C.W. Ritchie1,2,3, J.M.J. Waymont2,4, C. Pennington1,2,5, K. Draper2, A. Borthwick2, N. Fullerton2, M. Chantler6, M.E. Porteous1,3, S.O. Danso1, A. Green1, L. McWhirter1, G. Muniz Terrera1, S. Simpson7, G. Thompson1, D. Trépel8,9, T.J. Quinn7, A. Kilgour1,2
1. University of Edinburgh, 2. Brain Health Scotland, 3. NHS Lothian, 4. University of Aberdeen, 5. NHS Forth Valley, 6. Herriot Watt University, 7. University of Glasgow, 8. University of Dublin Trinity College, 9. Global Brain Health Institute, United Kingdom
Corresponding Author: Prof. Craig Ritchie, University of Edinburgh, United Kingdom, craig.ritchie@ed.ac.uk
Abstract
In order to address the oft-cited societal, economic, and health and social care impacts of neurodegenerative diseases, such as Alzheimer’s disease, we must move decisively from reactive to proactive clinical practice and to embed evidence-based brain health education throughout society. Most disease processes can be at least partially prevented, slowed, or reversed. We have long neglected to intervene in neurodegenerative disease processes, largely due to a misconception that their predominant symptom – cognitive decline – is a normal, age-related process, but also due to a lack of multi-disciplinary collaboration. We now understand that there are modifiable risk factors for neurodegenerative diseases, that successful management of common comorbidities (such as diabetes and hypertension) can reduce the incidence of neurodegenerative disease, and that disease processes begin (and, crucially, can be detected, reduced, and delayed, prevented, or treated) decades earlier in life than had previously been appreciated. Brain Health Scotland, established by Scottish Government and working in partnership with Alzheimer Scotland, propose far-reaching public health and clinical practice approaches to reduce neurodegenerative disease incidence. Focusing here on Brain Health Scotland’s clinical offerings, we present the Scottish Model for Brain Health Services. To our knowledge, the Scottish Model for Brain Health, built on foundations of personalised risk profiling, targeted risk reduction and prevention, early disease detection, equity of access, and harnessing comprehensive data to assist in clinical decision-making, marks the first example of a nationwide approach to overhauling clinical, societal, and political approaches to the prevention, assessment, and treatment of neurodegenerative disease.
Key words: Brain health, neurodegeneration, biomarkers, Alzheimer’s disease, functional cognitive disorders.
Abbreviations: AD: Alzheimer’s Disease; APOE: Apolipoprotein E; BP: Blood pressure; CSF: Cerebrospinal fluid; FCD: Functional Cognitive Disorders; MRI: Magnetic resonance imaging; NFL: Neurofilament light; OR: Odds ratio; PAF: Population-attributable factor; Ptau: Phosphorylated tau; Ttau: Total tau.
Introduction
The clinical, societal, and economic impact of neurodegenerative disease is vast and continues to grow. There are currently estimated to be over 50 million people worldwide living with Alzheimer’s disease (AD), the most common form of neurodegenerative disease giving rise to a clinical dementia syndrome, with the number of people living with AD predicted to double every twenty years (1). While current treatment options lack efficacy in reducing cognitive and functional decline associated with later-stage neurodegenerative disease (likely compounded by diagnosis often not occurring until late-stage disease), prevention and intervention are our best options to mitigate the negative impact of the anticipated rise in neurodegenerative disease prevalence. Age is the greatest risk factor for neurodegenerative disease and clinical dementia syndromes. Where life expectancy grows, so, too, does the urgency to prevent neurodegenerative disease and comorbidities, and to take decisive action to improve brain health at the earliest opportunity, from the population to the individual.
The growing consensus of the need to begin detection, prevention, and intervention earlier in the disease process arose from an accumulation of evidence from three areas: [1] modifiable risk factors for cognitive and functional decline across the life-course (2, 3), [2] evidence that pathophysiological processes underlying neurodegenerative disease begin many years before the emergence of a clinical dementia syndrome (4), and [3] from the recognised need for further development of and preparation for disease-modifying therapies and multimodal interventions for use earlier in the disease process than is currently common practice (5, 6). A recent review in the Journal of Prevention of Alzheimer’s Disease outlines thorough, practical recommendations for early detection of AD in modern clinical practice (7), providing tangible examples of how the presently proposed Scottish Brain Health Service Model may be implemented at the individual level. Further, a recent ‘A User Manual for Brain Health Services’ series (Alzheimer’s Research & Therapy, 2021, vol. 13) evidences the growing consensus of the need to move to preventative, proactive clinical practice, complementing currently existing services available to those in later-stages of neurodegenerative disease (8).
Risk profiling, reduction of modifiable risk factors, and early detection and intervention are standard clinical practice in the management of other diseases, including highly related diseases such as cardiometabolic disease. Clinicians are aware of and routinely assess for indicators of cardiometabolic risk, such as blood pressure (BP) and body mass index (BMI). Secondary prevention and intervention of concurrent conditions, such as type 2 diabetes mellitus and hypercholesterolemia, is routine practice. Risk profiling and increased focus on early disease detection have also been used to develop risk prediction algorithms, which have been successfully implemented to support clinical decision making (e.g., ‘ASSIGN’ score and ‘QRISK’ algorithms for the evaluation of future cardiovascular disease risk (9, 10)). Further, the use of risk profiling, early detection methods, and the development of risk prediction algorithms is already routine in large-scale observational cohort studies of neurodegenerative diseases, particularly of AD. Summaries of notable examples of such studies are presented in Table 1. Additionally, large-scale interdisciplinary research projects, such as the Occitanie Toulouse ‘INSPIRE’ project, continue to explore biomarkers of healthy ageing from animal and human models, with the ambition of identifying a composite biomarker to identify declines in intrinsic capacity (i.e., physiological, mental, and psychological capacity) and functional ability at the earliest possible stage (11). Each of these aforementioned research projects align with the WHO guidelines for Integrated Care for Older People (ICOPE) (12).
Note. CSF: cerebrospinal fluid; AD: Alzheimer’s disease.
The technology and infrastructure exist to integrate risk prediction and early disease detection in research settings, but have not yet been meaningfully translated into clinical practice. There is a clear and pressing need to provide effective brain health care pathways which are distinct from those in place for patients presenting with a clinical dementia syndrome or other presentations indicative of later-stage neurodegenerative disease. Existing ‘memory clinic’ and dementia services are optimised for symptomatic dementia diagnosis and post-diagnostic care. Similarly, while the general public are increasingly aware of neurodegenerative disease and dementia in later life, there remains a pervasive misconception that these diseases and their associated cognitive and functional decline are to be expected in normal ageing (13). Public health approaches to promoting brain health across the life-course are in their infancy. To address the need to move research into practice and public health, Brain Health Scotland was established by Scottish Government in 2020, in partnership with Alzheimer Scotland, to take public health and clinical approaches to reduce incident dementia in Scotland (see Figure 1).
