M. Milà-Alomà1,2,3,4, G.Salvadó1,2, M. Shekari1,2,3, O. Grau-Rivera1,2,4,5, A. Sala-Vila1,2, G. Sánchez-Benavides1,2,4, E.M. Arenaza-Urquijo1,2,4, J.M. González-de-Echávarri1,2, M. Simon6, G. Kollmorgen7, H. Zetterberg8,9,10,11, K. Blennow8,9, J.D. Gispert1,2,3,12, M. Suárez-Calvet1,2,4,5,
J.L. Molinuevo1,2,3,4 for the ALFA study†
1. Barcelonaβeta Brain Research Center (BBRC), Pasqual Maragall Foundation. Barcelona, Spain; 2. IMIM (Hospital del Mar Medical Research Institute), Barcelona, Spain; 3. Universitat Pompeu Fabra, Barcelona, Spain; 4. Centro de Investigación Biomédica en Red de Fragilidad y Envejecimiento Saludable (CIBERFES), Madrid, Spain; 5. Servei de Neurologia, Hospital del Mar, Barcelona, Spain; 6. Roche Diagnostics International Ltd, Rotkreuz, Switzerland; 7. Roche Diagnostics GmbH, Penzberg, Germany; 8. Department of Psychiatry and Neurochemistry, Institute of Neuroscience and Physiology, Sahlgrenska Academy, University of Gothenburg, Sweden;
9. Clinical Neurochemistry Laboratory, Sahlgrenska University Hospital, Mölndal, Sweden; 10. Department of Neurodegenerative Disease, UCL Institute of Neurology, Queen Square, London, United Kingdom; 11. UK Dementia Research Institute at UCL, London, United Kingdom; 12. Centro de Investigación Biomédica en Red Bioingeniería, Biomateriales y Nanomedicina, Madrid, Spain; †The complete list of collaborators of the ALFA Study can be found in the acknowledgements section.
Corresponding Authors: José Luis Molinuevo, Alzheimer Prevention Program – Barcelonaβeta Brain Research Center, Wellington 30, 08005, Barcelona, Spain, +34933160990, E-mail: jlmolinuevo@barcelonabeta.org; Marc Suárez-Calvet, Alzheimer Prevention Program – Barcelonaβeta Brain Research Center, Wellington 30, 08005, Barcelona, Spain
+34933160990, E-mail: msuarez@barcelonabeta.org
J Prev Alz Dis 2021;1(8):68-77
Published online September 29, 2020, http://dx.doi.org/10.14283/jpad.2020.51
Abstract
Amyloid-β (Aβ) positivity is defined using different biomarkers and different criteria. Criteria used in symptomatic patients may conceal meaningful early Aβ pathology in preclinical Alzheimer. Therefore, the description of sensitive cutoffs to study the pathophysiological changes in early stages of the Alzheimer’s continuum is critical. Here, we compare different Aβ classification approaches and we show their performance in detecting pathophysiological changes downstream Aβ pathology.
We studied 368 cognitively unimpaired individuals of the ALFA+ study, many of whom in the preclinical stage of the Alzheimer’s continuum. Participants underwent Aβ PET and CSF biomarkers assessment. We classified participants as Aβ -positive using five approaches: (1) CSF Aβ42 < 1098 pg/ml; (2) CSF Aβ42/40 < 0.071; (3) Aβ PET Centiloid > 12; (4) Aβ PET Centiloid > 30 or (5) Aβ PET Positive visual read. We assessed the correlations between Aβ biomarkers and compared the prevalence of Aβ positivity. We determined which approach significantly detected associations between Aβ pathology and tau/neurodegeneration CSF biomarkers.
We found that CSF-based approaches result in a higher Aβ-positive prevalence than PET-based ones. There was a higher number of discordant participants classified as CSF
Aβ-positive but PET Aβ-negative than CSF Aβ-negative but PET Aβ-positive. The CSF Aβ 42/40 approach allowed optimal detection of significant associations with CSF p-tau and t-tau in the Aβ-positive group.
Altogether, we highlight the need for sensitive Aβ -classifications to study the preclinical Alzheimer’s continuum. Approaches that define Aβ positivity based on optimal discrimination of symptomatic Alzheimer’s disease patients may be suboptimal for the detection of early pathophysiological alterations in preclinical Alzheimer.
Key words: Alzheimer’s disease, biomarkers, cerebrospinal fluid, positron emission tomography, preclinical Alzheimer.
Introduction
Alzheimer’s disease (AD) is preceded by a long preclinical phase, which is characterized by the emergence of pathological brain changes in the absence of evident clinical symptoms. Cognitive symptoms may arise decades after the first pathological brain changes and can eventually progress to dementia. AD is therefore understood as a continuum (the Alzheimer’s continuum) entailing a preclinical (i.e., asymptomatic) and a clinical (i.e., symptomatic) phase (1, 2). Following the latest NIA-AA guidelines, the term ‘Alzheimer’s disease’ should be applied when there is biomarker evidence of both amyloid-β (Aβ) and tau pathologies (2, 3).
A key aspect in preclinical Alzheimer’s studies is the biomarker-based definition of Aβ pathology. Currently established Aβ pathology biomarkers can be divided in two main categories: fluid [mainly cerebrospinal fluid (CSF) and, more recently, also blood] and neuroimaging (Aβ PET) biomarkers (4, 5). Although all these biomarkers may be used on a continuous scale, cutoffs are needed to dichotomize biomarker values for diagnosis or research individual categorization purposes. Different approaches may be used to establish biomarker cutoffs. These mainly include: (1) comparison against a gold standard (neuropathology, other validated biomarkers reflecting pathology), (2) optimal discrimination between a control and a pathological group (case-control studies), (3) prediction of disease progression, or (4) description of abnormality based on population percentiles in a ‘normal’ or reference population (e.g., 95% of the unaffected population have lower/higher values) (2, 6, 7).
Regarding CSF Aβ biomarkers, a decrease in CSF Aβ42 is the widely known pattern in AD, which also correlates with increased Aβ PET uptake (8, 9). In addition, CSF Aβ42 cutoffs have been validated against Aβ PET as gold standard (10, 11). Nevertheless, the use of CSF Aβ42 may be limited by interindividual differences in Aβ42 production, and by the impact of pre-analytical and assay-related factors. To overcome some of these limitations, normalization of Aβ42 with Aβ40, by using the Aβ42/40 ratio, was proposed (12). Studies have shown that the CSF Aβ42/40 ratio is a better predictor of abnormal Aβ PET and that the ratio allows a more accurate identification of AD patients than CSF Aβ42 (13, 14).
In the clinical setting, Aβ PET imaging is used to detect Aβ pathology in patients with cognitive impairment suspected to be AD-related. The definition of a positive or negative scan is done through visual read (VR) by trained specialists, who categorize images according to well-established criteria. The VR method has been validated against neuropathology and has shown high concordance with quantitative methods (15–17). Aβ PET images can be quantified using standardized uptake value ratios (SUVR), as well as with the “Centiloid” (CL) scale. The CL scale is a method for standardizing measurements to a uniform scale, thus facilitating comparisons across radiotracers and also across studies (18), and has a robust association with pathology (19,20).
