
45. Wu YT, Prina AM, Jones AP, Barnes LE, Matthews FE, Brayne C, Medical Research Council Cognitive F, Ageing S: Community environment, cognitive impairment and dementia in later life: results from the Cognitive Function and Ageing Study. Age Ageing 2015, 44(6):1005-1011. https://dx.doi.org/10.1093/ageing/afv137.
46. Wu YT, Prina AM, Jones A, Matthews FE, Brayne C, Mrc C: Older people, the natural environment and common mental disorders: cross-sectional results from the Cognitive Function and Ageing Study. BMJ Open 2015, 5(9):e007936. https://dx.doi.org/10.1136/bmjopen-2015-007936.
47. Levin OS, Vasenina EE: [Depression and cognitive decline in elderly: causes and consequences]. Zh Nevrol Psikhiatr Im S S Korsakova 2019, 119(7):87-94. https://dx.doi.org/10.17116/jnevro201911907187.
48. Zhou L, Ma X, Wang W: Relationship between Cognitive Performance and Depressive Symptoms in Chinese Older Adults: The China Health and Retirement Longitudinal Study (CHARLS). J Affect Disord 2021, 281:454-458. https://dx.doi.org/10.1016/j.jad.2020.12.059.
49. Calderon-Garciduenas L, Torres-Jardon R, Franco-Lira M, Kulesza R, Gonzalez-Maciel A, Reynoso-Robles R, Brito-Aguilar R, Garcia-Arreola B, Revueltas-Ficachi P, Barrera-Velazquez JA et al: Environmental Nanoparticles, SARS-CoV-2 Brain Involvement, and Potential Acceleration of Alzheimer’s and Parkinson’s Diseases in Young Urbanites Exposed to Air Pollution. J Alzheimers Dis 2020, 78(2):479-503. https://dx.doi.org/10.3233/JAD-200891.
50. Nussbaum R, Lucht S, Jockwitz C, Moebus S, Engel M, Jockel KH, Caspers S, Hoffmann B: Associations of Air Pollution and Noise with Local Brain Structure in a Cohort of Older Adults. Environ Health Perspect 2020, 128(6):67012. https://dx.doi.org/10.1289/EHP5859.
51. Barnett DW, Barnett A, Nathan A, Van Cauwenberg J, Cerin E, Council on E, Physical Activity – Older Adults working g: Built environmental correlates of older adults’ total physical activity and walking: a systematic review and meta-analysis. Int J Behav Nutr Phys Act 2017, 14(1):103. https://doi.org/10.1186/s12966-017-0558-z.
52. Cherrie MPC, Shortt NK, Thompson CW, Deary IJ, Pearce JR: CM-200717-4049023 Association Between the Activity Space Exposure to Parks in Childhood and Adolescence and Cognitive Aging in Later Life. Int J Env Res Pub He 2019, 16(4). https://dx.doi.org/ARTN 63210.3390/ijerph16040632.
53. Bancroft C, Joshi S, Rundle A, Hutson M, Chong C, Weiss CC, Genkinger J, Neckerman K, Lovasi G: Association of proximity and density of parks and objectively measured physical activity in the United States: A systematic review. Soc Sci Med 2015, 138:22-30. https://dx.doi.org/10.1016/j.socscimed.2015.05.034.
54. Angevaren M, Aufdemkampe G, Verhaar HJJ, Aleman A, Vanhees L: Physical activity and enhanced fitness to improve cognitive function in older people without known cognitive impairment. Cochrane Db Syst Rev 2008(3). https://dx.doi.org/10.1002/14651858.CD005381.pub3.
55. Angevaren M, Aufdemkampe G, Verhaar HJ, Aleman A, Vanhees L: Physical activity and enhanced fitness to improve cognitive function in older people without known cognitive impairment. Cochrane Database Syst Rev 2008(3):CD005381. https://dx.doi.org/10.1002/14651858.CD005381.pub3.2008(3):CD005381.
56. Sun F, Norman IJ, While AE: Physical activity in older people: a systematic review. BMC Public Health 2013, 13. https://dx.doi.org/Artn 44910.1186/1471-2458-13-449.
57. Gretebeck KA, Sabatini LM, Black DR, Gretebeck RJ: Physical Activity, Functional Ability, and Obesity in Older Adults: A Gender Difference. J Gerontol Nurs 2017, 43(9):38-46. https://dx.doi.org/10.3928/00989134-20170406-03.
58. Tani Y, Suzuki N, Fujiwara T, Hanazato M, Kondo K: Neighborhood Food Environment and Dementia Incidence: the Japan Gerontological Evaluation Study Cohort Survey. Am J Prev Med 2019, 56(3):383-392. https://dx.doi.org/10.1016/j.amepre.2018.10.028.
59. Teeters DA, Moua T, Li G, Kashyap R, Biehl M, Kaur R, Gajic O, Boeve BF, St Louis EK, Petersen RC et al: Mild Cognitive Impairment and Risk of Critical Illness. Crit Care Med 2016, 44(11):2045-2051. https://dx.doi.org/10.1097/ccm.0000000000001842.
60. Shankar A, Hamer M, McMunn A, Steptoe A: Social isolation and loneliness: relationships with cognitive function during 4 years of follow-up in the English Longitudinal Study of Ageing. Psychosom Med 2013, 75(2):161-170. https://dx.doi.org/10.1097/PSY.0b013e31827f09cd