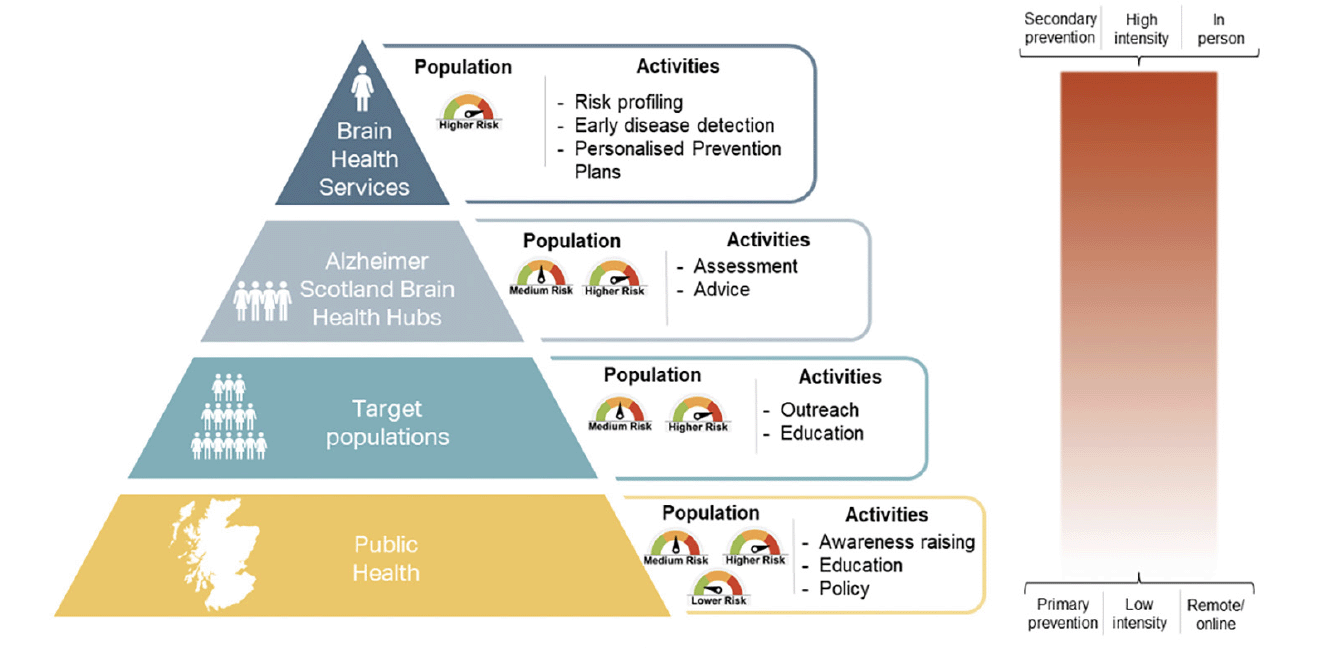
Figure 1. Pyramid of approaches to reduce incident dementia in Scotland, from public health interventions for the Scottish population (bottom tier) to clinical Brain Health Services for the individual (top tier)
The proposed clinical approach of Brain Health Scotland’s brain health services is based on three fundamental principles, which are complementary to but designed to be distinct from the existing ‘memory clinic’ model for later-stage disease: [1] individual risk profiling, [2] early disease detection, and [3] implementation of personalised prevention plans (19). As up to 70% of all cases of a dementia syndrome will have an aetiological contribution from AD pathology (20), the care pathway for brain health services is optimised for, although not monopolised by, the detection of and risk profiling for AD.
The symbiosis between patients, clinicians, community-based clinics, data systems and research facilities forms the foundation of brain health services. Through the integration of these groups and activities, we can generate and continuously update risk prediction algorithms, providing feedback to support clinical decision making and implementation of personalised prevention plans.
Through public health interventions (Figure 1) and brain health services (Figure 2) combined, Brain Health Scotland aims to reduce the incidence of dementia in Scotland, and to ameliorate neurodegenerative disease processes much earlier in their course than is achievable with current approaches. Here, we outline the domains to be assessed and the rationale and scientific basis for them which will allow completion of the first two objectives (risk profiling and early disease detection), with implementation of personalised prevention plans (including proposals for interventions and secondary prevention approaches) to be detailed elsewhere in due course. The following is segmented into sections relating to [1] risk profiling, [2] early disease detection, and [3] general considerations around data systems, equity of access, differential diagnosis, communication, and health economics.
Stage 1: generic, non-clinical support (advice, light-touch lifestyle assessment, information and signposting). Stage 2: initial clinical service (risk profiling, early disease detection, personalised prevention. Parallel referral to external services for management of comorbidities where appropriate). Stage 3: specialised clinical service (brain biomarker assessment, personalised prevention and intervention. Outwards referral to memory clinic for those with an established clinical dementia syndrome unlikely to benefit from continued care in Brain Health Services, parallel referral to external services for comorbidity management where appropriate). SBHR – Scottish Brain Health Register; CSF – cerebrospinal fluid; PDS – Post Diagnostic Support
Risk profiling
Genetic Risk
The apolipoprotein E (APOE) gene is among the most well-documented monogenic risk factors in relation to AD, with the ε4 allele being associated with increased risk of AD and the ε2 allele being a potential protective factor. A recent large neuropathologic study found individuals with ε4/ε4 genotype had an odds ratio (OR) of 11.39 (Confidence Intervals: 9.96-13.02) for developing AD and individuals with the ε2/ε2 genotype having an OR of 0.35 (CI: 0.2-0.61) for developing AD, compared to those with the more common ε3/ε3 genotype, after controlling for age, sex, and autopsy findings (with ORs greater than 1 indicating elevated odds, and ORs below 1 indicating reduced odds) (21). Further, it has been reported that allelic expression is not only related to susceptibility of developing AD, but that carriers of the ε4 allele with AD accrue greater tau accumulation and medial temporal lobe atrophy than those with AD but without the ε4 allele, among other identified heterogeneities, indicating APOE variant confers phenotypic differences in disease progression and clinical expression (22).
Genetic testing is routinely used in determining risk for hereditary disorders (e.g., Huntington’s disease) and in diseases associated with known genetic mutations (e.g., testing for mutations on the BRCA1 and BRCA2 genes associated with breast and ovarian cancers). It is important to note that, in brain health services, genetic testing for the APOE variant will be used for the determination of personal risk (i.e., there is no intention of determining APOE variant as a screening tool, rather as a method of understanding attributable genetic risk). Understanding risk attributable to APOE variant is a crucial component in determining personal risk, and allows for a more accurate determination of the proportion of potentially modifiable risk. Further considerations relating to risk disclosure are discussed later (see Section 5: Risk Disclosure).
Lifestyle risk
Lifestyle, defined by the World Health Organisation as “a way of living based on identifiable patterns of behaviour, which are determined by the interplay between an individual’s personal characteristics, social interactions, and socioeconomic and environmental living conditions”, plays a significant role in brain health and the risk for neurodegenerative disease. The 2020 Lancet Commission on dementia prevention, intervention, and care (3) lists twelve risk factors for dementia across the life-course which, if eradicated at the population level, could reduce worldwide prevalence of dementia by up to 40%. The majority of modifiable risk factors identified in the Lancet 2020 report consist of lifestyle factors or lifestyle-associated comorbidities such as diabetes (discussed further below). Each risk factor identified in the Lancet 2020 report is assigned a population attributable fraction (PAF) indicating the percentage reduction in worldwide dementia prevalence if the risk factor is eliminated entirely. In early life (<45 years), less education is the most important risk factor (PAF = 7%). In midlife (45-65 years), lifestyle risk factors include excessive alcohol consumption (>21 units/week; PAF = 1%), hypertension (PAF = 2%), and obesity (PAF = 1%). Additional factors which may be influenced by lifestyle include hearing loss (PAF = 8%) and traumatic brain injury (PAF = 3%). In later-life (>65 years), significant modifiable lifestyle factors include smoking (PAF = 5%), social isolation (PAF = 4%), physical inactivity (PAF = 2%), and exposure to air pollution across the life-course (PAF = 2%).