It is important to note that, although binary cutoffs allow us to stratify patients or research participants according to their biomarker profile, there is a continuum of Aβ pathology changes, that is a dynamic transition from Aβ-negative to Aβ-positive states. Therefore, binarization may leave emerging Aβ pathology undetected. This is especially important in the study of preclinical Alzheimer, in which the use of cutoffs that have been defined for a clinical use possibly conceals meaningful amounts of early Aβ pathology. Thus, the description of cutoffs that are sensitive enough to detect subtle pathological changes in the very early stage of the Alzheimer’s continuum is of great importance (21, 22).
The aim of this study is to describe and compare different Aβ classification approaches, derived through both CSF and PET measures, and to show their performance in the detection of other early pathophysiological changes in the Alzheimer’s continuum. We hypothesize that an optimal Aβ classification approach at this early stage would set the point where Aβ-downstream pathophysiological changes start.
Methods
Participants
The ALFA+ cohort is a nested longitudinal study of the ALFA (for ALzheimer and FAmilies) study, which aims at characterizing the preclinical stage of the Alzheimer’s continuum (23). The ALFA study includes 2,743 cognitively unimpaired individuals, aged between 45 and 75 years old, which are enriched for family history of AD and APOE-ε4 carriership. In the ALFA+ cohort, participants undergo a more detailed characterization, which entails acquisition of CSF samples for biomarker levels determination, neuroimaging biomarkers (MRI and FDG and Aβ PET), APOE genotyping and cognitive assessments. Among the ALFA+ participants, 381 had available CSF biomarker measurements. Since we aimed at studying participants in the Alzheimer’s continuum, we excluded 13 participants that had a CSF biomarker of suspected non-Alzheimer’s pathology (2), as defined by CSF Aβ42/40 ≥ 0.071 and CSF p-tau >24 pg/ml. None of them was Aβ-positive using Aβ PET in any of the approaches defined below. Thus, 368 participants were finally included in the present study. Of those, a subset of 303 and 301 participants also had CL quantification or VR assessment of Aβ PET imaging, respectively. For all participants, the time difference between CSF collection and Aβ PET assessments was less than one year.
CSF biomarkers assessment
CSF samples were obtained by lumbar puncture and collected, processed and stored following standard procedures (24). Total tau (t-tau) and phosphorylated tau (p-tau) measurements were performed using the electrochemiluminiscence Elecsys® Total-Tau CSF and Phospho-Tau(181P) CSF immunoassays, respectively. CSF Aβ42 and Aβ40 were measured with the prototype NeuroToolKit (Roche Diagnostics International Ltd.). All assays were performed on a fully automated cobas e 601 or e 401 instrument (Roche Diagnostics International Ltd.) at the Clinical Neurochemistry Laboratory, Sahlgrenska University Hospital, Mölndal, Sweden.
PET imaging acquisition and processing
Imaging acquisition and preprocessing protocols have been described previously (20). In brief, a T1-weighted MRI and an [18F] Flutemetamol PET scan was acquired in all participants. The T1-weighted 3D-TFE sequence was acquired in a Philips 3 T Ingenia CX scanner. PET imaging was conducted in a Siemens Biograph mCT, following a cranial CT scan for attenuation correction. Participants were injected with 185 MBq (range 166.5–203.5 Mbq) of [18F] Flutemetamol, and 4 frames of 5 min each were acquired 90 min post-injection.
PET processing was performed following the standard CL pipeline (18) using SPM12. In brief, PET images are first coregistered to their respective T1-weighted images and, afterwards, moved to MNI space using the normalization transformation derived from the segmentation of the T1-weighted image. PET images were intensity normalized using whole cerebellum as reference region. CL values were calculated from the mean values of the standard CL target region (http://www.gaain.org/centiloid-project) using the transformation previously calibrated (20).
A nuclear medicine physician visually rated the scans as Aβ-positive or Aβ-negative using standard clinical criteria as specified in the Summary of Product Characteristics of the tracer (https://www.ema.europa.eu/en/documents/product-information/vizamyl-epar-product-information_en.pdf).
Aβ pathology biomarkers and cutoffs
We classified the participants as Aβ-positive or Aβ-negative using five different Aβ biomarker/cutoff combinations (Aβ classification approaches; Table 1). Each of the approaches were determined as follows. In approach 1, participants with a CSF Aβ42 < 1098 pg/ml were considered as Aβ-positive. This cutoff had been previously established in concordance with Aβ PET in a similar cohort and had area under the ROC curve (AUC) of 0.85 to discriminate between Aβ PET-positive or negative (10). In approach 2, we used CSF Aβ42/40 as a biomarker of Aβ pathology and we determined a cutoff using a Gaussian mixture model (GMM). The cutoff was defined as the mean minus 2 standard deviations (SD) of the non-pathologic Gaussian distribution. As the fitting of the GMM can vary depending on initial conditions, the process was repeated 1000 times with random initial conditions. The final cutoff for CSF Aβ42/40, derived as the median value of the 1000 repetitions, was 0.071. In both approaches 3 and 4, we used the Aβ PET CL scale as biomarker of Aβ pathology, but with different cutoffs. In approach 3, we defined Aβ positivity when CL > 12; this cutoff has been previously proposed to detect subtle Aβ pathology in a comparison against CSF Aβ42 (20). In approach 4, we defined Aβ positivity when CL > 30, a cutoff that falls within the optimal range of agreement with VR method in clinical populations (24 – 35 CL), and has been proposed to detect established Aβ pathology (19,20,25–27). Finally, in approach 5, we categorized participants as Aβ-positive or Aβ-negative using the Aβ PET VR, the approach most commonly used in the clinical setting.
Abbreviations: Aβ, amyloid-β; CSF, cerebrospinal fluid; CL: Centiloids.
Statistical analysis
CSF biomarker distributions were tested for normality with the Kolmogorov-Smirnov test and histograms visual inspection. CSF Aβ42, p-tau and t-tau did not follow a normal distribution and were thus log10-transformed. In contrast, CSF Aβ42/40 ratio followed a normal distribution and was not transformed. CSF biomarker extreme values were identified following Tukey criterion (i.e., values falling at over three times the interquartile range below the first quartile or above the third quartile) and excluded from the analyses.
Differences in demographic characteristics and CSF biomarker values as a function of Aβ classification schemes were assessed by means of chi-squared or one-way analysis of variance (ANOVA) tests, as appropriate. Correlations between Aβ biomarkers were tested using partial Spearman rank correlation, corrected by age. We used a chi-squared analysis in order to test significant differences in Aβ-positive prevalence using different Aβ classification approaches.
We tested the association between tau and neurodegeneration CSF biomarkers (i.e., CSF p-tau, t-tau) and the Aβ biomarker (i.e., CSF Aβ42, Aβ42/40 or CL scale) in a linear model, adjusting by the effect of age and sex. These analyses were performed separately in Aβ-positive or Aβ-negative groups as defined by each approach. Moreover, in order to determine which of the approaches was more sensitive to detect early pathophysiological changes, we conducted the same analyses in the whole cohort but additionally introducing in the model the interaction term ‘Aβ biomarker x Aβ group’. A significant interaction term denotes that the association of the Aβ biomarker with CSF p-tau and t-tau differs between the Aβ-negative and Aβ-positive groups, as defined by that approach. These analyses were performed in each of the five Aβ classification approaches.
