Have you already an account ?
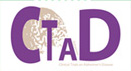
This entitles you to a free 1-year subscription for the online version of JPAD magazine.
Create a new account
Customer account
You need to get a customer account in order to buy single articles from the The Journal of Prevention of Alzheimer's Disease.
Individual Article (Electronic only) 40.00 EUR
Customer account
One year subscription
Register online now and immediately gain access to all articles from the The Journal of Prevention of Alzheimer's Disease.
- Institution 925 EUR (Pharmaceutical industry, library, etc.)
- Individual 385 EUR
One year subscription