These modifiable risk factors, among other lifestyle factors associated with brain health (e.g., diet (23) and sleep (24)), are easily quantifiable through standard clinical measurements (e.g., BP, BMI, glycated haemoglobin) and questionnaires (e.g., physical activity levels, smoker status). Patients’ risk factor data (e.g., total modifiable risk, risk scores for individual factors, combined non-/modifiable risk) will be reassessed and updated at each visit to a Brain Health Service, enabling the clinician and patient to track longitudinal changes in individual risk factors and projected disease trajectory (for those in whom early disease is identified), and to evaluate and re-evaluate the suitability and efficacy of personalised prevention plans.
Medical comorbidities and iatrogenic effects of drugs
Multi-morbidity is increasingly prevalent, yet much of the evidence describing AD risk investigates each risk factor separately. Evidence is now emerging that multimorbidity confers a cumulative risk, with neuropsychiatric and cardiovascular disease, sensory impairment, and cancer all being highlighted as notable risk factors (25). Furthermore, multi-morbidity is associated with polypharmacy (the use of ≥ 5 daily medications), which has been associated with incident dementia (26).
Key medical comorbidities that need to be screened for and, where identified or pre-existing, added to risk prediction algorithms include diabetes (27), atrial fibrillation (28), heart failure (29, 30), hypertension (31), respiratory disease (32), and traumatic brain injury (33, 34).
Anticholinergic medication has been investigated as a potential risk factor for dementia, but the evidence is mixed (35, 36). It may be that higher anticholinergic burden and male sex increases the risk (37). There is also mixed evidence about whether use of benzodiazepines and Z-drugs increase the risk of developing dementia, or whether studies are detecting evidence of reverse causation (i.e., prodromal dementia causing sleep disturbance which led to hypnotic prescription) (36). Moreover, these drugs may drive symptoms of dementia (like confusion) as opposed to actually driving disease processes (e.g., amyloidosis).
Mental health risk
Depression and anxiety have been associated with dementia risk in numerous epidemiological studies with biological plausibility for this association focussing on dysregulation of the hypothalamic-pituitary-adrenal axis (38). Mental health conditions may mediate social isolation and socioeconomic decline and therein the secondary accumulation of other risk factors both medical and lifestyle. The association with mood and anxiety symptoms is also subject to reverse causality as, while these conditions may act as a risk factor earlier in life, they may also act as an early expression of disease. Irrespective of this risk/symptom dilemma, these conditions should be sought for and managed to improve prognosis and well-being.
Modalities for early disease detection and capturing disease expression
Disease detection
Neuroimaging
Neuroimaging methods, including computed tomography (CT), magnetic resonance imaging (MRI), positron emission tomography (PET), and single-photon emission tomography (SPECT) have provided unparalleled insights into in vivo brain health across the life course, and have provided crucial evidence that neurodegenerative pathophysiologic processes begin long before their clinical manifestation as cognitive and functional decline.
Structural brain MRI scans (e.g., T1 MRI sequences) can provide valuable insights into baseline and longitudinal brain volumes, with hippocampal, temporal lobe, and ventricular volumes of particular relevance in early AD detection (39). Fluid attenuated inversion recovery (FLAIR), T2, and T2* (susceptibility-weighted) MR imaging protocols can be used to identify even subtle evidence of vascular disease, including cerebral small vessel disease, which is a highly prevalent brain imaging finding in adults, commonly accompanying neurodegenerative processes and acting as a leading contributor to vascular dementia (40).
PET imaging enables investigation of cortical metabolic function through the use of radiotracers. Of particular importance in the early detection of AD are measures of beta-amyloid (aβ) burden (e.g., PiB) and Tau burden (e.g., T807), both of which have been demonstrated to improve diagnostic confidence (41–43). PET imaging of cerebral glucose metabolism (e.g., Fluorodeoxyglucose-PET) is also widely used to assess hypometabolism, which is indicative of neurodegenerative disease more broadly (44).
These imaging methods are routinely included in clinical and research neuroimaging practice and there exist numerous medical image analysis pipelines for the quantification of neurodegenerative disease processes which provide clear, interpretable information on brain health, which can also be incorporated into risk prediction algorithms.
Cerebrospinal fluid and blood biomarkers
Cerebrospinal fluid (CSF) biomarkers for aiding in early AD detection have advanced considerably in recent years. There is significant evidence in favour of using CSF levels of phosphorylated tau (Ptau), total tau (Ttau), and neurofilament light (NFL) as biomarkers for preclinical AD detection (45). In isolation of other risk factor analysis, CSF biomarkers yield reasonable sensitivity but poor specificity for predicting progression from pre-clinical AD to a clinical dementia syndrome, highlighting the need to embed such biomarker assessments in multifaceted risk algorithms (46–48). The extraction of CSF through lumbar puncture (LP) is an invasive procedure and will not be suitable for all patients, but its benefits should not be overlooked.
Blood biomarkers, which are less invasive to obtain than CSF biomarkers and more accessible than CSF or neuroimaging biomarkers, are currently less widely accepted than CSF or imaging markers as being clinically effective. Blood biomarkers under particular scrutiny include plasma and serum NFL level, plasma aβ42/40 ratio, and plasma Ptau and Ttau levels. Zetterberg and colleagues (49) note that while plasma and serum NFL are good predictors of AD, they are not disease-specific. Similarly, the authors comment that aβ42/40 ratio is a strong predictor of brain amyloid burden, but this is also not specific to AD (and may be more indicative of amyloidosis, which is a prevalent feature of brain ageing). Plasma Ptau and Ttau levels are put forward as promising, AD-specific measurements, but they require further development and validation (49).
Additional and emerging biomarkers
Brain health services will provide a natural environment for the development and validation of further biomarkers and measures of early disease progress. The symbiosis of clinical and research activities will allow for efficient integration of well-established research methods and disease detection (such as electroencephalography – EEG (50)) and novel and emerging approaches (such as artificial intelligence in speech and language processing (51)) into clinical practice. This symbiosis is a key driver of the Scottish Brain Health and Dementia Research Strategy, launched in July 2021 (52).
Disease expression
We anticipate that the majority of patients accessing brain health services will not present with symptoms associated with later-stage disease (i.e., significant cognitive and functional decline). Cognitive and functional measures used in later-stage neurodegenerative disease will not necessarily be appropriate or suitably sensitive for our purposes. Here, we describe aspects of early disease expression, which are often subtle and for which further development of prodromal disease assessment methods are required.
Cognitive
Cognitive changes detectable in preclinical AD include poorer performance in tasks involving visuospatial processing, assessable through measures such as the Four Mountains test, which is sensitive to changes in allocentric spatial processing and indicative of change to hippocampal structure beginning in midlife (53, 54). When assessing cognitive performance at any stage of the disease-course, other factors potentially contributing to poorer performance on these tests should be considered (e.g., less education, anxiety, delirium). The transition proposed from considering neurodegenerative diseases as brain diseases with cognitive symptoms, rather than ‘cognitive disorders’, is critical to the development of brain health services. It is likely that many, if not the majority, accessing brain health services will have no cognitive symptoms observable using traditional measures such as the Mini Mental State Examination (MMSE) or Clinical Dementia Rating (CDR), which are prone to ceiling effects and are optimised for populations with overt clinical symptoms.