All statistical models were adjusted for the effects of age and sex. For all analysis, we applied a false discovery rate (FDR) multiple comparison correction following the Benjamini-Hochberg procedure (28). All tests were 2-tailed, with a significance level of α = 0.05.
Results
Participants’ demographics
Participants’ characteristics are depicted in Table 2. We compared the demographical characteristics of the Aβ-positive and Aβ-negative groups defined by the different Aβ classification approaches. We observed that Aβ-positive participants were significantly older using PET-based versus CSF-based approaches. There were not significant differences in years of education, frequency of the APOE-ε4 allele or sex between the different Aβ-classification approaches.
Most of the AD biomarkers significantly differed between the five Aβ classification approaches within the Aβ positive or Aβ-negative group (Table 2). PET-based Aβ classification approaches (namely, approach 3, 4 and 5) had a more pathologic biomarker profile (that is, lower CSF Aβ42 and CSF Aβ42/40 ratio, but higher CL scale and CSF p-tau and t-tau), than the CSF-based Aβ classification approaches (namely, approach 1 and 2). These results suggest that CSF-based Aβ biomarkers are capturing Aβ accumulation at an earlier stage of the Alzheimer’s continuum. This idea is supported by the fact that Aβ-negative groups defined by PET-based approaches had higher levels of CSF p-tau and t-tau (Table 2).
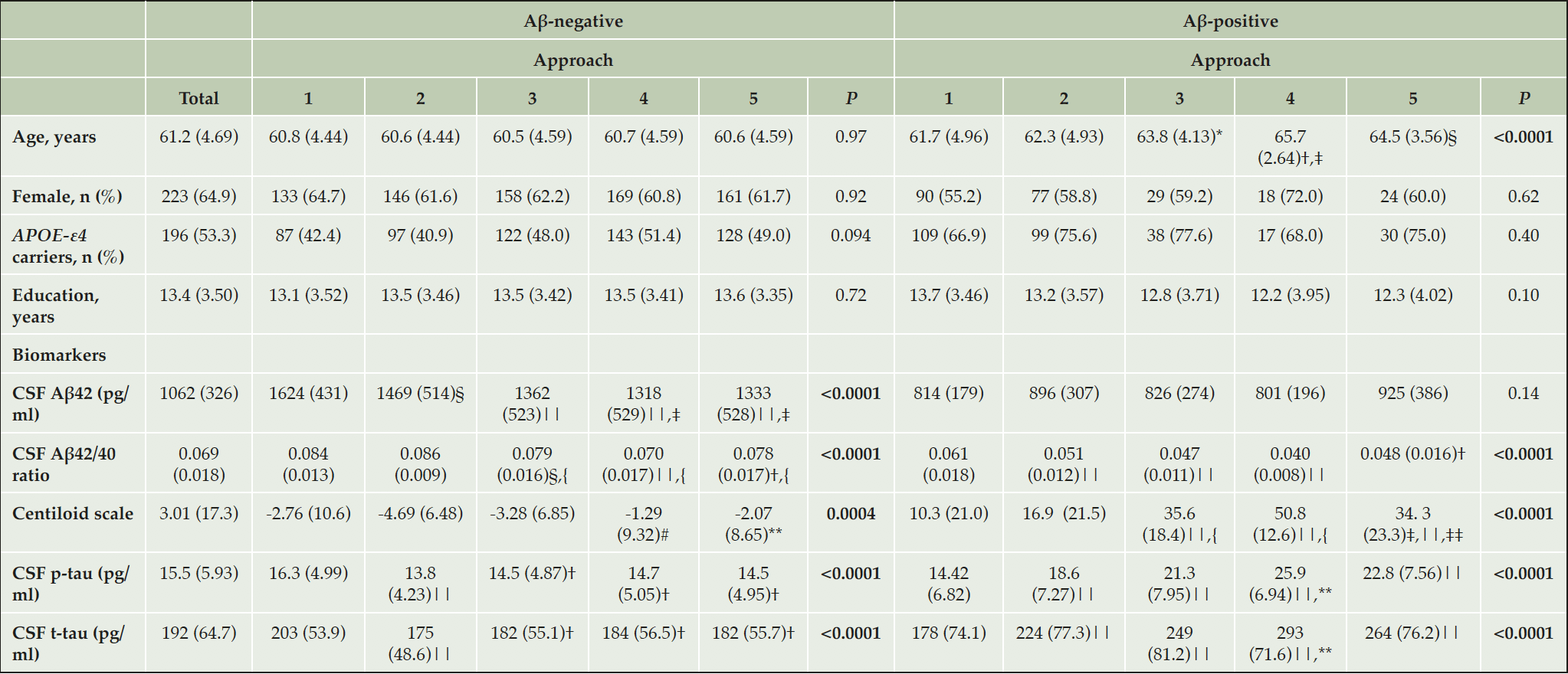
Table 2. Participants’ demographic characteristics and biomarker levels by five Aβ classification approaches
Data are expressed as mean (M) and standard deviation (SD) or number of participants (n) and percentage (%), as appropriate. One-way ANOVA followed by Tukey corrected post hoc comparisons was used to compared age and education and Pearson’s Chi-square test to compare sex and APOE-ε4 status between approaches within each Aβ-group. CSF biomarkers and centiloid scale were compared with an ANCOVA adjusted by age and sex followed by Tukey corrected post hoc comparison. The P-values indicated in the last column refer to the approach main effect. All P-values are corrected for multiple comparisons using the FDR method; Abbreviations: Aβ42, amyloid-β 42; p-tau, phosphorylated tau; t-tau, total tau; * P<0.05 vs approach 1; † P<0.001 vs approach 1; ‡ P<0.01 vs approach 2; § P<0.01 vs approach 1; ||P<0.0001 vs approach 1; { P<0.0001 vs approach 2; # P<0.001 vs approach 2; ** P<0.05 vs approach 2; ‡‡ P< 0.05 vs approach 4
Correlations between Aβ biomarkers
We tested the correlations between CSF and PET Aβ biomarkers, corrected by age. There was a moderate correlation between CSF Aβ42 and CL values (Spearman’s rho = -0.41; P<0.0001; Figure 1A and B) and CSF Aβ42/40 and CL values (Spearman’s rho = -0.54; P<0.0001; Figure 1C and D). There was also a high correlation between CSF Aβ42 and CSF Aβ42/40 ratio (Spearman’s rho = -0.75; P<0.0001; Figure 1E). Prevalence of Aβ positivity using different Aβ classification approaches We next determined the prevalence of Aβ-positive and Aβ-negative participants according to each Aβ classification approach and, remarkably, we found a wide range of Aβ-positive prevalences ranging from 8.25 to 44.3% (Figure 2; Table 3). Approach 1 (i.e., CSF Aβ42) resulted in the highest prevalence of Aβ-positive participants (N = 163, 44.3%), followed by approach 2 (CSF Aβ42/40 ratio; Aβ-positive: N = 131, 35.6%), approach 3 (CL > 12; Aβ-positive: N = 49, 16.2%), approach 5 (VR; Aβ-positive: N = 40, 13.3%) and, finally, approach 4 (CL > 30; Aβ-positive: N = 25, 8.25%) (Figure 2). The prevalence of Aβ-positive participants in CSF-based classification approaches (approach 1 and 2) was significantly higher than that in PET-based classification approaches (approach 3, 4 and 5, P < 0.0001; Figure 2). Notably, approach 3 (CL > 12) showed a statistically significant higher prevalence of Aβ-positive participants compared to approach 4 (CL > 30, P = 0.043, Figure 2).
Scatter plots representing the associations between each Aβ biomarker. Each point depicts the value of the Aβ biomarker of an individual. Green areas depict discordant subjects and percentage of them is shown in red. The red dashed lines indicate the Aβ biomarker cutoffs used in each Aβ classification approach: CSF Aβ42 = 1098 pg/ml, CSF Aβ42/40 = 0.071, CL = 12 and CL = 30. A, B. Correlation and comparison between CSF Aβ42 and CL; cutoff CL = 12 (A) and CL = 30 (B). C, D. Correlation and comparison between CSF Aβ42/40 and CL; cutoff CL = 12 (C) and CL = 30 (D). E. Correlation and comparison between CSF Aβ42 and CSF Aβ42/40. F. Comparison between CSF Aβ42 and VR. G. Comparison between CSF Aβ42/40 and VR. H, I. Comparison between VR and CL; cutoff CL = 12 (H) and CL = 30 (I). Abbreviations: Aβ, amyloid β; CL, Centiloids; CSF, Cerebrospinal fluid; PET, Positron Emission Tomography
The graph depicts the prevalence of the Aβ-positive (Aβ+; color) and Aβ-negative (Aβ-; grey) individuals in each Aβ classification approach. We conducted a pair wise chi squared comparison between all the approaches and we found significant statistical differences between them (P < 0.0001). Bonferroni-corrected pair-wise post hoc comparisons: * P<0.0001 vs approach 1 and approach 2¸† P<0.05 vs approach 3. Abbreviations: Aβ, amyloid-β; CL, Centiloids; CSF, Cerebrospinal fluid; PET, Positron Emission Tomography; VR, Visual Reads.
We assessed the differences between the CSF- and PET-defined Aβ-positive participants (i.e., CSF/PET-discordant participants) within each Aβ classification approach (shown in Table 3 and Figure 1). The participants that were classified as CSF Aβ-positive but PET Aβ-negative range from 19.8 to 37.0% (Table 3; green areas in Figure 1). The highest discordance occurred between Aβ classification approach 4 (CL < 30) and approach 1 (CSF Aβ42; 37.0% discordance; Figure 1B), and the lowest occurred between approach 3 (CL < 12) and approach 2 (CSF Aβ42/40; 19.8% discordance, Figure 1C). By contrast, fewer participants showed discordance in the other direction, i.e., a CSF Aβ-negative but a PET Aβ-positive biomarker (range: 0.33% to 2.99%; Figure 1; Table 3). Of note, the proportion of discordant participants was higher for CSF Aβ42 (approach 1) than for CSF Aβ42/40 ratio (approach 2) in all comparisons. Association of Aβ biomarkers with tau pathology and neurodegeneration CSF biomarkers We next assessed whether changes in tau pathology and neurodegeneration CSF biomarkers as a function of Aβ pathology differed before and after the computed Aβ cutoffs. We hypothesized that an optimal Aβ biomarker cutoff would discriminate between an Aβ-negative state (where Aβ pathology is not associated with downstream tau pathology and neurodegeneration changes) and an Aβ-positive state (where those downstream changes arise). For these purposes, we computed the association between each Aβ biomarker and CSF p-tau and t-tau in Aβ-negative and Aβ-positive groups defined by the different Aβ classification approaches. We also computed the interaction term ‘Aβ biomarker x Aβ group’. The results of the analyses are summarised in Table 4 and Figure 3. Remarkably, robust statistically significant interactions occurred using approach 2. In other words, the cutoff derived from the CSF Aβ42/40 ratio significantly discriminated between two states: (1) an Aβ-negative state where tau and neurodegeneration CSF biomarkers were not associated with CSF Aβ42/40 levels and (2) an Aβ-positive state where CSF p-tau and t-tau significantly increase as a function of higher Aβ pathology (i.e., decreased CSF Aβ42/40 ratio). In approach 1 (i.e., CSF Aβ42), we also observed statistical significance in the interaction term ‘Aβ biomarker x Aβ group’ for CSF p-tau and t-tau. Nevertheless, both CSF p-tau and t-tau were positively associated with CSF Aβ42 in the Aβ-negative group. None of the Aβ-positive and Aβ-negative groups defined with Aβ PET-based approaches differed in their association between CL scale and CSF p-tau and t-tau. However, there was a tendency to statistical significance in the interaction term ‘Aβ biomarker x Aβ group’ for CSF p-tau in approach 3 (i.e., CL > 12). Moreover, there was a significant association between CSF p-tau and t-tau with CL scale in the Aβ-positive group using approach 3 and approach 5 (i.e., VR).