Behavioural and neuropsychiatric
Although sleep was not included in the 2020 Lancet Commission Report (3), there is clear and consistent evidence linking poor quality sleep with risk of cognitive decline (55). The brain in sleep is considered to be an optimised state for clearance of toxic proteins and other waste products via the glymphatic system, highlighting the potential association between poor sleep and amyloidosis (56), and is likely a symptom of neurodegenerative disease, demonstrating a bidirectional relationship similar to that of depression and cognitive decline.
Apathy has also been associated as an early symptom of neurodegenerative disease (including Parkinson’s disease (57)) and a predictor of conversion from ‘Mild Cognitive Impairment’ (MCI, early clinical disease phase) to an established dementia syndrome (later clinical disease phase) (58, 59). Collectively, neuropsychiatric and behavioural symptoms have been collected into the concept of Mild Behavioural Impairment (MBI), which is considered to be a separate and perhaps earlier indicator of neurodegeneration, worthy of inclusion in Brain Health Service assessment (60).
Gait/power and autonomic instability
Gait speed is known to reach a peak in the third decade and decline thereafter, not becoming apparent until older age (61). Slower gait speed has been found to predict incident dementia by up to seven years in older adults (62). Even in midlife, gait speed has been shown to be associated with white matter hyperintensity accumulation and cognitive decline (63). While a single measure of gait speed could be used as a predictive tool for neurodegenerative disease risk, longitudinal measurements are likely to be more useful. Similarly, weaker grip strength in midlife is associated with poorer cognitive ability, and several studies have found an association between declining grip strength in older adults and the risk of incipient dementia (64).
Autonomic dysfunction can feature as a part of any neurodegenerative disease, most commonly in Lewy body disorders, while evidence of autonomic dysfunction prevalence in AD and vascular dementia is conflicting (65). There is evidence of sympathetic dysfunction and cognitive impairment in older adults without an apparent clinical dementia syndrome (66), indicating that detection of autonomic dysfunction in older patients without dementia could represent early disease expression of neurodegenerative disorders.
Analytical approaches and data use
Risk algorithms
An individual’s risk for neurodegenerative disease can be estimated through the use of prediction algorithms and machine learning to support clinical decision making. Examples of automated or semi-automated risk prediction methods routinely used in other areas of clinical practice include the ASSIGN cardiovascular risk assessment tool (10), which is used in primary care in Scotland to estimate ten-year risk of adverse cardiovascular events in asymptomatic individuals with no clinical evidence of cardiovascular disease, and the QCancer ten-year risk tool (67) which calculates the absolute risk of an asymptomatic individual having a yet undiagnosed cancer. These online tools are only as accurate as their source data and, as such, are updated on a regular basis to reflect newly identified risk factors and changing population characteristics. Linkage of patient data between hospital and GP records and centrally held information (e.g., death certification) will allow more accurate projections than relying on information from fewer sources. The veracity of the data being collected to deliver risk prediction algorithms for the onset or progression of neurodegenerative diseases is a fundamental factor and drives the comprehensive range of data being collected, as above, on risk factors, biomarkers, and expression of disease. The data pathway will be honed over the first few years of operation, but we initially intend to include all data collected in Brain Health Services (risk factors, biomarkers, disease expression) and relevant data made available through data linkage derived from patients’ interactions with NHS services. The quantity and exact nature of data required will alter over time as the algorithm(s) and developers identify the core minimum dataset required for accurate prediction of disease progression. Output from the risk prediction and disease progression algorithm(s) will be fed back to clinicians in Brain Health Services to act as a support tool in diagnosis and disease management.
Secondary research environment
As alluded to above, brain health services will generate a vast amount of data, particularly around early- and mid-life factors associated with neurodegenerative disease. Secondary analyses of data naturally and routinely generated within brain health services, with the explicit consent of clinic patients (and anonymised to adhere with data security protocols), will provide highly valuable epidemiology data enabling further understanding of the origins of neurodegenerative diseases and enabling assessment of the efficacy of treatments and interventions in this naturally occurring Phase IV setting. Moreover, highly characterised patients, where willing, can be offered opportunities to enter clinical trials, where low screen failure rates can be anticipated – a key issue affecting trial delivery in AD. Clinically-based recruitment has been shown to be far more effective than population, register-based recruitment (68).
Differential diagnosis
While brain health services will be optimised for the early detection of neurodegenerative disease, we will inevitably detect functional and cognitive changes in patients which are attributable to other disease processes (e.g., infection, mental illness, delirium), and we will uncover incidental findings in patients who appear otherwise healthy (e.g., an incidental neuroimaging finding of a tumour). In cases where disease processes or incidental findings suggest no concurrent neurodegenerative disease and specialist services are required (e.g., oncology, neurosurgery), patients will be referred out of brain health services to the appropriate service. There may be other circumstances in which it is appropriate for brain health services to support a patient through referral into other services while maintaining their involvement in brain health service pathways (e.g., where a patient has increased risk or early indicators of neurodegenerative disease and a comorbidity requiring additional, specialist treatment).
We are working on developing a Red/Amber/Green (RAG) guideline list of conditions to aid decision making that will be subject to change as the pathway develops on the basis of experience and multi-disciplinary discussions (see Figure 3). Red conditions are those where management takes place entirely outside a Brain Health Service (e.g., brain tumour, significant mental illness including suicidal ideation and psychosis). Amber conditions are those where management may be shared with other clinical services (e.g., alcohol use disorders, Multiple Sclerosis, Motor Neurone Disease). Green conditions are neurodegenerative and cerebrovascular diseases. As services develop (both within and without the Brain Health Service), this RAG categorisation will be subject to review and change.