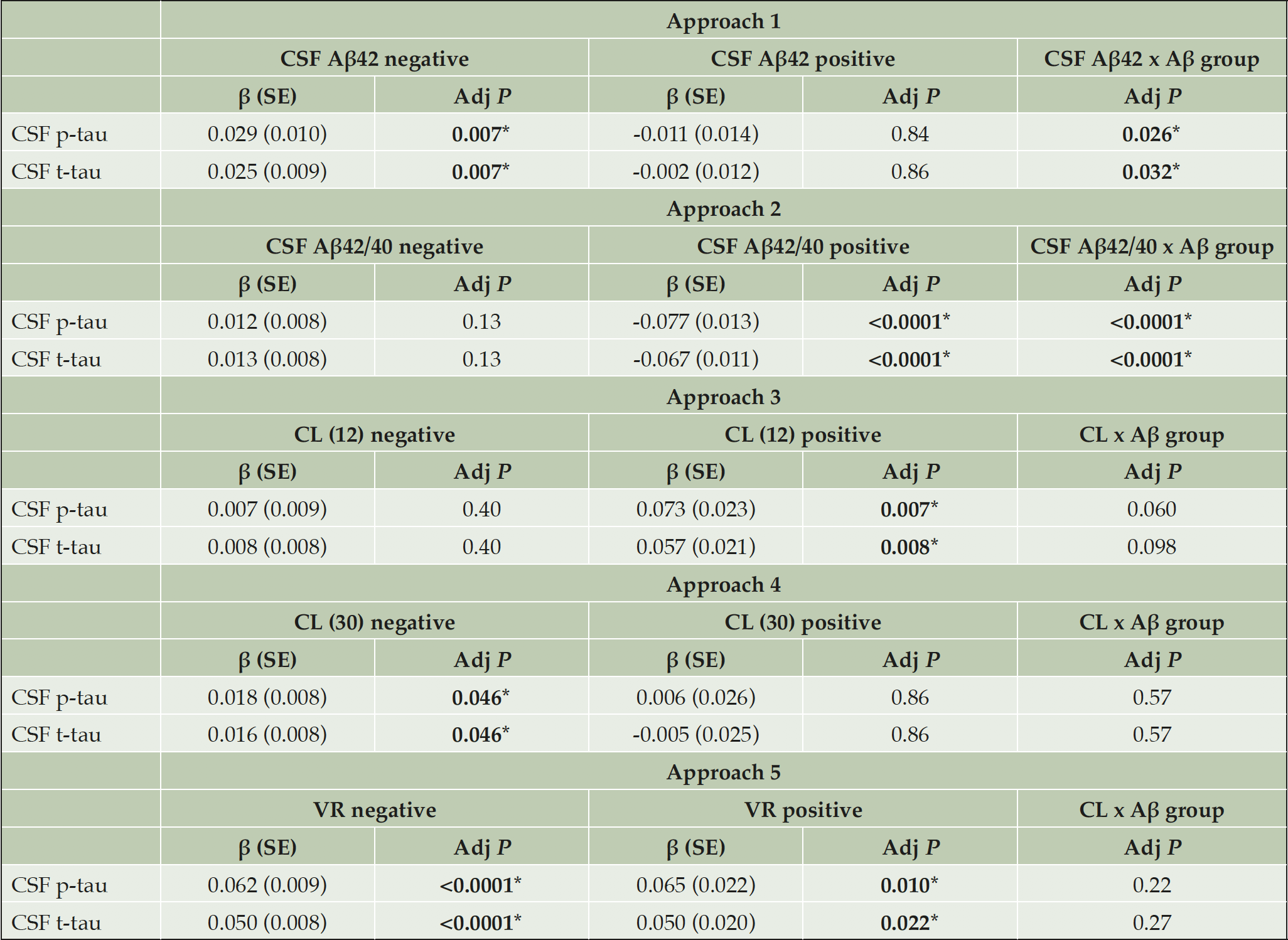
Table 3. Contingency table with the percentage of agreement and discordance between the different Aβ classification approaches
Data are expressed as percentage (%) of participants in each category. Bold letters indicate discordance. We performed a chi-square test of independence to examine the relation between Aβ positivity and the Aβ classification approach used and we observed that the percentage of Aβ positivity was not equally distributed between Aβ-classification approaches X2 (4, N = 301) = 176, P < 0.0001. A Bonferroni corrected pair-wise post hoc comparisons showed significant differences between the approach 1 and approach 3, 4 and 5 (P < 0.0001)¸ between the approach 2 and approach 3, 4 and 5 (P < 0.0001), and between approach 3 and 4 (P < 0.05). Abbreviations: Aβ, amyloid-β; Aβ+, amyloid-β positive; Aβ-, amyloid-β negative; CL, Centiloids; VR, Visual Read.
Scatter plots representing the associations of CSF p-tau and t-tau with each Aβ biomarker in Aβ-positive and Aβ-negative groups. Each point depicts the value of the CSF biomarker of an individual and the solid lines indicate the regression line for each group. The P-values shown for the ‘Aβ biomarker x Aβ group’ interaction were computed using a linear model adjusting for age and sex. All P-values are corrected for multiple comparisons using the FDR method. The dashed lines indicate the Aβ biomarker cutoffs used in each Aβ classification approach: CSF Aβ42 = 1098 pg/ml, CSF Aβ42/40 = 0.071, CL = 12 and CL = 30; Abbreviations: Aβ, amyloid β; CL, Centiloids; CSF, Cerebrospinal fluid; PET, Positron Emission Tomography; p-tau, phosphorylated tau; t-tau, total tau; VR, Visual Reads.
CSF p-tau or t-tau were assessed by a linear model with the Aβ biomarker (CSF Aβ42, CSF Aβ42/40 or CL) as main effect and age and sex as covariates. This analysis was performed separately in Aβ-negative or Aβ-positive groups as defined by each approach. Moreover, we added the interaction term ‘Aβ biomarker x Aβ group’ in order to test statistical differences in the regression slopes between Aβ-negative and –positive groups. The standardized regression coefficients (β) and standard errors (SE) are depicted. P-values are corrected for multiple comparisons using FDR method. *Significant values; Abbreviations: Aβ42, amyloid-β 42; CL, Centiloids; p-tau, phosphorylated tau; t-tau, total tau; VR, Visual Read.
Discussion
In this study, we compared five different approaches to classify cognitively unimpaired individuals as Aβ-positive or Aβ-negative, and we found considerable differences between them. Specifically, our results show that: (1) Aβ-positive prevalence vary from 8 to 44% depending on the approach used; (2) Aβ CSF-based approaches result in the highest prevalence of Aβ-positive subjects compared with PET-based approaches; (3) Discordant classification appears more frequently as a CSF Aβ-positive but PET Aβ-negative (19.8 – 37.0%), while only a few individuals were CSF Aβ-negative but PET Aβ-positive (0.33 – 2.99%); (4) In Aβ PET, the use of a lower CL cutoff (12 CL instead of 30 CL) increases the detection of Aβ-positive individuals based on CSF levels; (5) among all Aβ classification approaches assessed, the CSF Aβ42/40 ratio cutoff shows the greater concordance with Aβ PET imaging (using the 12 CL cutoff), and the most significant difference of regression slopes between the Aβ-positive and Aβ-negative groups in the association with Aβ downstream pathology (e.g., tau pathology and neurodegeneration).
The ATN classification is widely used in AD research to classify patients or study participants based on biomarker evidence of Aβ pathology (A), tau pathology (T) or neurodegeneration/neuronal injury (N) (7). Each biomarker group can be defined with different biomarkers. For Aβ pathology (A), the most accepted biomarkers are CSF Aβ42, the CSF Aβ42/40 ratio and Aβ PET.
In regard to CSF Aβ biomarkers, CSF Aβ42 has been the most widely used, and cutoffs have been established against Aβ PET (10,11). However, increasing evidence favors the use of CSF Aβ42/40 ratio to normalize both preanalytical and interindividual differences, which provides a higher reproducibility and specificity for AD pathology (13,14). We show that the CSF Aβ42 approach results in a higher number of Aβ-positive participants than any other approach. However, CSF Aβ42 approach also results in a higher frequency of discrepancies with the PET-based approaches compared to the CSF Aβ42/40 ratio approach. This observation is in line with the idea that the CSF Aβ42/40 ratio predicts more accurately Aβ PET than CSF Aβ42. Furthermore, a high concordance between Aβ PET VR and ratios of CSF biomarkers have been shown to best distinguish Aβ-positive from negative individuals in both cognitively unimpaired and impaired populations. The CSF p-tau/Aβ42 ratio has a high diagnostic clinical utility as its cutoff has been derived using Aβ PET VR concordance (10). However, we have not used this ratio in this setting because it represents a mixed pathology of Aβ and tau, and tau biomarkers become abnormal later in the disease stage. For CSF Aβ42, we used the cutoff of 1098 pg/ml, that was previously defined in a study where CSF Aβ42 was measured by a Roche Elecsys assay and a ROC curve was built in comparison against Aβ PET. This cutoff is also very similar to that obtained in the BioFINDER study (1100 pg/ml) which is anchored to a positive Aβ PET VR (11).
For CSF Aβ42/40, we rendered a cutoff with a different approach. Instead of comparing the CSF values with Aβ PET as the gold standard, we applied a data-driven approach, namely a Gaussian Mixture Modelling (GMM). This method allows to describe a normal range of CSF Aβ42/40 ratios in the non-pathological group and derive a cutoff defined as the mean minus 2 standard deviations of this Gaussian distribution. By definition, under this criterion, 97.7% of Aβ-negative subjects are expected to display high CSF Aβ42/40 values. In other words, this criterion is highly specific by design. We hypothesised that this method would be more sensitive to detect incipient Aβ pathology in cognitively unimpaired individuals than criteria based on discriminating asymptomatic from symptomatic AD populations. In fact, GMM has already been used to derive cohort-specific CSF Aβ cutoffs, showing a robust ability to discriminate normal and pathologic distributions of Aβ42 (29–31). Our results confirm the suitability of both using the CSF Aβ42/40 ratio and deriving its cutoff with a GMM method. In fact, approach 2 shows the lowest number of discordant values compared with any other approach. According with our hypothesis, we observed that this approach provides optimal discrimination between Aβ-negative participants, showing no associations with Aβ-downstream pathologies (as measured by CSF p-tau and t-tau), and Aβ-positive participants, which display significant association with them. This is shown by the fact that this approach results in a significant difference of regression slopes between the Aβ-positive and Aβ-negative groups in the association between CSF p-tau or t-tau as a function of Aβ pathology. In fact, visual inspection of Figure 4 shows that the CSF Aβ42/40 cutoff we have computed by GMM falls close to the intersection of the regression lines in the Aβ-positive and Aβ-negative groups. Remarkably, this result is not seen when using the CSF Aβ42 approach. In this approach, we found unexpected significant positive correlations between CSF Aβ42 and both CSF p-tau and t-tau in the Aβ-negative group. Without the Aβ40 normalization, CSF Aβ42 may unspecifically correlate with other proteins because of the abovementioned limitations in terms of interindividual differences (high and low Aβ producers) and preanalytical variability.
In relation to Aβ PET biomarkers, Aβ PET classification can be performed through VR, widely used in the clinical practice, or using a quantitative approach such as the CL scale. This scale is expressed as universal units and allows the comparison among different tracers and studies (18). Different cutoffs to categorize participants according to CL might be used, depending on the sample and on the study objectives. Optimal cutoffs for CL against VR typically fall within the range of 24 – 35 CL in clinical populations (25–27). However, these VR-based CL cutoffs might not be optimal to study the preclinical stage of the Alzheimer’s continuum, when the goal is to detect subtle Aβ accumulation. Therefore, here we compared three different PET-based approaches: CL > 12, which was established as a cutoff with an optimal agreement against the standard cutoff of CSF Aβ42 < 1098 pg/ml (20) and is expected to detect subtle early Aβ changes; CL > 30, a cutoff falling within the range proposed to robustly detect established Aβ pathology (19,20,25–27); and VR, expected to represent a cutoff closer to the clinical practice. As expected, a cutoff of CL > 12 showed a higher prevalence of Aβ-positive individuals than the other two PET-based approaches. The slopes of CSF p-tau or t-tau as a function of CL did not significantly differ between the Aβ-positive and Aβ-negative groups defined by the CL12 approach, although tendency to this direction is observed, especially for CSF p-tau. However, the CL12 approach allowed the detection of significant associations of CSF p-tau and t-tau in the Aβ-positive group, an association that is also observed with the VR approach, but not with the CL30 one. These results suggest that the CL12 approach may be detecting Aβ deposition that is already associated with increasing tau pathology and neurodegeneration biomarkers. On the contrary, classifying Aβ groups using CL > 30 as cutoff, resulted in the lowest prevalence of Aβ-positive individuals and did not allow the discrimination of changes in Aβ-downstream CSF biomarkers changes between the two Aβ-groups. This suggests that 30 CL is a late cutoff to apply in preclinical Alzheimer’s studies, especially in cohort such as ALFA+, comprised by individuals in the very earliest phase of the Alzheimer’s continuum. Aβ-positive subjects according to the CL30 criterion are probably in a more advanced stage of Aβ pathology. We did not find a significant association between either CSF t-tau or p-tau with CL in the Aβ-positive group defined by the CL30 approach. This might be explained by the reduced sample size in that Aβ-positive group (n = 25), but also because Aβ-pathology might start to plateau in this late stage of preclinical Alzheimer. Conversely, a positive association is observed in the Aβ-negative group, supporting the idea that CL30 is a late cutoff and that Aβ-downstream processes have arisen before this cutoff. Interestingly, the VR approach rendered an Aβ-positive prevalence closer to the CL12 approach than to the CL30 approach. Of note, the raters in our study are highly trained to detect early Aβ deposition, but may differ in other studies or in the clinical setting. Similarly to the CL30 approach, the VR approach does not discriminate between the associations of the CL scale with CSF t-tau or p-tau in the Aβ-positive and Aβ-negative groups, although in this case positive associations are found in the Aβ-positive group.
Finally, we found obvious differences between the CSF- and PET-based Aβ classification approaches. Our results show only a moderate correlation between Aβ CSF and Aβ PET, which is not surprising as they may reflect different aspects of Aβ pathology, i.e., soluble Aβ and fibrillar Aβ aggregates, respectively (1). Noteworthy, the proportion of discordant values that were CSF Aβ-positive but PET Aβ-negative was considerably higher than those that were CSF Aβ-negative but PET Aβ-positive. This is consistent with the idea that Aβ CSF biomarkers detect earlier stages of Aβ pathology than Aβ PET, that is Aβ dysregulation that precedes Aβ plaques formation (32). Furthermore, this earlier dysregulation seems to be already associated to changes in Aβ-downstream events (i.e., tau pathology and neurodegeneration), thus proving the importance of studying this very early stage.
Our study has some limitations: (1) it is a cross sectional study and we could not track the progression of Aβ biomarkers, which would be particularly relevant in the Aβ-discordant biomarkers individuals; (2) it is a comparative analysis between different approaches, lacking a gold standard, so conclusions on sensitivity and specificity for each approach cannot be drawn. However, since comparisons among Aβ positivity criteria are made in the same dataset, conclusions on the relative sensitivity to detect associations with downstream pathophysiological events are valid; (3) results might not be generalizable to other cohorts because ALFA+ is a very specific cohort aimed at studying the preclinical stage of the Alzheimer’s continuum.
Overall, in this study we show that, in the preclinical stage of the Alzheimer’s continuum, there are important differences in the prevalence and the characteristics of Aβ-positive and Aβ-negative study participants depending on the Aβ classification approach used for the dichotomization. This emphasizes the importance of considering the sample and the study aims when deriving cutoffs for participant dichotomization. This is particularly important when studying the preclinical Alzheimer’s ccontinuum stage, where the approaches usually used in symptomatic AD patients may be not suitable to detect subtle changes in Aβ pathology.
Acknowledgements: This publication is part of the ALFA study (ALzheimer and FAmilies). The authors would like to express their most sincere gratitude to the ALFA project participants and relatives without whom this research would have not been possible.