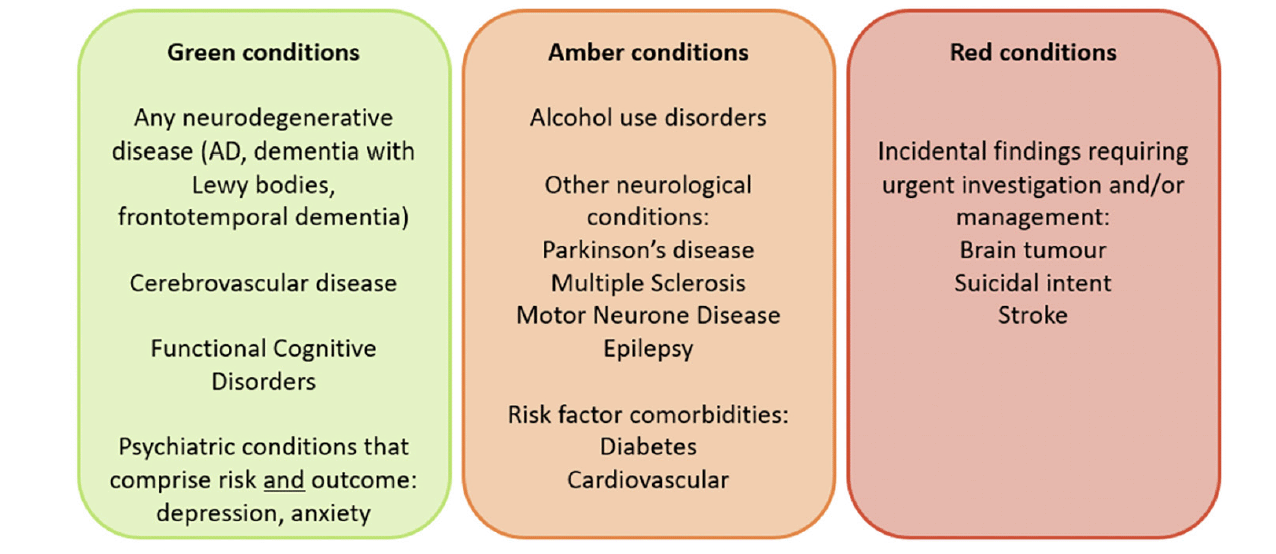
Figure 3. Red/Amber/Green (RAG) chart providing examples of which conditions would be treated within brain health services (green), conditions which would be managed within brain health services alongside additional specialist care (amber), and conditions which would be referred out of brain health services (red)
Functional Cognitive Disorders
Functional cognitive disorders (FCD) are conditions in which cognitive symptoms are experienced as the result of internally-inconsistent changes in attentional functions and metacognition, and not as the result of neurodegenerative disease or structural brain injury (69). They account for up to one in four memory clinic presentations, but are more frequent in attendees who have self-referred or are younger than 65 years of age (70). Sometimes unhelpfully described as the ‘worried well’, people with FCD, in contrast, have more anxiety, depression, poor sleep, and somatic symptoms than those with other causes of cognitive symptoms (70) (and McWhirter et al., 2021, under review). Clinical examination integrating consideration of linguistic and interactive features can support a positive diagnosis of FCD (71). Ongoing research aims to better understand longitudinal FCD trajectories, mechanisms, and to develop effective and scalable interventions. Low level psychological intervention alongside personal risk reductions plans may be appropriate in many cases; in others, specialist psychological or psychiatric follow-up may be required.
Risk disclosure
In developing individual risk profiles, careful consideration will need to be given to the impact of communicating the results to the recipient. A systematic review of the psychological, behavioural, and social effects of disclosing AD biomarker status found there was no increase in depression or anxiety in those to whom it was disclosed that they were carriers of the APOE ε4 variant (72). However, there was an uptake in long-term care insurance and positive health-related behaviour compared to those who tested negative for the ε4 allele. Nearly all of the subjects of studies reported in this review were first-degree relatives of someone with AD, therefore any detrimental impact of genetic testing may be mediated by the individual already perceiving themselves to be at increased risk.
In brain health services, patients will be encouraged to play an active role in the risk disclosure process. Patients will be made aware of what each test they undertake could indicate, and of the immediate and longer-term implications of test results. Ultimately, patients should be able to choose how much information they receive and how much remains known to the clinicians but is not disclosed to the patient. For example, a patient may wish to know the results of brain imaging, but may not wish to know whether they are an APOE ε4 carrier. Patients’ wishes should be accommodated, and patients should be provided with the best information available for them to decide their preferred extent of risk disclosure and testing.
Further, all patients will be provided with a personalised risk prevention and reduction plan. Again, this will be developed in partnership with the patient and be reflective of their own personal goals (73). When disclosing risk to a patient, emphasis should be placed on modifiable risk and on the positive actions that can be taken to reduce risk over time. Patients should be reassured that they will be offered follow-up appointments and will be supported in implementing their risk reduction and prevention plans. As stated previously, the rationale and implementation of personalised prevention plans and secondary prevention and intervention approaches in Brain Health Scotland’s clinical services will be elaborated upon in future publications.
Health economics
In moving towards prevention and early intervention of neurodegenerative disease, ultimately empowering individuals to live a greater proportion of life in better health, it is possible that we can alleviate some of the economic burden arising from a growing proportion of the population requiring advanced care. This is demonstrated in research indicating the increasing costs of dementia care over time (74), and in studies suggesting that efforts to prevent or intervene in specific risk factors offer the most cost-effective approach to dementia care (75). Of particular note is a recent study which found that NHS in England would save £1.863 billion per year (based on 2012/13 prices) in formal (health and social) and informal care with the successful implementation of interventions for hearing loss, hypertension, and smoking (76). Data collection to feed into ongoing health and economic analyses and reporting will form a key part of our brain health services.
Equity of access
Equity of access is a fundamental principle underpinning all of Brain Health Scotland’s activities. Many of the risk factors associated with poorer brain health accumulate and are exacerbated within underrepresented populations, and too many underserved members of the population struggle to access healthcare. In Scotland, there are also vast differences in accessibility to health services for those in rural and remote areas compared with those in better-connected towns and cities. It is hoped that through increasing accessibility of services, we can improve access to research programmes, enabling the science our clinics is based on to better represent the populations we serve. Further information on our approach to equity of access will be presented elsewhere, but includes co-design of services with marginalised communities, provision of mobile/roving brain health services, digital and telehealth services, and active and ongoing evaluation of the impact of our services within underrepresented groups.
Demonstrator sites
‘Demonstrator sites’ – Brain Health Scotland’s clinical services, cultivated to provide models of best practice and as hubs for supporting continued professional development – are currently being identified and established in partnership with Scottish Government, NHS Scotland, and Alzheimer Scotland. Brain health services will routinely undergo quality improvement assessments, with data from these exercises in initial demonstrator sites to provide insights for further development before a wider roll-out of brain health services for Scotland. This national availability is in the Scottish Programme for Government, to be completed by 2025.
Conclusions
It is time for research evidence to be transferred into routine clinical practice. Evidence that neurodegenerative disease processes begin many years before disease expression, particularly in diseases that give rise to a clinical dementia syndrome, is widely accepted, and methods to detect neurodegenerative disease and to reduce risk factors at the earliest opportunity are ready for clinical implementation. This is of particular importance for patients who currently fall through the gaps, such as those who may have early clinical symptoms, but their case is not severe enough to warrant referral to or ongoing engagement with memory clinics or specialist dementia care providers. Through the combined effort of brain health clinicians and academic research programmes in Scotland, we are well positioned to implement the Scottish Model of Brain Health clinical services for early disease detection, intervention, and personalised prevention, and will soon be prepared to demonstrate the impact these brain health services can have on reducing the incidence of late-stage neurodegenerative disease in the Scottish population.
Funding: Brain Health Scotland, who are overseeing the development of Brain Health Services within NHS Scotland, are funded through a grant from the Scottish Government. Brain Health Scotland is hosted legally within Alzheimer Scotland who received this grant.
Conflicts of Interest: CWR is the director of Brain Health Scotland and receives consultancy fees for this role. JMJW, AB, and NF are employed by Brain Health Scotland (Alzheimer Scotland). The following authors wish to declare additional conflicts of interest and funding received for work unrelated to the present manuscript: CWR has received consultancy fees (Biogen, Eisai, MSD, Actinogen, Roche, Eli Lilly), speaker fees (Eisai, Roche), sits on an NIHR data safety monitoring board (DSMB), and is the unpaid chair of the Brain Health Clinic Consortium and the Scottish Dementia Research Consortium. JMJW received salary support through an education grant provided to Brain Health Scotland from Biogen, and has previously received studentship funding from TauRx Pharmaceuticals. CP receives funding from the Scottish Government’s Chief Scientist Office through a Career Researcher Fellowship. LM received funding from Baillie Gifford, currently receives grant/contract funding from the Scottish Government Chief Scientist Office, is a director of a limited personal services company providing independent medical testimony in court cases, sits on the editorial board of the British Journal of Psychiatry, and on the board of directors of the British Neuropsychiatry Association. GT has received consulting fees from QMENTA. DT has received grant/contract funding from the Public Health Agency Northern Ireland, the Irish Research Council, the Health Research Board, HealthLat, the National Health and Medical Research Council, and the Global Brain Health Institute. TJQ has received grant/contract funding from NIHR, Scottish Government’s Chief Scientist Office, ESPRC, Stroke Association, Dunhill Medical, and sits on the DSMB for Novartis. The remaining authors (MEP, SOD, SS, and AK) have no funding or conflicts of interest to declare.
Ethical standard: The present manuscript is a review of the literature and proposal for a novel care pathway – this required no ethics review.
Open Access: This article is distributed under the terms of the Creative Commons Attribution 4.0 International License (http://creativecommons.org/licenses/by/4.0/), which permits use, duplication, adaptation, distribution and reproduction in any medium or format, as long as you give appropriate credit to the original author(s) and the source, provide a link to the Creative Commons license and indicate if changes were made.
References
1. Prince M, Wimo A, Guerchet M, et al. World Alzheimer Report 2015 The Global Impact of Dementia An AnalysIs of Prevalence, Incidence, Cost and Trends.; 2015. www.alz.co.uk/worldreport2015corrections. Accessed January 25, 2021.
2. Livingston G, Sommerlad A, Orgeta V, et al. Dementia prevention, intervention, and care. Lancet. 2017;390(10113):2673-2734. doi:10.1016/S0140-6736(17)31363-6
3. Livingston G, Huntley J, Sommerlad A, et al. Dementia prevention, intervention, and care: 2020 report of the Lancet Commission. Lancet. 2020;396(10248):413-446. doi:10.1016/S0140-6736(20)30367-6
4. Sperling RA, Aisen PS, Beckett LA, et al. Toward defining the preclinical stages of Alzheimer’s disease: Recommendations from the National Institute on Aging-Alzheimer’s Association workgroups on diagnostic guidelines for Alzheimer’s disease. Alzheimer’s Dement. 2011;7(3):280-292. doi:10.1016/j.jalz.2011.03.003
5. Ritchie CW, Russ TC, Banerjee S, et al. The Edinburgh Consensus: Preparing for the advent of disease-modifying therapies for Alzheimer’s disease. Alzheimer’s Res Ther. 2017;9(1):1-7. doi:10.1186/s13195-017-0312-4
6. Yu JT, Xu W, Tan CC, et al. Evidence-based prevention of Alzheimer’s disease: Systematic review and meta-analysis of 243 observational prospective studies and 153 randomised controlled trials. J Neurol Neurosurg Psychiatry. 2020;91(11):1201-1209. doi:10.1136/jnnp-2019-321913
7. Porsteinsson A, Isaacson R, Knox S, Sabbagh M, Rubino I. Diagnosis of Early Alzheimer’s Disease: Clinical Practice in 2021. J Prev Alzheimer’s Dis. 2021;8(3). doi:10.14283/JPAD.2021.23
8. Altomare D, Molinuevo JL, Ritchie C, et al. Brain Health Services: organization, structure, and challenges for implementation. A user manual for Brain Health Services—part 1 of 6. Alzheimer’s Res Ther 2021 131. 2021;13(1):1-11. doi:10.1186/S13195-021-00827-2
9. Hippisley-Cox J, Coupland C, Vinogradova Y, Robson J, May M, Brindle P. Derivation and validation of QRISK, a new cardiovascular disease risk score for the United Kingdom: Prospective open cohort study. Br Med J. 2007;335(7611):136-141. doi:10.1136/bmj.39261.471806.55
10. Tunstall-Pedoe H. Cardiovascular risk and risk scores: ASSIGN, Framingham, QRISK and others: How to choose. Heart. 2011;97(6):442-444. doi:10.1136/hrt.2010.214858
11. Ader I, Pénicaud L, Andrieu S, et al. Healthy Aging Biomarkers: The INSPIRE’s Contribution. J frailty aging. 2021;10(4):313-319. doi:10.14283/JFA.2021.15
12. WHO. Integrated care for older people: guidelines on community-level interventions to manage declines in intrinsic capacity. https://www.who.int/publications/i/item/9789241550109. Accessed November 4, 2021.
13. Cations M, Radisic G, Crotty M, Laver KE. What does the general public understand about prevention and treatment of dementia? A systematic review of population-based surveys. Werner P, ed. PLoS One. 2018;13(4):e0196085. doi:10.1371/journal.pone.0196085
14. Bateman RJ, Xiong C, Benzinger TLS, et al. Clinical and Biomarker Changes in Dominantly Inherited Alzheimer’s Disease. N Engl J Med. 2012;367(9):795-804. doi:10.1056/NEJMoa1202753
15. Bateman RJ, Benzinger TL, Berry S, et al. The DIAN-TU Next Generation Alzheimer’s prevention trial: Adaptive design and disease progression model. Alzheimer’s Dement. 2017;13(1):8-19. doi:10.1016/j.jalz.2016.07.005
16. Ellis KA, Bush AI, Darby D, et al. The Australian Imaging, Biomarkers and Lifestyle (AIBL) study of aging: Methodology and baseline characteristics of 1112 individuals recruited for a longitudinal study of Alzheimer’s disease. Int Psychogeriatrics. 2009;21(4):672-687. doi:10.1017/S1041610209009405
17. Ritchie CW, Ritchie K. The PREVENT study: A prospective cohort study to identify mid-life biomarkers of late-onset Alzheimer’s disease. BMJ Open. 2012;2(6):1893. doi:10.1136/bmjopen-2012-001893
18. Ritchie CW, Molinuevo JL, Truyen L, Satlin A, Van der Geyten S, Lovestone S. Development of interventions for the secondary prevention of Alzheimer’s dementia: The European Prevention of Alzheimer’s Dementia (EPAD) project. The Lancet Psychiatry. 2016;3(2):179-186. doi:10.1016/S2215-0366(15)00454-X
19. Frisoni GB, Molinuevo JL, Altomare D, et al. Precision prevention of Alzheimer’s and other dementias: Anticipating future needs in the control of risk factors and implementation of disease-modifying therapies. Alzheimer’s Dement. 2020;16(10):1457-1468. doi:10.1002/alz.12132
20. Sosa-Ortiz AL, Acosta-Castillo I, Prince MJ. Epidemiology of Dementias and Alzheimer’s Disease. Arch Med Res. 2012;43(8):600-608. doi:10.1016/j.arcmed.2012.11.003
21. Reiman EM, Arboleda-Velasquez JF, Quiroz YT, et al. Exceptionally low likelihood of Alzheimer’s dementia in APOE2 homozygotes from a 5,000-person neuropathological study. Nat Commun. 2020;11(1):1-11. doi:10.1038/s41467-019-14279-8
22. Emrani S, Arain HA, DeMarshall C, Nuriel T. APOE4 is associated with cognitive and pathological heterogeneity in patients with Alzheimer’s disease: a systematic review. Alzheimer’s Res Ther 2020 121. 2020;12(1):1-19. doi:10.1186/S13195-020-00712-4
23. Scarmeas N, Stern Y, Tang M-X, Mayeux R, Luchsinger JA. Mediterranean diet and risk for Alzheimer’s disease. Ann Neurol. 2006;59(6):912-921. doi:10.1002/ana.20854
24. Minakawa E, Wada K, Nagai Y. Sleep Disturbance as a Potential Modifiable Risk Factor for Alzheimer’s Disease. Int J Mol Sci. 2019;20(4):803. doi:10.3390/ijms20040803
25. Grande G, Marengoni A, Vetrano DL, et al. Multimorbidity burden and dementia risk in older adults: The role of inflammation and genetics. Alzheimer’s Dement. January 2021:alz.12237. doi:10.1002/alz.12237