Collaborators of the ALFA study are: Annabella Beteta, Raffaele Cacciaglia, Alba Cañas, Carme Deulofeu, Irene Cumplido, Natalia Vilor Tejedor, Ruth Dominguez, Maria Emilio, Carles Falcon, Karine Fauria, Sherezade Fuentes, Laura Hernandez, Gema Huesa, Jordi Huguet, Paula Marne, Tania Menchón, Carolina Minguillon, Grégory Operto, Albina Polo, Sandra Pradas, Anna Soteras, and Marc Vilanova. The authors thank Roche Diagnostics International Ltd. for providing the kits to measure CSF biomarkers and GE Healthcare for kindly providing 18F-flutemetamol doses of ALFA+ study participants. ELECSYS, COBAS, and COBAS E are registered trademarks of Roche. The Elecsys β-Amyloid (1-42) CSF immunoassay in use is not a commercially available IVD assay. It is an assay that is currently under development and for investigational use only. The measuring range of the assay is 200 (lower technical limit) – 1700 pg/mL (upper technical limit). The performance of the assay beyond the upper technical limit has not been formally established. Therefore, use of values above the upper technical limit, which are provided based on an extrapolation of the calibration curve, is restricted to exploratory research purposes and is excluded for clinical decision making or for the derivation of medical decision points.
Funding: The project leading to these results has received funding from “la Caixa” Foundation (ID 100010434), under agreement LCF/PR/GN17/50300004 and the Alzheimer’s Association and an international anonymous charity foundation through the TriBEKa Imaging Platform project (TriBEKa-17-519007). Additional support has been received from the Universities and Research Secretariat, Ministry of Business and Knowledge of the Catalan Government under the grant no. 2017-SGR-892. OGR is supported by the Spanish Ministry of Science, Innovation and Universities (FJCI-2017-33437). ASV is the recipient of an Instituto de Salud Carlos III Miguel Servet II fellowship (CP II 17/00029). EMAU is supported by the Spanish Ministry of Science, Innovation and Universities – Spanish State Research Agency (RYC2018-026053-I). JDG is supported by the Spanish Ministry of Science and Innovation (RYC-2013-13054). MSC received funding from the European Union’s Horizon 2020 Research and Innovation Program under the Marie Sklodowska-Curie action grant agreement No 752310, and currently receives funding from Instituto de Salud Carlos III (PI19/00155) and from the Spanish Ministry of Science, Innovation and Universities (Juan de la Cierva Incorporación grant IJC2018-037478-I). KB is supported by the Swedish Research Council (#2017-00915), the Alzheimer Drug Discovery Foundation (ADDF), USA (#RDAPB-201809-2016615), the Swedish Alzheimer Foundation (#AF-742881), Hjärnfonden, Sweden (#FO2017-0243), the Swedish state under the agreement between the Swedish government and the County Councils, the ALF-agreement (#ALFGBG-715986), and European Union Joint Program for Neurodegenerative Disorders (JPND2019-466-236). HZ is a Wallenberg Scholar supported by grants from the Swedish Research Council (#2018-02532), the European Research Council (#681712), the Swedish state under the agreement between the Swedish government and the County Councils, the ALF-agreement (#ALFGBG-720931), the Alzheimer Drug Discovery Foundation (ADDF), USA (#201809-2016862), and the UK Dementia Research Institute at UCL.
Conflicts of Interest: JLM has served/serves as a consultant or at advisory boards for the following for-profit companies, or has given lectures in symposia sponsored by the following for-profit companies: Roche Diagnostics, Genentech, Novartis, Lundbeck, Oryzon, Biogen, Lilly, Janssen, Green Valley, MSD, Eisai, Alector, BioCross, GE Healthcare, ProMIS Neurosciences. JDG has given lectures in symposia sponsored by the following for-profit companies: General Electric, Philips and Biogen. KB has served as a consultant or at advisory boards for Abcam, Axon, Biogen, Lilly, MagQu, Novartis and Roche Diagnostics, and is a co-founder of Brain Biomarker Solutions in Gothenburg AB (BBS), which is a part of the GU Ventures Incubator Program. HZ has served at scientific advisory boards for Denali, Roche Diagnostics, Wave, Samumed and CogRx, has given lectures in symposia sponsored by Fujirebio, Alzecure and Biogen, and is a co-founder of Brain Biomarker Solutions in Gothenburg AB, a GU Ventures-based platform company at the University of Gothenburg. GK is a full-time employee of Roche Diagnostics GmbH. MS is a full-time employee of Roche Diagnostics International Ltd. The remaining authors declare that they have no conflict of interest.
Ethical Standards: The ALFA+ study (ALFA-FPM-0311) was approved by the Independent Ethics Committee “Parc de Salut Mar,” Barcelona, and registered at Clinicaltrials.gov (Identifier: NCT02485730). All participants signed the study’s informed consent form that had also been approved by the Independent Ethics Committee “Parc de Salut Mar,” Barcelona.
Open Access: This article is distributed under the terms of the Creative Commons Attribution 4.0 International License (http://creativecommons.org/licenses/by/4.0/), which permits use, duplication, adaptation, distribution and reproduction in any medium or format, as long as you give appropriate credit to the original author(s) and the source, provide a link to the Creative Commons license and indicate if changes were made.
References
1. Dubois B, Hampel H, Feldman HH, Scheltens P, Aisen P, Andrieu S, et al. Preclinical Alzheimer’s disease: Definition, natural history, and diagnostic criteria. Alzheimer’s Dement. 2016 Mar;12(3):292–323.
2. Jack CR, Bennett DA, Blennow K, Carrillo MC, Dunn B, Haeberlein SB, et al. NIA-AA Research Framework: Toward a biological definition of Alzheimer’s disease. Alzheimer’s Dement. 2018;14(4):535–62.
3. Burnham SC, Coloma PM, Li Q-X, Collins S, Savage G, Laws S, et al. Application of the NIA-AA Research Framework: Towards a Biological Definition of Alzheimer’s Disease Using Cerebrospinal Fluid Biomarkers in the AIBL Study. J Prev Alzheimer’s Dis. 2019;6(4):248–55.
4. Milà-Alomà M, Suárez-Calvet M, Molinuevo JL. Latest advances in cerebrospinal fluid and blood biomarkers of Alzheimer’s disease. Ther Adv Neurol Disord. 2019 Jan 18;12:175628641988881.
5. Cavedo E, Lista S, Khachaturian Z, Aisen P, Amouyel P, Herholz K, et al. The Road Ahead to Cure Alzheimer’s Disease: Development of Biological Markers and Neuroimaging Methods for Prevention Trials Across all Stages and Target Populations. J Prev Alzheimer’s Dis. 2014 Dec;1(3):181–202.
6. Jack CR, Wiste HJ, Weigand SD, Therneau TM, Lowe VJ, Knopman DS, et al. Defining imaging biomarker cut points for brain aging and Alzheimer’s disease. Alzheimer’s Dement. 2017 Mar 1;13(3):205–16.
7. Jack CR, Bennett DA, Blennow K, Carrillo MC, Feldman HH, Frisoni GB, et al. A / T / N : An unbiased descriptive classification scheme for Alzheimer disease biomarkers. Neurology. 2016 Aug;0(5):1–10.