26. Park H-Y, Park J-W, Song HJ, Sohn HS, Kwon J-W. The Association between Polypharmacy and Dementia: A Nested Case-Control Study Based on a 12-Year Longitudinal Cohort Database in South Korea. Laks J, ed. PLoS One. 2017;12(1):e0169463. doi:10.1371/journal.pone.0169463
27. Biessels GJ, Staekenborg S, Brunner E, Brayne C, Scheltens P. Risk of dementia in diabetes mellitus: A systematic review. Lancet Neurol. 2006;5(1):64-74. doi:10.1016/S1474-4422(05)70284-2
28. Kwok CS, Loke YK, Hale R, Potter JF, Myint PK. Atrial fibrillation and incidence of dementia: A systematic review and meta-analysis. Neurology. 2011;76(10):914-922. doi:10.1212/WNL.0b013e31820f2e38
29. Eggermont LHP, De Boer K, Muller M, Jaschke AC, Kamp O, Scherder EJA. Cardiac disease and cognitive impairment: A systematic review. Heart. 2012;98(18):1334-1340. doi:10.1136/heartjnl-2012-301682
30. Wolters FJ, Segufa RA, Darweesh SKL, et al. Coronary heart disease, heart failure, and the risk of dementia: A systematic review and meta-analysis. Alzheimer’s Dement. 2018;14(11):1493-1504. doi:10.1016/j.jalz.2018.01.007
31. Sharp SI, Aarsland D, Day S, Sønnesyn H, Ballard C. Hypertension is a potential risk factor for vascular dementia: systematic review. Int J Geriatr Psychiatry. 2011;26(7):661-669. doi:10.1002/gps.2572
32. Russ TC, Kivimäki M, Batty GD. Respiratory Disease and Lower Pulmonary Function as Risk Factors for Dementia: A Systematic Review With Meta-analysis. In: Chest. Vol 157. Elsevier Inc; 2020:1538-1558. doi:10.1016/j.chest.2019.12.012
33. Gardner RC, Burke JF, Nettiksimmons J, Kaup A, Barnes DE, Yaffe K. Dementia risk after traumatic brain injury vs nonbrain trauma: The role of age and severity. JAMA Neurol. 2014;71(12):1490-1497. doi:10.1001/jamaneurol.2014.2668
34. Godbolt AK, Cancelliere C, Hincapié CA, et al. Systematic review of the risk of dementia and chronic cognitive impairment after mild traumatic brain injury: Results of the international collaboration on mild traumatic brain injury prognosis. Arch Phys Med Rehabil. 2014;95(3 SUPPL):S245-S256. doi:10.1016/j.apmr.2013.06.036
35. Grossi CM, Richardson K, Fox C, et al. Anticholinergic and benzodiazepine medication use and risk of incident dementia: A UK cohort study. BMC Geriatr. 2019;19(1):276. doi:10.1186/s12877-019-1280-2
36. Hafdi M, Hoevenaar-Blom MP, Beishuizen CRL, Moll van Charante EP, Richard E, van Gool WA. Association of Benzodiazepine and Anticholinergic Drug Usage With Incident Dementia: A Prospective Cohort Study of Community-Dwelling Older Adults. J Am Med Dir Assoc. 2020;21(2):188-193.e3. doi:10.1016/j.jamda.2019.05.010
37. Liu YP, Chien WC, Chung CH, Chang HA, Kao YC, Tzeng NS. Are Anticholinergic Medications Associated With Increased Risk of Dementia and Behavioral and Psychological Symptoms of Dementia? A Nationwide 15-Year Follow-Up Cohort Study in Taiwan. Front Pharmacol. 2020;11:1. doi:10.3389/fphar.2020.00030
38. Smith SM, Vale WW. The role of the hypothalamic-pituitary-adrenal axis in neuroendocrine responses to stress. Dialogues Clin Neurosci. 2006;8(4):383. doi:10.31887/DCNS.2006.8.4/SSMITH
39. Scheltens P, Fox N, Barkhof F, De Carli C. Structural magnetic resonance imaging in the practical assessment of dementia: Beyond exclusion. Lancet Neurol. 2002;1(1):13-21. doi:10.1016/S1474-4422(02)00002-9
40. Wardlaw JM, Smith EE, Biessels GJ, et al. Neuroimaging standards for research into small vessel disease and its contribution to ageing and neurodegeneration. Lancet Neurol. 2013;12(8):822-838. doi:10.1016/S1474-4422(13)70124-8
41. Altomare D, Caprioglio C, Assal F, et al. Diagnostic value of amyloid-PET and tau-PET: a head-to-head comparison. Eur J Nucl Med Mol Imaging. February 2021:1-12. doi:10.1007/s00259-021-05246-x
42. Villemagne VL, Doré V, Bourgeat P, et al. Aβ-amyloid and Tau Imaging in Dementia. Semin Nucl Med. 2017;47(1):75-88. doi:10.1053/j.semnuclmed.2016.09.006
43. Villemagne VL, Doré V, Burnham SC, Masters CL, Rowe CC. Imaging tau and amyloid-? proteinopathies in Alzheimer disease and other conditions. Nat Rev Neurol. 2018;14(4):225-236. doi:10.1038/nrneurol.2018.9
44. Dave A, Hansen N, Downey R, Johnson C. FDG-PET Imaging of Dementia and Neurodegenerative Disease. Semin Ultrasound, CT MRI. 2020;41(6):562-571. doi:10.1053/j.sult.2020.08.010
45. Olsson B, Lautner R, Andreasson U, et al. CSF and blood biomarkers for the diagnosis of Alzheimer’s disease: a systematic review and meta-analysis. Lancet Neurol. 2016;15(7):673-684. doi:10.1016/S1474-4422(16)00070-3
46. Kokkinou M, Beishon LC, Smailagic N, et al. Plasma and cerebrospinal fluid ABeta42 for the differential diagnosis of Alzheimer’s disease dementia in participants diagnosed with any dementia subtype in a specialist care setting. Cochrane Database Syst Rev. 2021;2. doi:10.1002/14651858.cd010945.pub2
47. Noel-Storr AH, Flicker L, Ritchie CW, et al. Systematic review of the body of evidence for the use of biomarkers in the diagnosis of dementia. Alzheimer’s Dement. 2013;9(3):e96-e105. doi:10.1016/j.jalz.2012.01.014
48. Ritchie C, Smailagic N, Noel-Storr AH, et al. Plasma and cerebrospinal fluid amyloid beta for the diagnosis of Alzheimer’s disease dementia and other dementias in people with mild cognitive impairment (MCI). Cochrane Database Syst Rev. 2014;2014(6):CD008782-CD008782. doi:10.1002/14651858.CD008782.pub4
49. Zetterberg H. Blood-based biomarkers for Alzheimer’s disease—An update. J Neurosci Methods. 2019;319:2-6. doi:10.1016/j.jneumeth.2018.10.025
50. Rossini PM, Di Iorio R, Vecchio F, et al. Early diagnosis of Alzheimer’s disease: the role of biomarkers including advanced EEG signal analysis. Report from the IFCN-sponsored panel of experts. Clin Neurophysiol. 2020;131(6):1287-1310. doi:10.1016/j.clinph.2020.03.003
51. De La Fuente Garcia S, Ritchie CW, Luz S. Artificial Intelligence, Speech, and Language Processing Approaches to Monitoring Alzheimer’s Disease: A Systematic Review. J Alzheimer’s Dis. 2020;78(4):1547-1574. doi:10.3233/JAD-200888
52. Scottish Dementia Research Consortium; Alzheimer Scotland; Brain Health Scotland. Scottish Brain Health and Dementia Research Strategy. https://www.sdrc.scot/wp-content/uploads/2021/07/Scottish-Brain-Health-Dementia-Research-Strategy-2021-min-1-1.pdf. Published July 2021. Accessed September 6, 2021.
53. Dounavi ME, Mak E, Wells K, et al. Volumetric alterations in the hippocampal subfields of subjects at increased risk of dementia. Neurobiol Aging. 2020;91:36-44. doi:10.1016/J.NEUROBIOLAGING.2020.03.006
54. Ritchie K, Carrière I, Howett D, et al. Allocentric and Egocentric Spatial Processing in Middle-Aged Adults at High Risk of Late-Onset Alzheimer’s Disease: The PREVENT Dementia Study. J Alzheimer’s Dis. 2018;65(3):885-896. doi:10.3233/JAD-180432
55. Xu W, Tan chen-chen, Zou J-J, cao X-P, Tan lan, department neurology. Sleep problems and risk of all-cause cognitive decline or dementia: an updated systematic review and meta-analysis. J Neurol Neurosurg Psychiatry. 2020;91:236-244. doi:10.1136/jnnp-2019-321896
56. Rasmussen M, Mestre H, Nedergaard M. The glymphatic pathway in neurological disorders. Lancet Neurol. 2018;17(11):1016-1024. doi:10.1016/S1474-4422(18)30318-1
57. Dujardin K, Sockeel P, Delliaux M, Destée A, Defebvre L. Apathy may herald cognitive decline and dementia in Parkinson’s disease. Mov Disord. 2009;24(16):2391-2397. doi:10.1002/MDS.22843
58. Robert PH, Berr C, Volteau M, et al. Apathy in patients with mild cognitive impairment and the risk of developing dementia of Alzheimer’s disease: A one-year follow-up study. Clin Neurol Neurosurg. 2006;108(8):733-736. doi:10.1016/J.CLINEURO.2006.02.003
59. Richard E, Schmand B, Eikelenboom P, et al. Symptoms of Apathy Are Associated with Progression from Mild Cognitive Impairment to Alzheimer’s Disease in Non-Depressed Subjects. Dement Geriatr Cogn Disord. 2012;33(2-3):204-209. doi:10.1159/000338239
60. Ismail Z, Agüera-Ortiz L, Brodaty H, et al. The Mild Behavioral Impairment Checklist (MBI-C): A rating scale for neuropsychiatric symptoms in pre-dementia populations. J Alzheimers Dis. 2017;56(3):929. doi:10.3233/JAD-160979
61. Cruz-Jentoft AJ, Bahat G, Bauer J, et al. Sarcopenia: Revised European consensus on definition and diagnosis. Age Ageing. 2019;48(1):16-31. doi:10.1093/ageing/afy169
62. Dumurgier J, Artaud F, Touraine C, et al. Gait Speed and Decline in Gait Speed as Predictors of Incident Dementia. Journals Gerontol Ser A Biol Sci Med Sci. 2016;72(5):glw110. doi:10.1093/gerona/glw110
63. Rasmussen LJH, Caspi A, Ambler A, et al. Association of Neurocognitive and Physical Function With Gait Speed in Midlife. JAMA Netw open. 2019;2(10):e1913123. doi:10.1001/jamanetworkopen.2019.13123
64. Adamo DE, Anderson T, Koochaki M, Fritz NE. Declines in grip strength may indicate early changes in cognition in healthy middle-aged adults. Wylie GR, ed. PLoS One. 2020;15(4):e0232021. doi:10.1371/journal.pone.0232021
65. Allan LM. Diagnosis and Management of Autonomic Dysfunction in Dementia Syndromes. Curr Treat Options Neurol. 2019;21(8):1-11. doi:10.1007/s11940-019-0581-2
66. Dalise AM, Prestano R, Fasano R, Gambardella A, Barbieri M, Rizzo MR. Autonomic Nervous System and Cognitive Impairment in Older Patients: Evidence From Long-Term Heart Rate Variability in Real-Life Setting. Front Aging Neurosci. 2020;12. doi:10.3389/fnagi.2020.00040
67. Usher-Smith J, Emery J, Hamilton W, Griffin SJ, Walter FM. Risk prediction tools for cancer in primary care. Br J Cancer 2015 11312. 2015;113(12):1645-1650. doi:10.1038/bjc.2015.409
68. Vermunt L, Muniz-Terrera G, Ter Meulen L, et al. Prescreening for European Prevention of Alzheimer Dementia (EPAD) trial-ready cohort: impact of AD risk factors and recruitment settings. Alzheimers Res Ther. 2020;12(1). doi:10.1186/S13195-019-0576-Y
69. Ball H, McWhirter L, Ballard C, et al. Functional cognitive disorder: dementia’s blind spot. Brain. 2020;143(10):2895-2903. doi:10.1093/BRAIN/AWAA224
70. McWhirter L, Ritchie C, Stone J, Carson A. Functional cognitive disorders: a systematic review. The lancet Psychiatry. 2020;7(2):191-207. doi:10.1016/S2215-0366(19)30405-5
71. Alexander M, Blackburn D, Reuber M. Patients’ accounts of memory lapses in interactions between neurologists and patients with functional memory disorders. Sociol Health Illn. 2019;41(2):249-265. doi:10.1111/1467-9566.12819
72. Bemelmans SASA, Tromp K, Bunnik EM, et al. Psychological, behavioral and social effects of disclosing Alzheimer’s disease biomarkers to research participants: a systematic review. Alzheimer’s Res Ther. 2016;8(1):1-17. doi:10.1186/s13195-016-0212-z
73. Saunders S, Sheehan S, Evans A, Luz S, Ritchie CW, Terrera GM. The electronic person specific outcome measure (ePSOM) programme: Overview and survey results. Alzheimer’s Dement. 2020;16(S6):e040097. doi:10.1002/ALZ.040097
74. Wimo A, Guerchet M, Ali G-C, et al. The worldwide costs of dementia 2015 and comparisons with 2010. Alzheimer’s Dement. 2017;13(1):1-7. doi:10.1016/j.jalz.2016.07.150
75. Breeze P, Thomas C, Thokala P, Lafortune L, Brayne C, Brennan A. The Impact of Including Costs and Outcomes of Dementia in a Health Economic Model to Evaluate Lifestyle Interventions to Prevent Diabetes and Cardiovascular Disease. Med Decis Mak. 2020;40(7):912-923. doi:10.1177/0272989X20946758
76. Mukadam N, Anderson R, Knapp M, et al. Effective interventions for potentially modifiable risk factors for late-onset dementia: a costs and cost-effectiveness modelling study. Lancet Heal Longev. 2020;1(1):e13-e20. doi:10.1016/s2666-7568(20)30004-0