8. Palmqvist S, Zetterberg H, Blennow K, Vestberg S, Andreasson U, Brooks DJ, et al. Accuracy of Brain Amyloid Detection in Clinical Practice Using Cerebrospinal Fluid β-Amyloid 42. JAMA Neurol. 2014 Oct 1;71(10):1282.
9. Landau SM, Lu M, Joshi AD, Pontecorvo M, Mintun MA, Trojanowski JQ, et al. Comparing positron emission tomography imaging and cerebrospinal fluid measurements of β-amyloid. Ann Neurol. 2013 Dec;74(6):826–36.
10. Schindler SE, Gray JD, Gordon BA, Xiong C, Batrla-Utermann R, Quan M, et al. Cerebrospinal fluid biomarkers measured by Elecsys assays compared to amyloid imaging. Alzheimer’s Dement. 2018 Nov;14(11):1460–9.
11. Hansson O, Seibyl J, Stomrud E, Zetterberg H, Trojanowski JQ, Bittner T, et al. CSF biomarkers of Alzheimer’s disease concord with amyloid-β PET and predict clinical progression: A study of fully automated immunoassays in BioFINDER and ADNI cohorts. Alzheimer’s Dement. 2018 Nov;14(11):1470–81.
12. Vanderstichele HMJ, Janelidze S, Demeyer L, Coart E, Stoops E, Herbst V, et al. Optimized Standard Operating Procedures for the Analysis of Cerebrospinal Fluid Aβ42 and the Ratios of Aβ Isoforms Using Low Protein Binding Tubes. Galimberti D, editor. J Alzheimer’s Dis. 2016 Aug 3;53(3):1121–32.
13. Janelidze S, Zetterberg H, Mattsson N, Palmqvist S, Vanderstichele H, Lindberg O, et al. CSF A β 42/A β 40 and A β 42/A β 38 ratios: better diagnostic markers of Alzheimer disease. Ann Clin Transl Neurol. 2016 Mar;3(3):154–65.
14. Hansson O, Lehmann S, Otto M, Zetterberg H, Lewczuk P. Advantages and disadvantages of the use of the CSF Amyloid β (Aβ) 42/40 ratio in the diagnosis of Alzheimer’s Disease. Alzheimers Res Ther. 2019 Dec 22;11(1):34.
15. Thurfjell L, Lilja J, Lundqvist R, Buckley C, Smith A, Vandenberghe R, et al. Automated quantification of 18F-flutemetamol PET activity for categorizing scans as negative or positive for brain amyloid: Concordance with visual image reads. J Nucl Med. 2014 Oct 1;55(10):1623–8.
16. Cohen AD, Mowrey W, Weissfeld LA, Aizenstein HJ, McDade E, Mountz JM, et al. Classification of amyloid-positivity in controls: Comparison of visual read and quantitative approaches. Neuroimage. 2013 May 1;71:207–15.
17. Schreiber S, Landau SM, Fero A, Schreiber F, Jagust WJ. Comparison of visual and quantitative florbetapir F 18 positron emission tomography analysis in predicting mild cognitive impairment outcomes. JAMA Neurol. 2015 Oct 1;72(10):1183–90.
18. Klunk WE, Koeppe RA, Price JC, Benzinger TL, Devous MD, Jagust WJ, et al. The Centiloid project: Standardizing quantitative amyloid plaque estimation by PET. Alzheimer’s Dement. 2015 Jan;11(1):1-15.e4.
19. La Joie R, Ayakta N, Seeley WW, Borys E, Boxer AL, DeCarli C, et al. Multisite study of the relationships between antemortem [ 11 C]PIB-PET Centiloid values and postmortem measures of Alzheimer’s disease neuropathology. Alzheimer’s Dement. 2019 Feb 1;15(2):205–16.
20. Salvadó G, Molinuevo JL, Brugulat-Serrat A, Falcon C, Grau-Rivera O, Suárez-Calvet M, et al. Centiloid cut-off values for optimal agreement between PET and CSF core AD biomarkers. Alzheimers Res Ther. 2019 Dec 21;11(1):27.
21. Aisen PS, Cummings J, Doody R, Kramer L, Salloway S, Selkoe DJ, et al. The Future of Anti-Amyloid Trials. J Prev Alzheimer’s Dis. 2020 Mar 1;7(3):146–51.
22. Blennow K, Zetterberg H. Amyloid and Tau Biomarkers in CSF. J Prev Alzheimer’s Dis. 2015;2(1):46–50.
23. Molinuevo JL, Gramunt N, Gispert JD, Fauria K, Esteller M, Minguillon C, et al. The ALFA project: A research platform to identify early pathophysiological features of Alzheimer’s disease. Alzheimer’s Dement (New York, N Y). 2016 Jun;2(2):82–92.
24. Teunissen CE, Tumani H, Engelborghs S, Mollenhauer B. Biobanking of CSF: International standardization to optimize biomarker development. Clin Biochem. 2014 Mar;47(4–5):288–92.
25. Amadoru S, Doré V, McLean CA, Hinton F, Shepherd CE, Halliday GM, et al. Comparison of amyloid PET measured in Centiloid units with neuropathological findings in Alzheimer’s disease. Alzheimers Res Ther. 2020 Dec 4;12(1):22.
26. Leuzy A, Chiotis K, Hasselbalch SG, Rinne JO, de Mendonça A, Otto M, et al. Pittsburgh compound B imaging and cerebrospinal fluid amyloid-β in a multicentre European memory clinic study. Brain. 2016 Sep;139(9):2540–53.
27. Rowe CC, Doré V, Jones G, Baxendale D, Mulligan RS, Bullich S, et al. 18F-Florbetaben PET beta-amyloid binding expressed in Centiloids. Eur J Nucl Med Mol Imaging. 2017 Nov 1;44(12):2053–9.
28. Benjamini, Yoav ; Hochberg Y. Controlling the False Discovery Rate – a Practical and Powerful Approach to Multiple Testing. Journal of the Royal Statistical Society Series B-Methodological 1995.pdf. J R Stat Soc Ser B. 1995;57(1):289–300.
29. Bertens D, Tijms BM, Scheltens P, Teunissen CE, Visser PJ. Unbiased estimates of cerebrospinal fluid β-amyloid 1-42 cutoffs in a large memory clinic population. Alzheimer’s Res Ther. 2017 Feb 14;9(1):8.
30. Willemse EAJ, van Maurik IS, Tijms BM, Bouwman FH, Franke A, Hubeek I, et al. Diagnostic performance of Elecsys immunoassays for cerebrospinal fluid Alzheimer’s disease biomarkers in a nonacademic, multicenter memory clinic cohort: The ABIDE project. Alzheimer’s Dement Diagnosis, Assess Dis Monit. 2018 Jan 1;10:563–72.
31. De Meyer G, Shapiro F, Vanderstichele H, Vanmechelen E, Engelborghs S, De Deyn PP, et al. Diagnosis-independent Alzheimer disease biomarker signature in cognitively normal elderly people. Arch Neurol. 2010 Aug;67(8):949–56.
32. Palmqvist S, Mattsson N, Hansson O. Cerebrospinal fluid analysis detects cerebral amyloid-β accumulation earlier than positron emission tomography. Brain. 2016 Apr;139(4):1226–36.